A new spatio-temporal background–foreground bimodal for motion segmentation and detection in urban traffic scenes
Neural Computing and Applications(2019)
摘要
Automatic vehicle detection in urban traffic surveillance is an important and urgent issue, since it provides necessary information for further processing. Conventional techniques utilize either motion segmentation or appearance-based detection, which involves either poor adaptation or high computation. The complexity of urban traffic scenarios lies in slow motion temporarily stopped or parked vehicles, dynamic background, and sudden illumination variations. In this paper, a new motion segmentation technique is proposed based on spatio-temporal background–foreground bimodal. The temporal background information is modeled using a weighted sigma–delta estimation, cumulative frame differencing is used to model the foreground pixels, and the spatial correlation between neighboring pixels is utilized to combine both background and foreground models. The median of consecutive frame difference adapts sudden illumination variation, update background model, and reinitialize foreground model. Comparative experimental results for typical urban traffic sequences show that the proposed technique achieves robust and accurate segmentation, which improves adaptation, reduce false detection, and satisfy real-time requirements.
更多查看译文
关键词
Motion segmentation, Background subtraction, Cumulative frame differencing, Sigma–delta filter, Vehicle detection
AI 理解论文
溯源树
样例
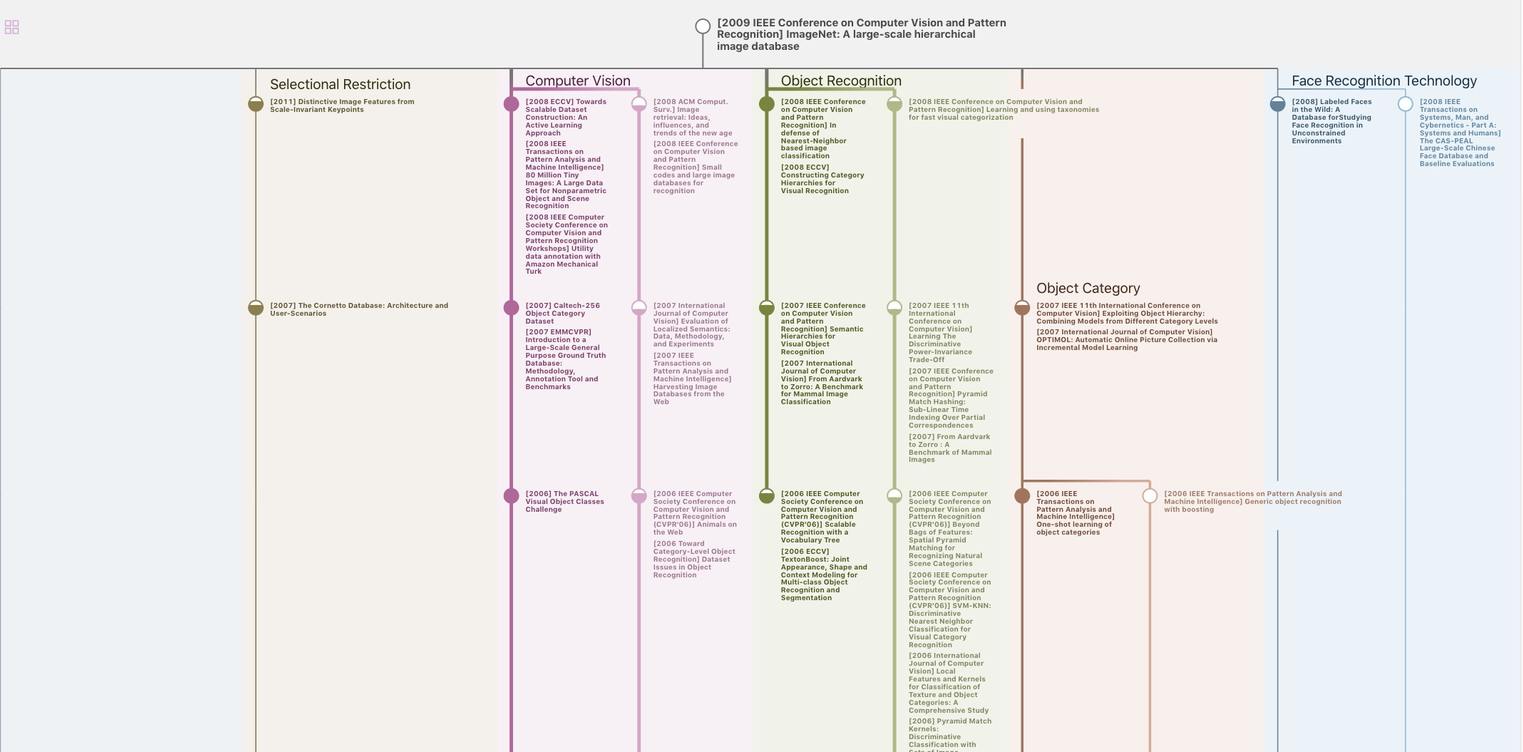
生成溯源树,研究论文发展脉络
Chat Paper
正在生成论文摘要