Learning Decentralized Control Policies For Multi-Robot Formation
2019 IEEE/ASME INTERNATIONAL CONFERENCE ON ADVANCED INTELLIGENT MECHATRONICS (AIM)(2019)
摘要
Decentralized formation control has been extensively studied using model-based methods, which rely on model accuracy and communication reliability. Motivated by recent advances in deep learning techniques whereby an intelligent agent is trained to compute its actions directly from high-dimensional raw sensory inputs using end-to-end decision-making policies, we consider the problem of learning decentralized control policies for multi-robot formation. A deep neural network is designed to model the control policy that maps the robot's local observations to control commands. We propose to use a centralized training framework based on supervised learning for control policy learning. The learned policy is then deployed on each robot in a decentralized manner for online formation control. Our proposed approach is verified and evaluated in experiments using a robotic simulator. Simulation results demonstrate satisfactory performance of formation control. Compared with existing methods for formation control, the proposed approach does not need inter-robot communication, and avoids hand-engineering the components of perception and control separately.
更多查看译文
关键词
decentralized control policies,decentralized formation control,model-based methods,communication reliability,deep learning techniques,deep neural network,supervised learning,control policy learning,online formation control,robotic simulator,inter-robot communication,end-to-end decision making policies,high dimensional raw sensory inputs,multi-robot formation
AI 理解论文
溯源树
样例
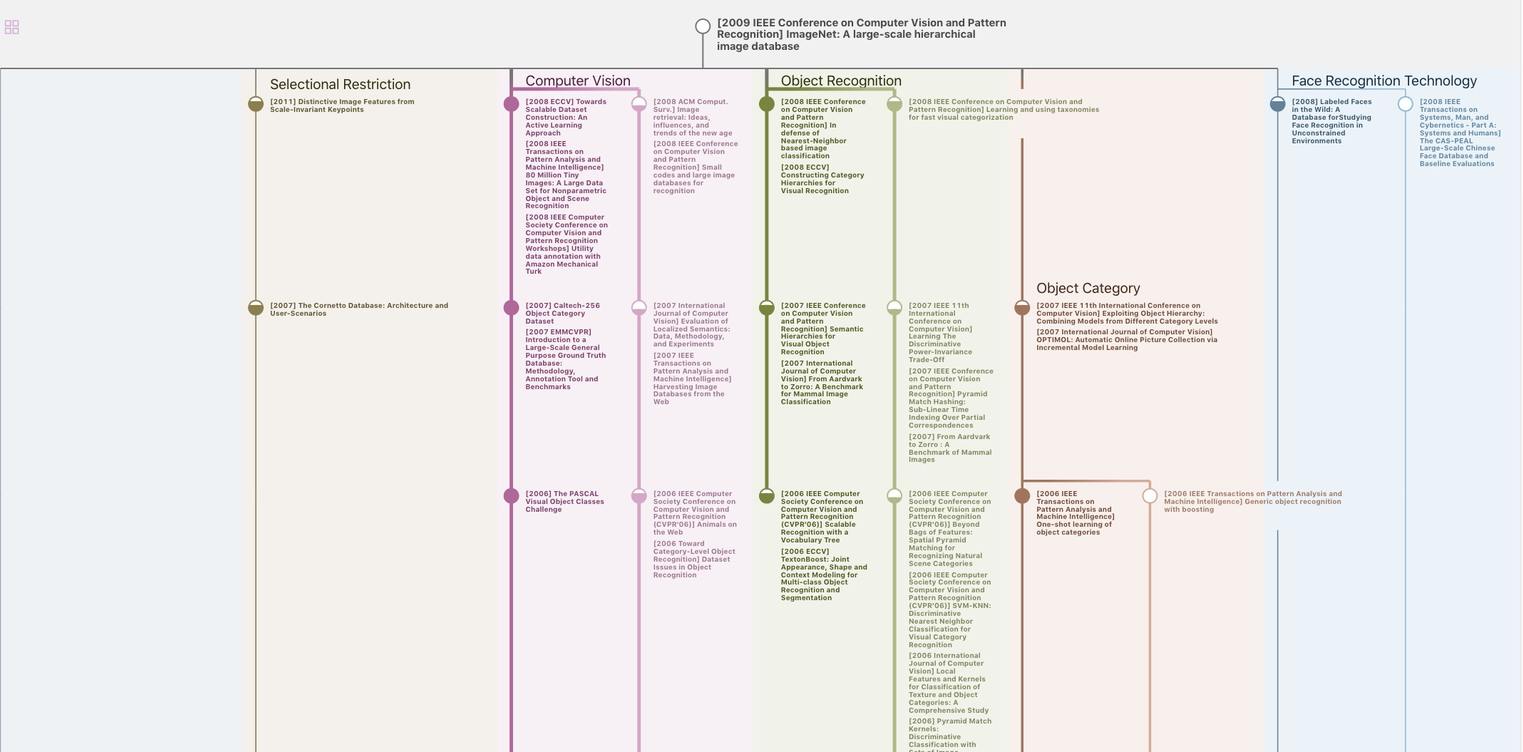
生成溯源树,研究论文发展脉络
Chat Paper
正在生成论文摘要