Simulation-driven machine learning for robotics and automation
TM-TECHNISCHES MESSEN(2019)
摘要
Mass personalization-a megatrend in industrial manufacturing and production-requires fast adaptations of robotics and automation solutions to continually decreasing lot sizes. In this paper, the challenges of applying robot-based automation in a highly individualized production are highlighted. To face these challenges, a framework is proposed that combines latest machine learning (ML) techniques, like deep learning, with high-end physics simulation environments. ML is used for programming and parameterizing machines for a given production task with minimal human intervention. If the simulation environment realistically captures physical properties like forces or elasticity of the real world, it provides a high-quality data source for ML. In doing so, new tasks are mastered in simulation faster than in real-time, while at the same time existing tasks are executed. The functionality of the simulation-driven ML framework is demonstrated on an industrial use case.
更多查看译文
关键词
Mass personalization,machine learning,simulation,robotics,automation,reinforcement learning,artificial neural networks
AI 理解论文
溯源树
样例
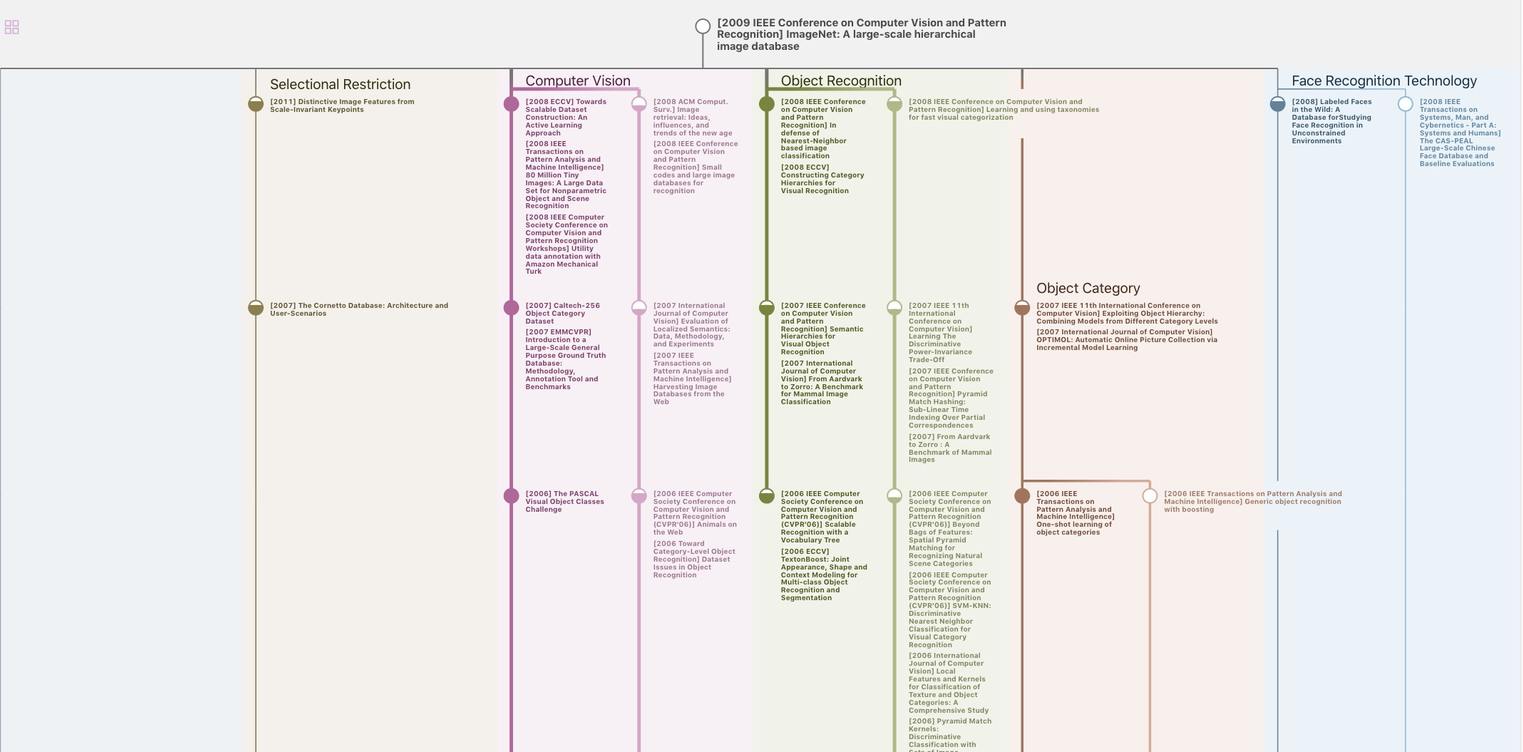
生成溯源树,研究论文发展脉络
Chat Paper
正在生成论文摘要