Support vector machine regression on selected wavelength regions for quantitative analysis of caffeine in tea leaves by near infrared spectroscopy
JOURNAL OF CHEMOMETRICS(2019)
摘要
Caffeine is an important component that determines the quality of tea, and its rapid estimation is very much needed for the industry. In this pursuit, a near-infrared (NIR) spectroscopy-based technique for the estimation of caffeine is developed and presented in this paper. On the basis of responses of the different bonds present in caffeine, four specific wavelength windows-(a) 1075 to 1239.5 nm (C?H stretch second overtone); (b) 1339.25 to 1440.75 nm (C?H stretch and C?H deformation); (c) 1640.25 to 1700 nm (C?H stretch first overtone, CH & amp; ?CH3 asymmetric); and (d) 900 to 1700 nm (whole range of the spectrometer)-were analyzed in details for model development and to obtain the effective wavelength (EW). Five different preprocessing techniques followed by two regression techniques-(a) the partial least-squares (PLS) and (b) the support vector regression (SVR) were implemented on raw data for analysis. Comparing all the models, the wavelength band of 1075 to 1239.5 nm and 1339.25 to 1440.75 nm were found to produce satisfactory results. The best discrimination result was obtained using the combination of standard normal variate (SNV) preprocessing with SVR at the 1075 to 1239.5 nm wavelength region. The SVR regression with 105 samples in the training set and 15 samples in the testing set resulted in the performance parameters as RMSECV = 0.134, RMSEP = 0.069, r(cv)(2) = 0.869, r(p)(2) = 0.65, and RPD = 5.626 at 1075 to 1239.5 nm, whereas the PLS model produced the best RMSECV = 0.287, RMSEP = 0.077, r(cv)(2) = 0.637, r(p)(2) = 0.675, and RPD = 5.218 at 1339.25 to 1440.75-nm wavelength band.
更多查看译文
关键词
caffeine,near infrared reflectance (NIR) spectroscopy,partial least square regression (PLSR),preprocessing,selected waveband,support vector regression (SVR)
AI 理解论文
溯源树
样例
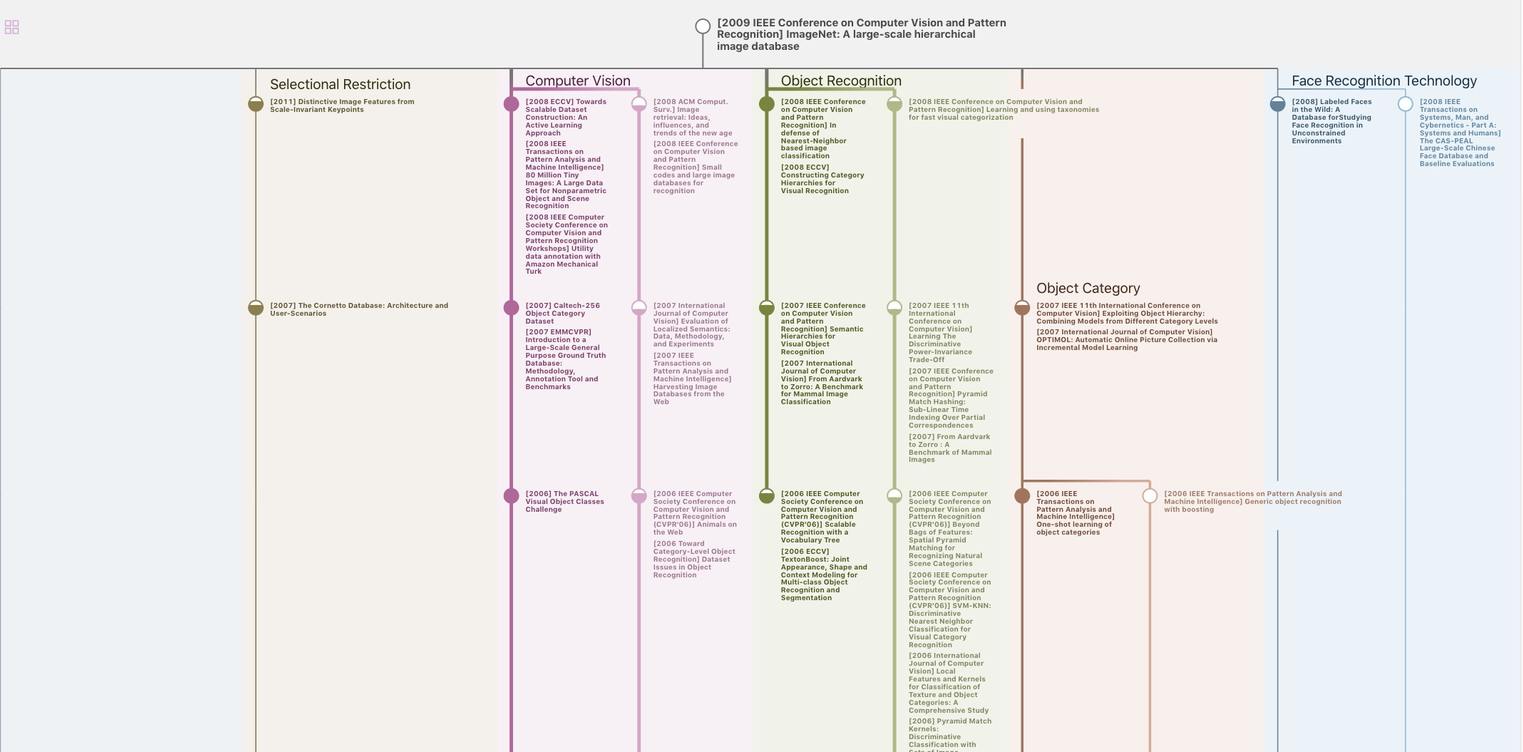
生成溯源树,研究论文发展脉络
Chat Paper
正在生成论文摘要