Visualizing the Stability of 2D Point Sets from Dimensionality Reduction Techniques
COMPUTER GRAPHICS FORUM(2020)
摘要
We use k-order Voronoi diagrams to assess the stability of k-neighbourhoods in ensembles of 2D point sets, and apply it to analyse the robustness of a dimensionality reduction technique to variations in its input configurations. To measure the stability of k-neighbourhoods over the ensemble, we use cells in the k-order Voronoi diagrams, and consider the smallest coverings of corresponding points in all point sets to identify coherent point subsets with similar neighbourhood relations. We further introduce a pairwise similarity measure for point sets, which is used to select a subset of representative ensemble members via the PageRank algorithm as an indicator of an individual member's value. The stability information is embedded into the k-order Voronoi diagrams of the representative ensemble members to emphasize coherent point subsets and simultaneously indicate how stable they lie together in all point sets. We use the proposed technique for visualizing the robustness of t-distributed stochastic neighbour embedding and multi-dimensional scaling applied to high-dimensional data in neural network layers and multi-parameter cloud simulations.
更多查看译文
关键词
visualization,center dot Human-centred computing -> Visualization techniques,center dot Computing methodologies -> Dimensionality reduction and manifold learning
AI 理解论文
溯源树
样例
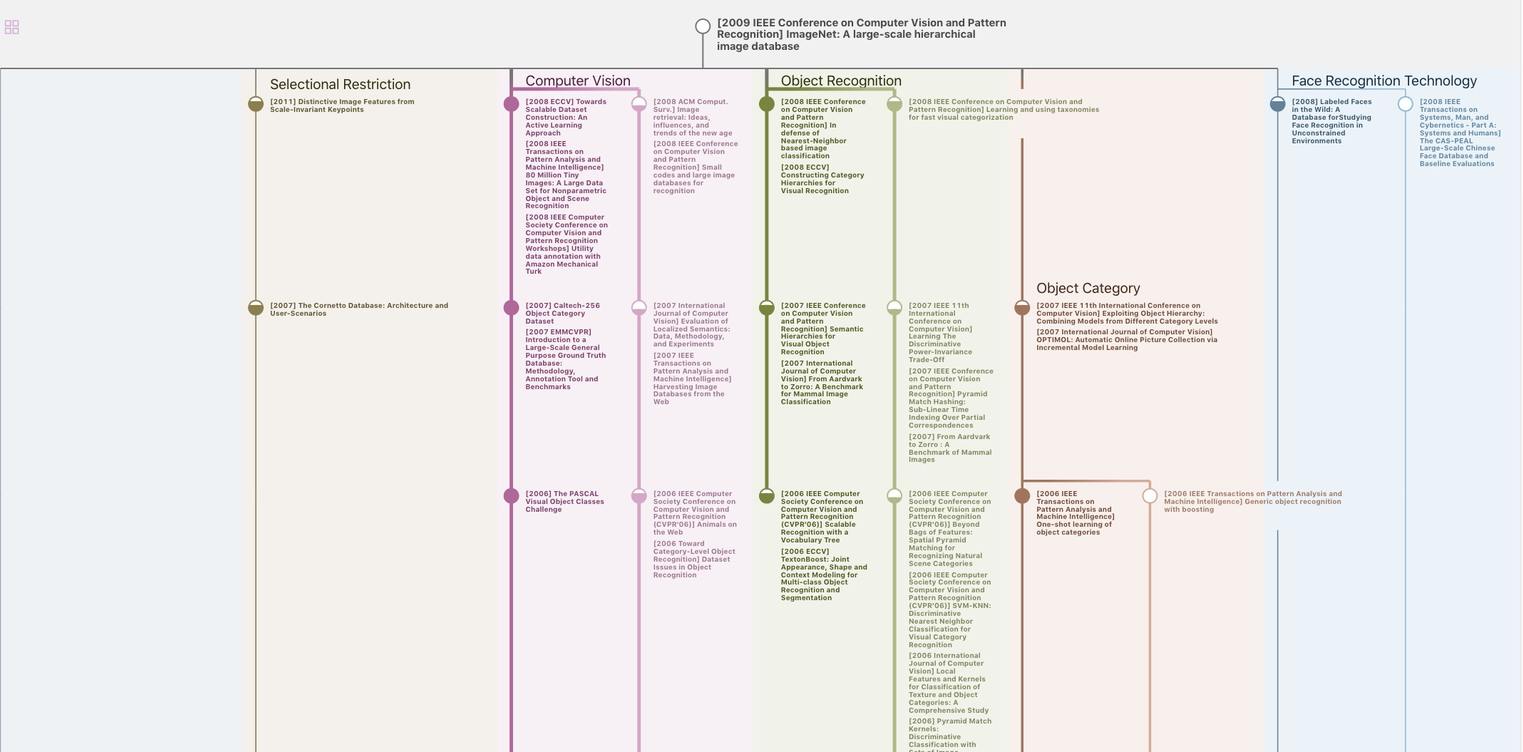
生成溯源树,研究论文发展脉络
Chat Paper
正在生成论文摘要