Joint Time-Frequency RSSI Features for Convolutional Neural Network-Based Indoor Fingerprinting Localization
IEEE ACCESS(2019)
摘要
The performance of localization methods based on the receiver signal strength (RSS) is significantly affected by the signal strength indicator's (RSSI) instability. To date, there is no adequate approach which significantly reduces the impact of such an instability on the localization accuracy. Hence, in this paper, we propose a continuous wavelet transform (CWT)-based feature extraction method for convolutional neural network (CNN)-based indoor fingerprinting localization method. The proposed feature extraction method uses the continuous wavelet transform to extract the joint time-frequency representation of each raw RSSI data which provides more discriminative information. The extracted features are used with a CNN model to efficiently predict the closest reference points (RPs). Then, a K-nearest neighbors (KNN) model is used to compute the target location. The proposed feature extraction method can be used with a generic deep neural network model to increase the performance where the computing node is not powerful. The proposed method has been evaluated on different datasets and has achieved good performance compared with other well-known existing methods. The experimental results also demonstrated that the proposed approach reduces the influence of RSSI variation.
更多查看译文
关键词
Convolutional neural network,continuous wavelet transform,RSSI-based fingerprinting localization
AI 理解论文
溯源树
样例
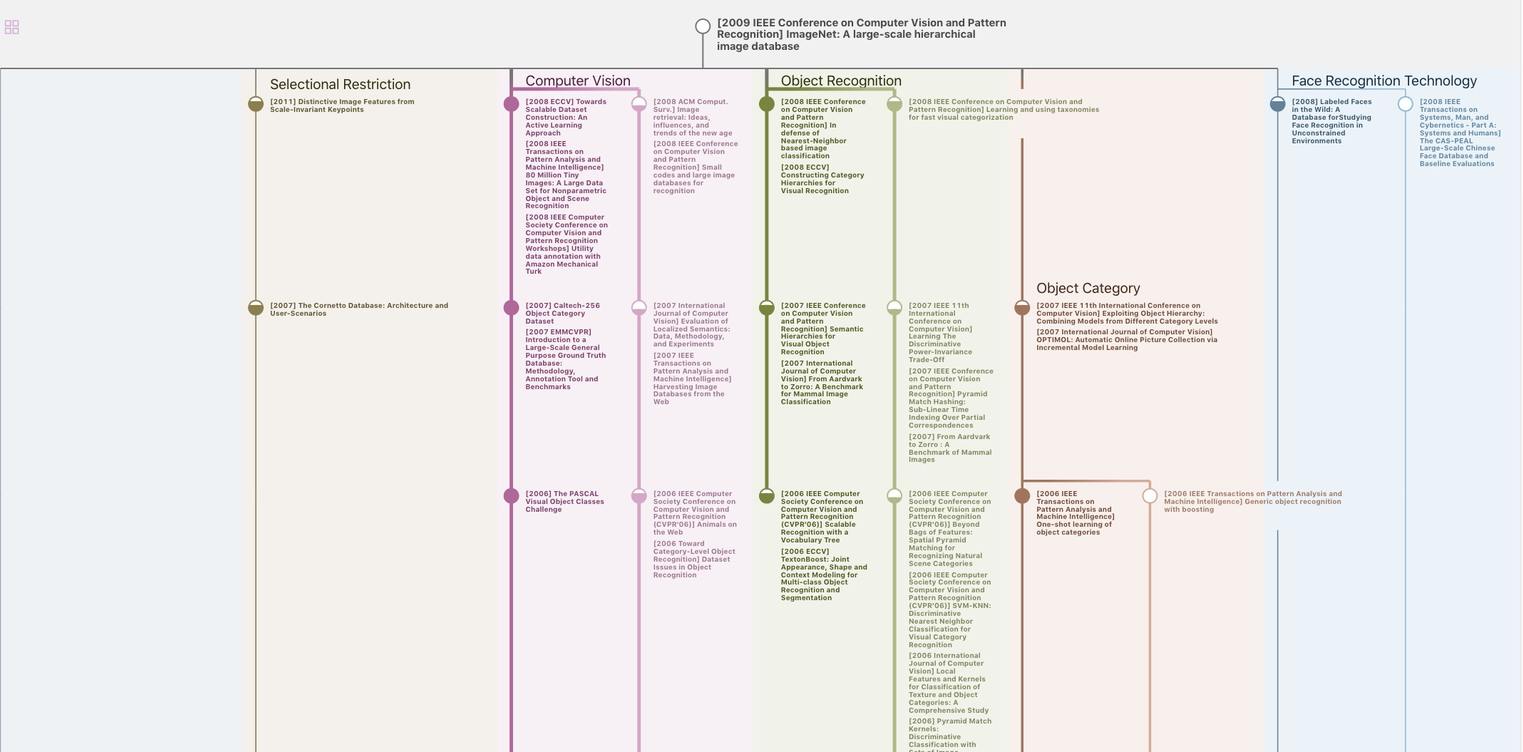
生成溯源树,研究论文发展脉络
Chat Paper
正在生成论文摘要