Pedestrian Attribute Recognition via Hierarchical Multi-task Learning and Relationship Attention
Proceedings of the 27th ACM International Conference on Multimedia(2019)
摘要
Pedestrian Attribute Recognition (PAR) is an important task in surveillance video analysis. In this paper, we propose a novel end-to-end hierarchical deep learning approach to PAR. The proposed network introduces semantic segmentation into PAR and formulates it as a multi-task learning problem, which brings in pixel-level supervision in feature learning for attribute localization. According to the spatial properties of local and global attributes, we present a two stage learning mechanism to decouple coarse attribute localization and fine attribute recognition into successive phases within a single model, which strengthens feature learning. Besides, we design an attribute relationship attention module to efficiently capture and emphasize the latent relations among different attributes, further enhancing the discriminative power of the feature. Extensive experiments are conducted and very competitive results are reached on the RAP and PETA databases, indicating the effectiveness and superiority of the proposed approach.
更多查看译文
关键词
deep learning, multi-task learning, pedestrian attribute recognition, visual attention
AI 理解论文
溯源树
样例
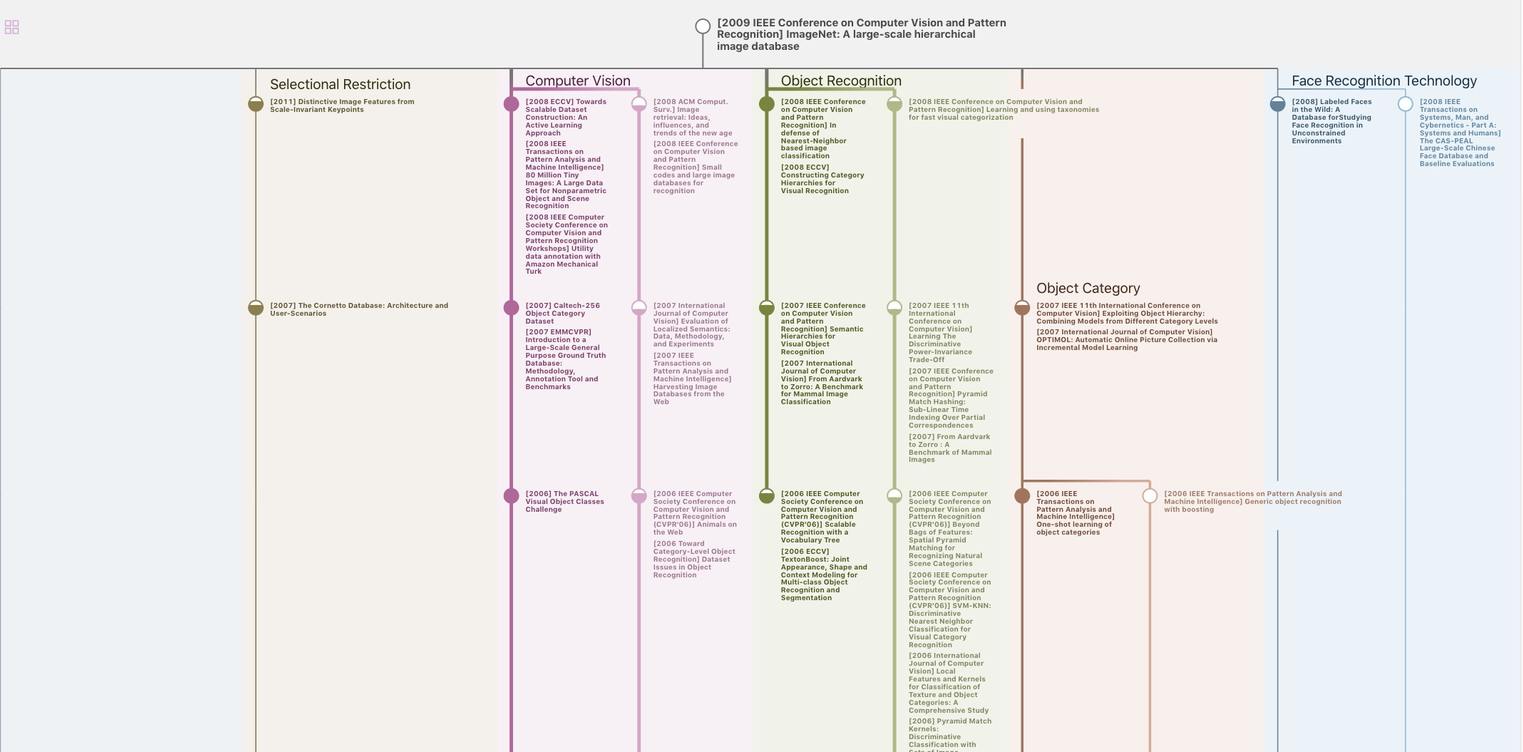
生成溯源树,研究论文发展脉络
Chat Paper
正在生成论文摘要