Embedding to reference t-SNE space addresses batch effects in single-cell classification
DS(2021)
摘要
Dimensionality reduction techniques, such as t-SNE, can construct informative visualizations of high-dimensional data. When jointly visualising multiple data sets, a straightforward application of these methods often fails; instead of revealing underlying classes, the resulting visualizations expose dataset-specific clusters. To circumvent these batch effects, we propose an embedding procedure that uses a t-SNE visualization constructed on a reference data set as a scaffold for embedding new data points. Each data instance from a new, unseen, secondary data is embedded independently and does not change the reference embedding. This prevents any interactions between instances in the secondary data and implicitly mitigates batch effects. We demonstrate the utility of this approach by analyzing six recently published single-cell gene expression data sets with up to tens of thousands of cells and thousands of genes. The batch effects in our studies are particularly strong as the data comes from different institutions using different experimental protocols. The visualizations constructed by our proposed approach are clear of batch effects, and the cells from secondary data sets correctly co-cluster with cells of the same type from the primary data. We also show the predictive power of our simple, visual classification approach in t-SNE space matches the accuracy of specialized machine learning techniques that consider the entire compendium of features that profile single cells.
更多查看译文
关键词
Batch effects,Embedding,t-SNE,Visualization,Single-cell transcriptomics,Data integration,Domain adaptation.
AI 理解论文
溯源树
样例
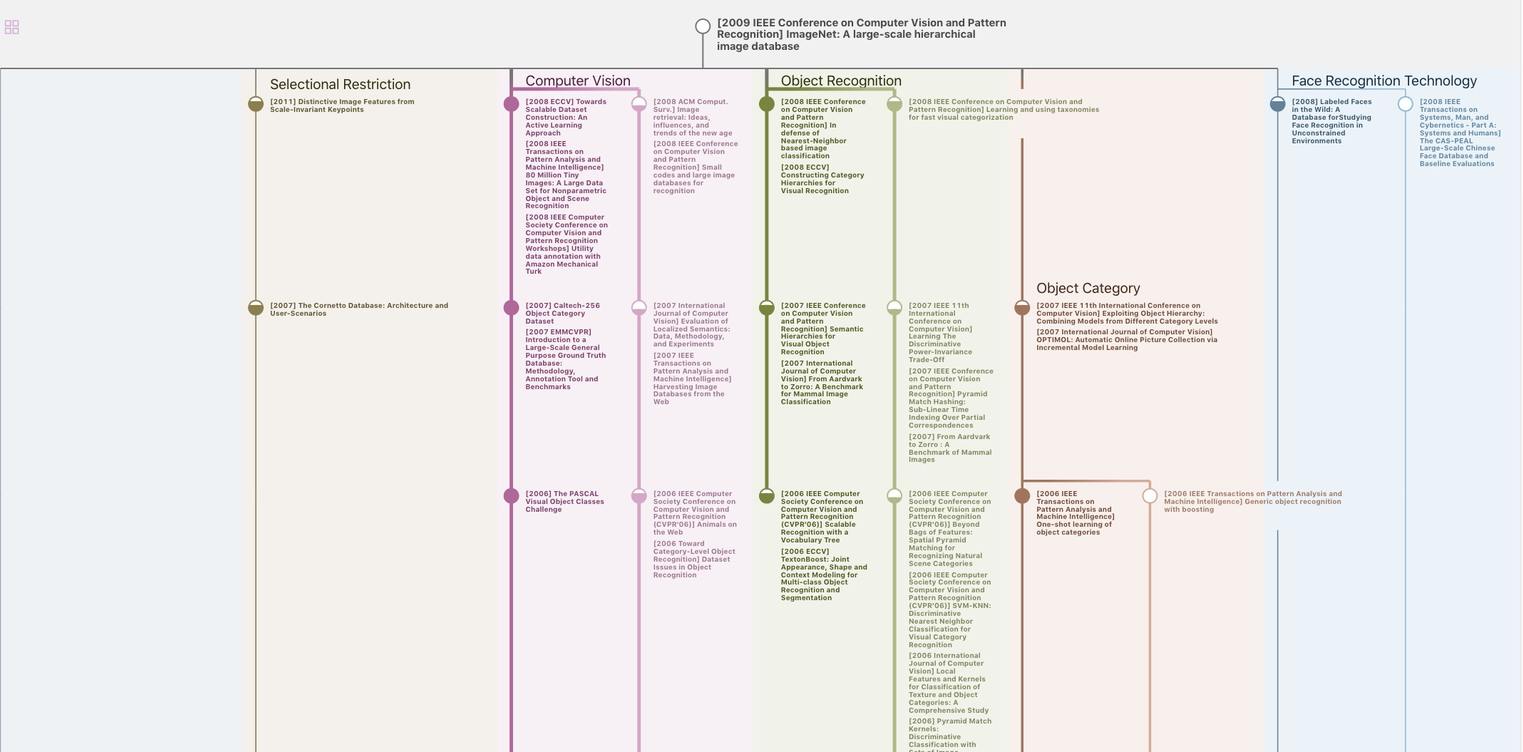
生成溯源树,研究论文发展脉络
Chat Paper
正在生成论文摘要