Differential Privacy Meets Verifiable Computation: Achieving Strong Privacy And Integrity Guarantees
PROCEEDINGS OF THE 16TH INTERNATIONAL JOINT CONFERENCE ON E-BUSINESS AND TELECOMMUNICATIONS, VOL 2: SECRYPT(2019)
摘要
Often service providers need to outsource computations on sensitive datasets and subsequently publish statistical results over a population of users. In this setting, service providers want guarantees about the correctness of the computations, while individuals want guarantees that their sensitive information will remain private. Encryption mechanisms are not sufficient to avoid any leakage of information, since querying a database about individuals or requesting summary statistics can lead to leakage of information. Differential privacy addresses the paradox of learning nothing about an individual, while learning useful information about a population. Verifiable computation addresses the challenge of proving the correctness of computations. Although verifiable computation and differential privacy are important tools in this context, their interconnection has received limited attention. In this paper, we address the following question: How can we design a protocol that provides both differential privacy and verifiable computation guarantees for outsourced computations? We formally define the notion of verifiable differentially private computation (VDPC) and what are the minimal requirements needed to achieve VDPC. Furthermore, we propose a protocol that provides verifiable differentially private computation guarantees and discuss its security and privacy properties.
更多查看译文
关键词
Verifiable Computation, Differential Privacy, Privacy-preservation
AI 理解论文
溯源树
样例
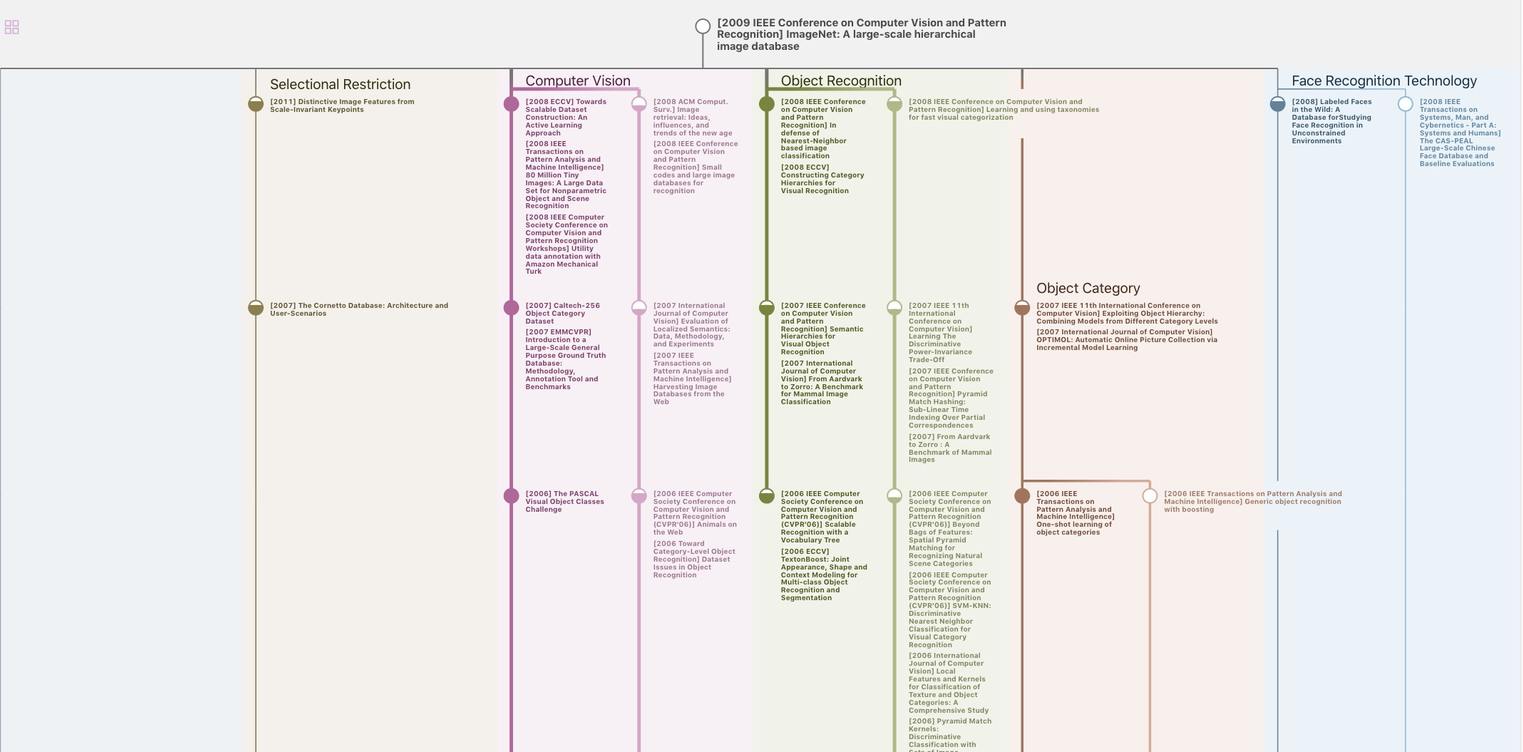
生成溯源树,研究论文发展脉络
Chat Paper
正在生成论文摘要