Long-Term Real-Time Correlation Filter Tracker For Mobile Robot
INTELLIGENT ROBOTICS AND APPLICATIONS, ICIRA 2019, PT I(2019)
摘要
Computer vision has received a significant attention in recent years, which is one of the important parts for robots to apperceive external environment. Discriminative Correlation Filter (DCF) based trackers gained more popularity due to their efficiency, however, most of the-state-of-the-art trackers are effective for short-term tracking, not yet successfully addressed in long-term scene. In this work, we tackle the problems by introducing Long-term Real-time Correlation Filter (LRCF) tracker. First, fused features only including HOG and Color Names are employed to boost the tracking efficiency. Second, we used the standard principal component analysis (PCA) to reduction scheme in the translation and scale estimation phase for accelerating. Third, we learned a long-term correlation filter to keep the long-term memory ability. Finally, we update the filter with interval updates. The extensive experiments on popular Object Tracking Benchmark OTB-2013 datasets have demonstrated that the proposed tracker outperforms the state-of-the-art trackers significantly achieves a high real-time (33FPS) performance in our mobile robot hardware. The experimental results show that the novel tracker performance is better than the-state-of-the-art trackers.
更多查看译文
关键词
Object tracking, Correlation filter, Long-term tracking, Robot
AI 理解论文
溯源树
样例
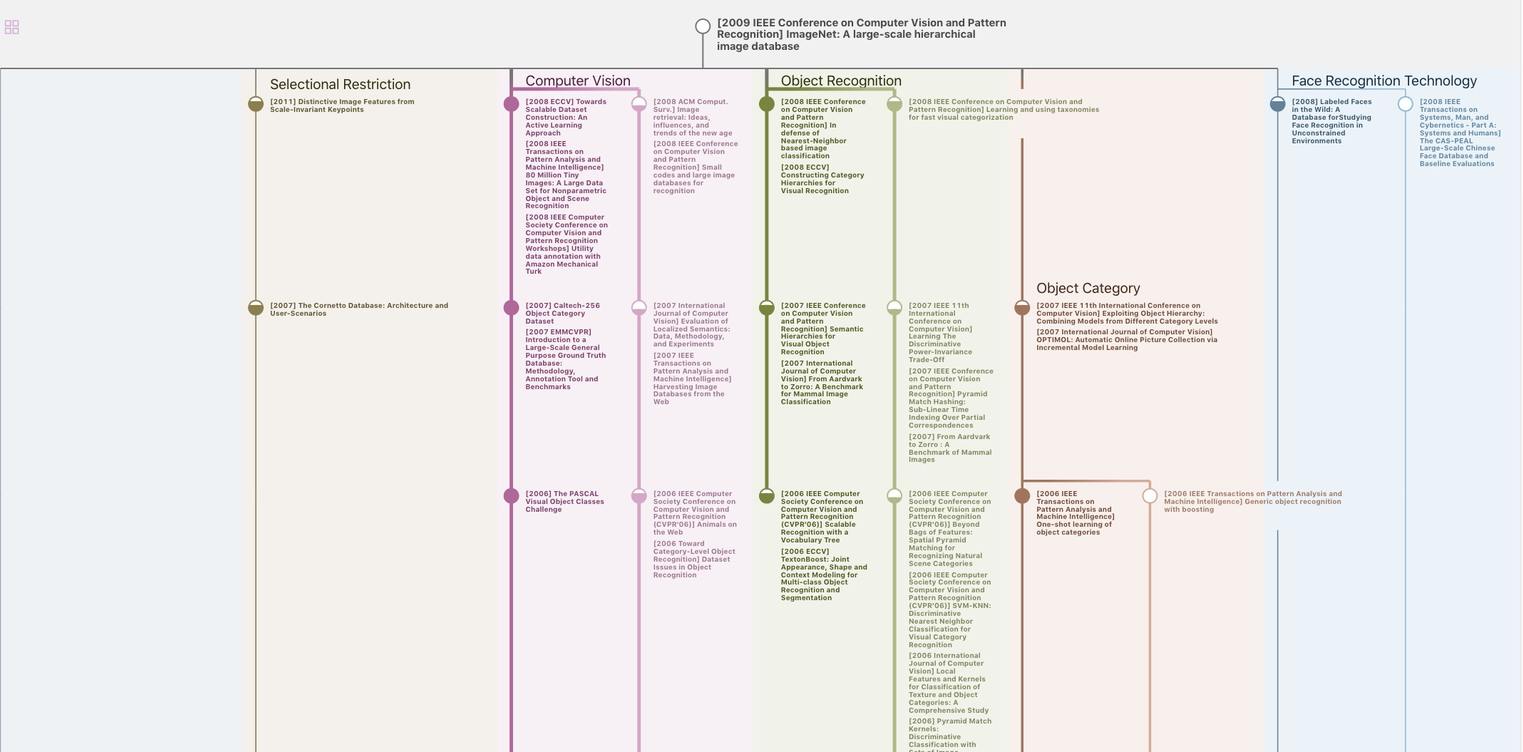
生成溯源树,研究论文发展脉络
Chat Paper
正在生成论文摘要