A vision and learning-based indoor localization and semantic mapping framework for facility operations and management
Automation in Construction(2019)
摘要
Recent research on facility management has focused on leveraging location-based services (LBS) to assist on-demand access to model information and on-site documentation. Fast and robust indoor localization is of great importance for location-based facility management services, such as the ones used in mobile computing settings. However, there are several challenges in achieving fast and robust indoor localization: 1) Signal-based indoor localization methods, such as WIFI, RFID, Bluetooth and Ultrasound, require installation of extra infrastructures in a building to support localization; 2) Visual-based indoor localization methods, such as LiDAR and camera, depend on feature point detection and matching, which require heavy computation and can also be impacted by environmental conditions, such as lighting and texture richness. In addition to these, existing localization methods do not support semantic understanding which is of great importance when associating a component with its digital twin. To address the stated problems above, this paper presents a vision and learning-based framework that utilizes a shared convolutional neural network to perform localization and semantic segmentation simultaneously. The proposed framework can support facility management by locating facility components within a building and associate them with their digital twins in an information repository. Compared to conventional methods, the developed image-based indoor localization and semantic mapping framework has the following advantages: 1) It only requires image as input to support localization, semantic understanding, and association, which eliminates the need for extra infrastructure, such as deployment of RFID tags, etc.; 2) It reuses the feature extraction network for simultaneous localization and semantic understanding, which saves computing resources; 3) With 6-DoF poses and semantic labels, it supports component-level association. The authors evaluated the proposed framework on publicly available data sets using three metrics: localization accuracy, semantic segmentation accuracy, and association success rate. The results show that the proposed image-based method can achieve 6-DoF localization and semantic segmentation concurrently. Also, formalized experiments on a synthetic data set with different noise levels introduced to localization and semantic segmentation showed that a main factor affecting the performance of association of an image to its digital twin is the accuracy of its localization.
更多查看译文
关键词
Facility operation and management,Image,Indoor localization,Semantic understanding,Multi-task deep learning,Convolutional neural network
AI 理解论文
溯源树
样例
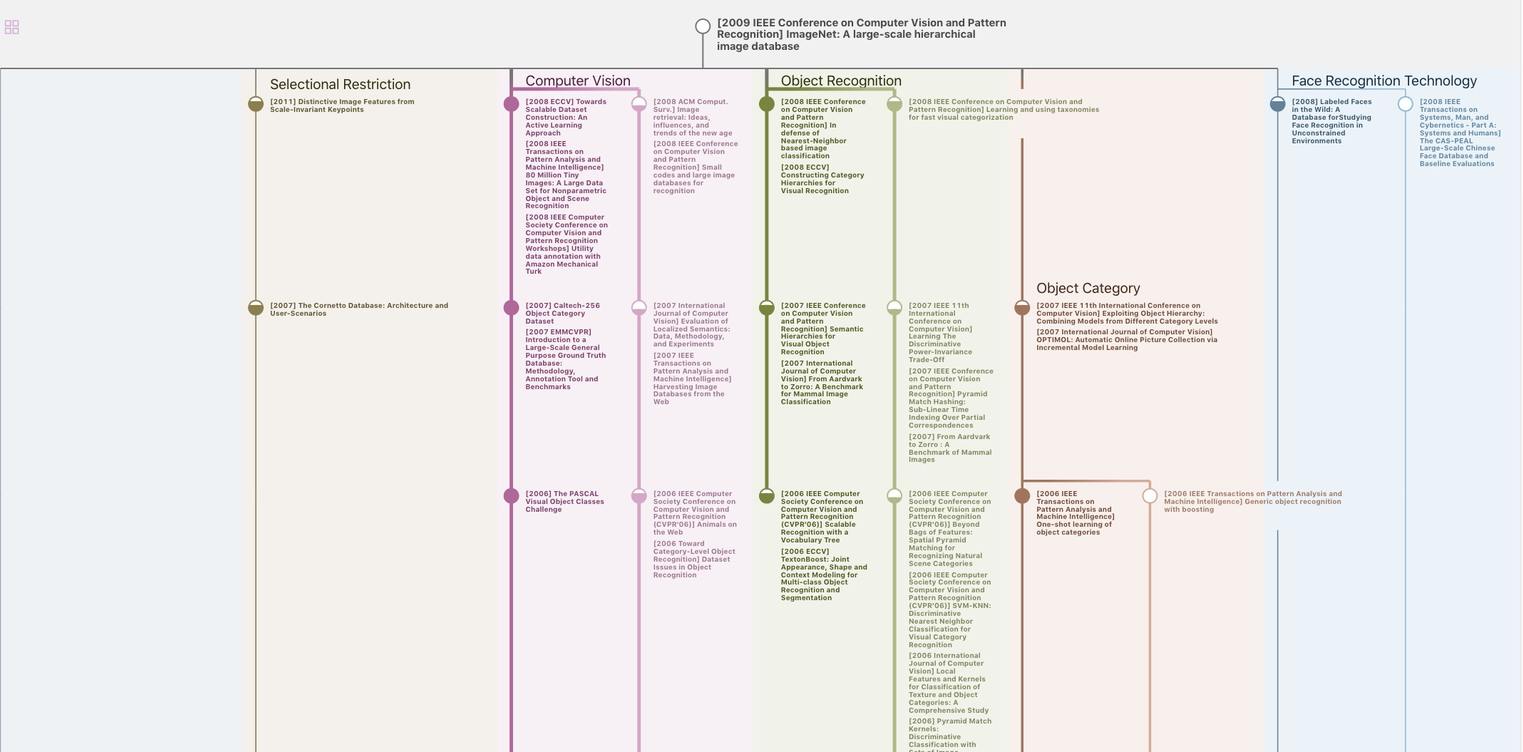
生成溯源树,研究论文发展脉络
Chat Paper
正在生成论文摘要