110th Anniversary: Surrogate Models Based on Artificial Neural Networks To Simulate and Optimize Pressure Swing Adsorption Cycles for CO2 Capture
INDUSTRIAL & ENGINEERING CHEMISTRY RESEARCH(2019)
摘要
Carbon capture technologies are expected to play a key role in the global energy system, as it is likely that fossil fuels will continue to be dominant in the world's energy mix in the near future. Pressure swing adsorption (PSA) is a promising alternative among currently available technologies for carbon capture due to its low energy requirements. Still, the design of the appropriate PSA cycle for a given adsorbent material is a challenge that must be addressed to make PSA commercially competitive for carbon capture applications. In this work, we propose and test a model reduction-based approach that systematically generates low-order representations of rigorous PSA models. These reduced-order models are obtained by training artificial neural networks on data collected from full partial differential algebraic equation (PDAE) model simulations. The main contribution of this paper is the development of surrogate models for every possible step in PSA cycles: pressurization, adsorption, and depressurization steps in cocurrent and counter-current operation. Three different PSA cycles (three-step, Skarstrom, and five-step cycle) for postcombustion carbon capture applications were employed for training purposes, and two adsorbents, Ni-MOF-74 and zeolite 13X, were chosen to evaluate the surrogate models under optimized cycle conditions. A good agreement was observed between the results of the ANN models and the PDAE simulations. Average mean square errors, for dimensionless state variables, of 1.7 x 10(-8), 5.8 x 10(-8), and 9.9 x 10(-7) were obtained for the three PSA cycles analyzed in this work, and the highest relative error, regarding the CO2 purity and recovery, was 1.42%. These results suggest that the use of machine learning techniques to develop PSA surrogate models is feasible and that these models can be implemented in optimization environments to synthesize PSA cycles.
更多查看译文
AI 理解论文
溯源树
样例
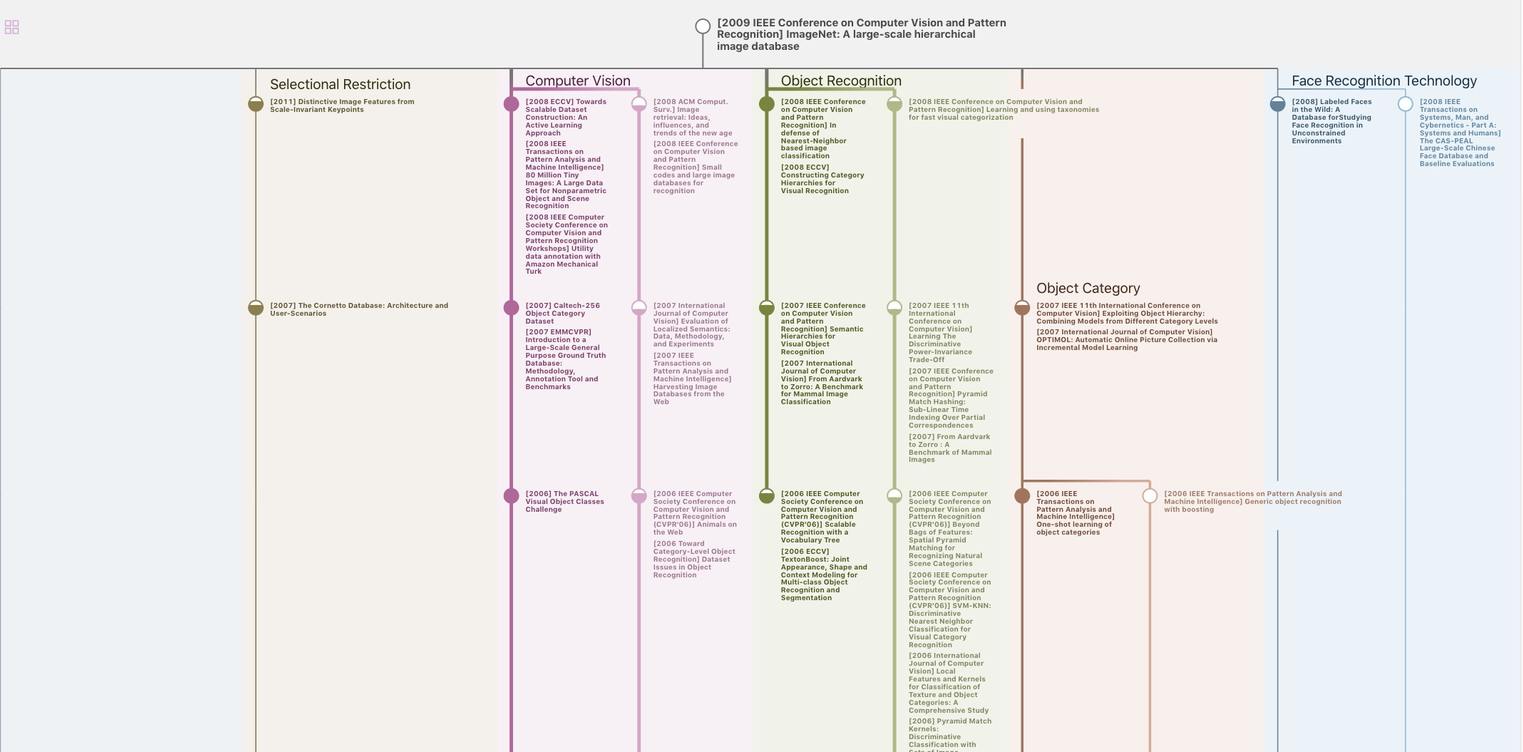
生成溯源树,研究论文发展脉络
Chat Paper
正在生成论文摘要