Deep Speaker Recognition: Modular or Monolithic?
INTERSPEECH(2019)
摘要
Speaker recognition has made extraordinary progress with the advent of deep neural networks. In this work, we analyze the performance of end-to-end deep speaker recognizers on two popular text-independent tasks - NIST-SRE 2016 and VoxCeleb. Through a combination of a deep convolutional feature extractor, self-attentive pooling and large-margin loss functions, we achieve state-of-the-art performance on VoxCeleb. Our best individual and ensemble models show a relative improvement of 70% an 82% respectively over the best reported results on this task. On the challenging NIST-SRE 2016 task, our proposed end-to-end models show good performance but are unable to match a strong i-vector baseline. State-of-the-art systems for this task use a modular framework that combines neural network embeddings with a probabilistic linear discriminant analysis (PLDA) classifier. Drawing inspiration from this approach we propose to replace the PLDA classifier with a neural network. Our modular neural network approach is able to outperform the i-vector baseline using cosine distance to score verification trials.
更多查看译文
关键词
deep speaker recognition, end-to-end, large margin loss
AI 理解论文
溯源树
样例
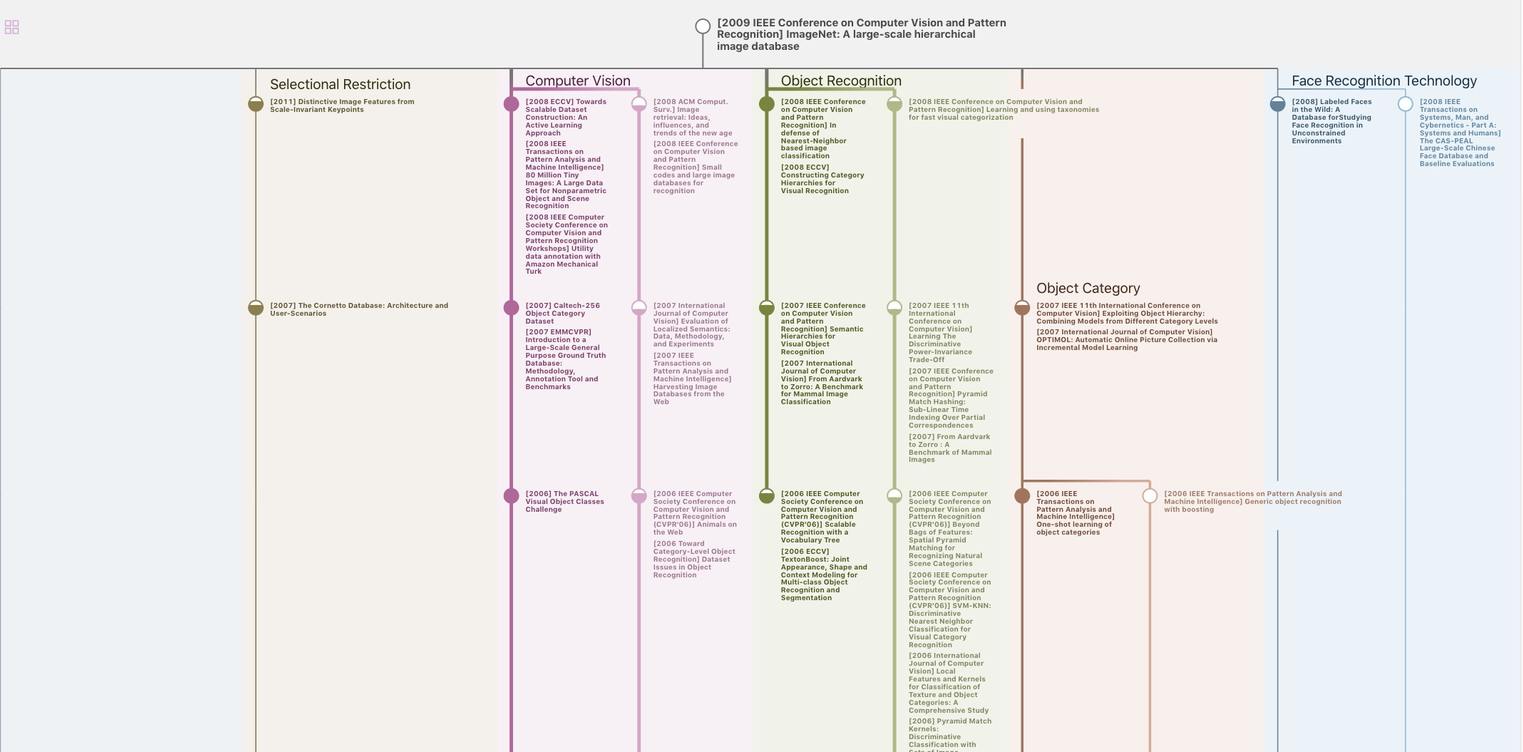
生成溯源树,研究论文发展脉络
Chat Paper
正在生成论文摘要