Prediction Of Multiple 3d Tissue Structures Based On Single-Marker Images Using Convolutional Neural Networks
2019 IEEE INTERNATIONAL CONFERENCE ON IMAGE PROCESSING (ICIP)(2019)
摘要
A quantitative understanding of complex biological systems such as tissues requires reconstructing the structure of the different components of the system. Fluorescence microscopy provides the means to visualize simultaneously several tissue components. However, it can be time consuming and is limited by the number of fluorescent markers that can be used. In this study, we describe a toolbox of algorithms based on convolutional neural networks for the prediction of 3D tissue structures by learning features embedded within single-marker images. As proof of principle, we aimed to predict the network of bile canaliculi (BC) in liver tissue using images of the cortical actin mesh as input. The actin meshwork has a characteristic organization in specific cellular domains, such as BC. However, the use of manually selected features from images of actin is not sufficient to properly reconstruct BC structure. Our deep learning framework showed a remarkable accuracy for the prediction of BC network and was successfully adapted (i.e. transfer learning) to predict the sinusoidal network. This approach allows for a complete reconstruction of tissue microarchitecture using a single fluorescent marker.
更多查看译文
关键词
Deep Learning, convolutional neural networks, fluorescence microscopy, biological tissue, liver
AI 理解论文
溯源树
样例
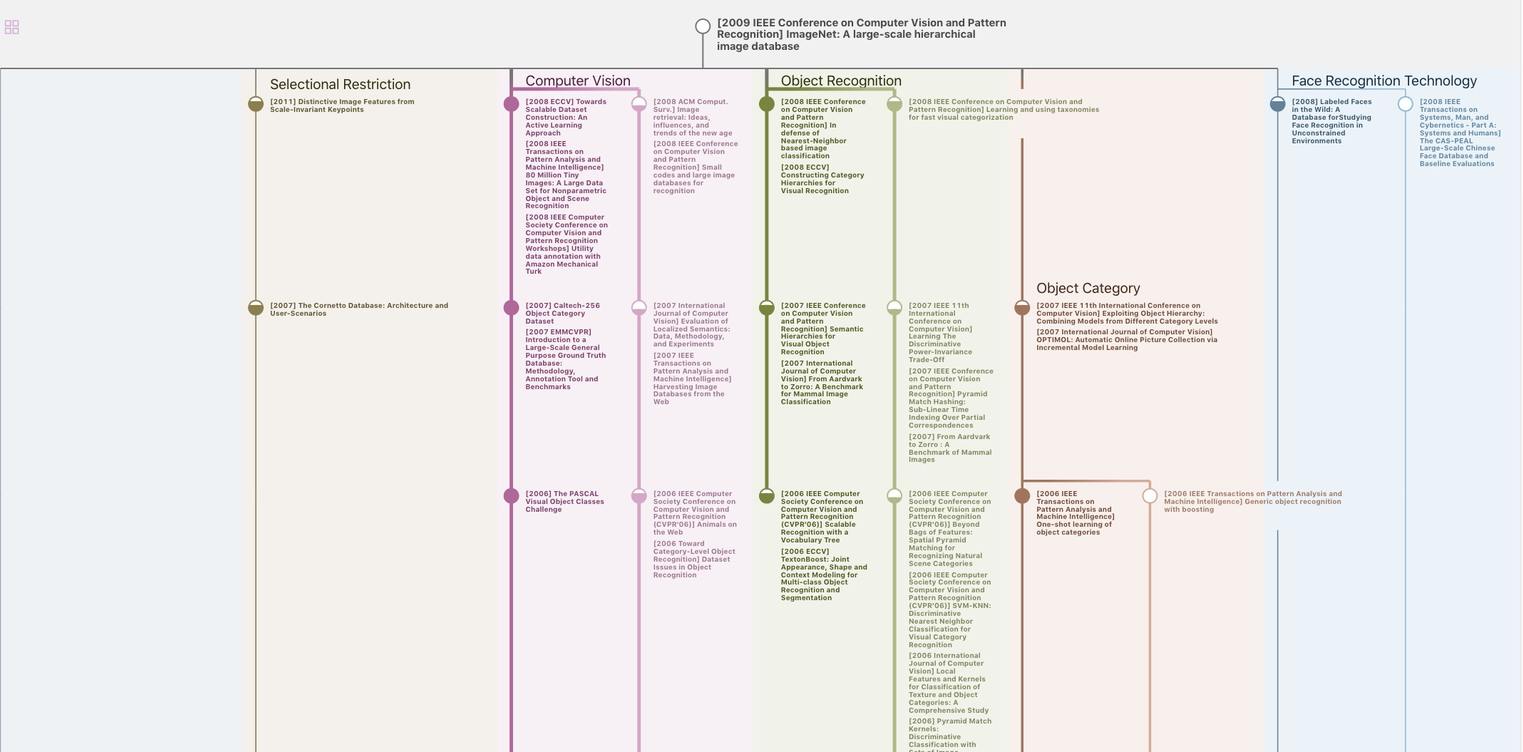
生成溯源树,研究论文发展脉络
Chat Paper
正在生成论文摘要