Micro-level Modularity of Computaion-intensive Programs in Big Data Platforms: A Case Study with Image Data
arxiv(2019)
摘要
With the rapid advancement of Big Data platforms such as Hadoop, Spark, and Dataflow, many tools are being developed that are intended to provide end users with an interactive environment for large-scale data analysis (e.g., IQmulus). However, there are challenges using these platforms. For example, developers find it difficult to use these platforms when developing interactive and reusable data analytic tools. One approach to better support interactivity and reusability is the use of microlevel modularisation for computation-intensive tasks, which splits data operations into independent, composable modules. However, modularizing data and computation-intensive tasks into independent components differs from traditional programming, e.g., when accessing large scale data, controlling data-flow among components, and structuring computation logic. In this paper, we present a case study on modularizing real world computationintensive tasks that investigates the impact of modularization on processing large scale image data. To that end, we synthesize image data-processing patterns and propose a unified modular model for the effective implementation of computation-intensive tasks on data-parallel frameworks considering reproducibility, reusability, and customization. We present various insights of using the modularity model based on our experimental results from running image processing tasks on Spark and Hadoop clusters.
更多查看译文
关键词
big data,platforms,programs,micro-level,computaion-intensive
AI 理解论文
溯源树
样例
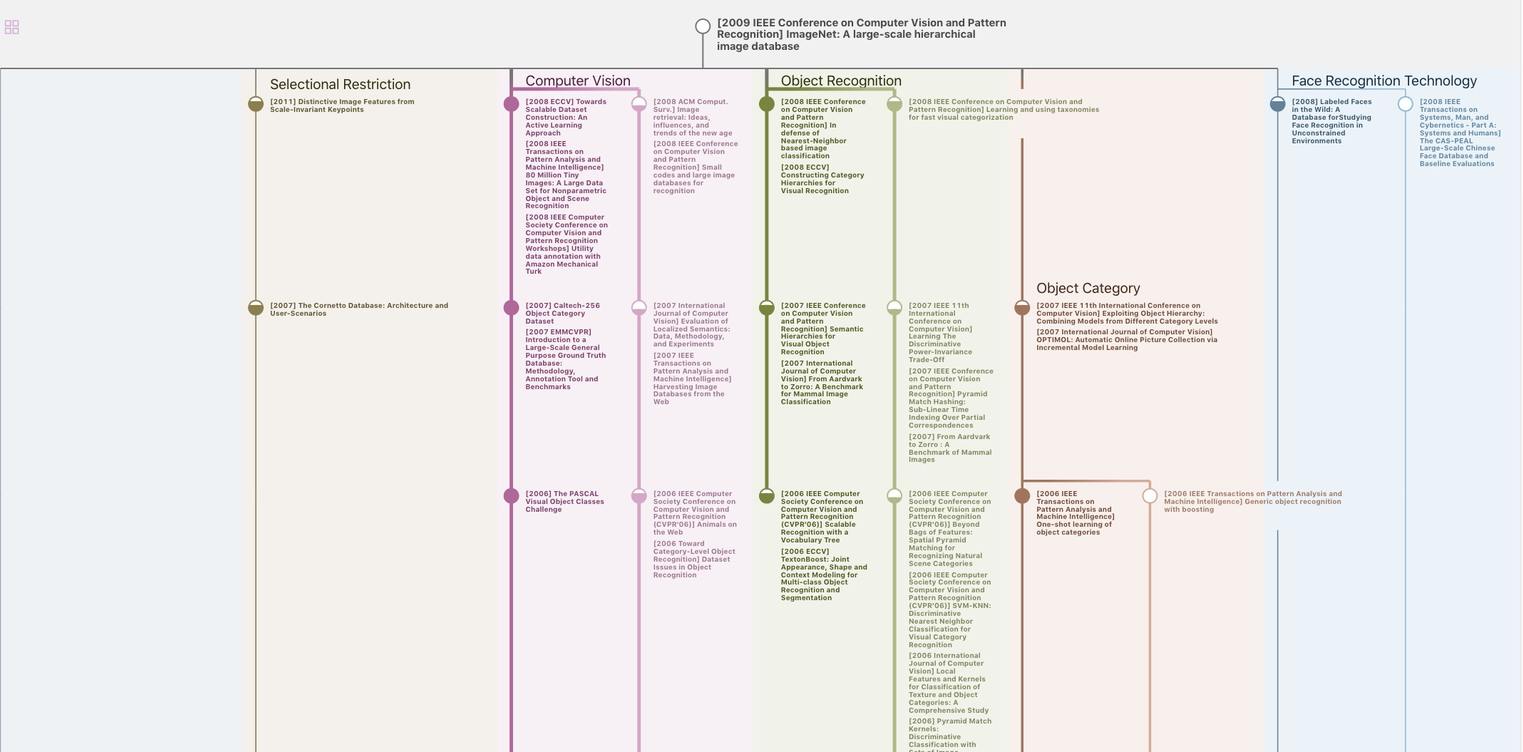
生成溯源树,研究论文发展脉络
Chat Paper
正在生成论文摘要