Multi-Classification Model for Spoken Language Understanding.
ICMI(2019)
摘要
The spoken language understanding (SLU) is an important part of spoken dialogue system (SDS). In the paper, we focus on how to extract a set of act-slot-value tuples from users’ utterances in the 1st Chinese Audio-Textual Spoken Language Understanding Challenge (CATSLU). This paper adopts the pretrained BERT model to encode users’ utterances and builds multiple classifiers to get the required tuples. In our framework, finding acts and values of slots are recognized as classification tasks respectively. Such multi-task training is expected to help the encoder to get better understanding of the utterance. Since the system is built on the transcriptions given by automatic speech recognition (ASR), some tricks are applied to correct the errors of the tuples. We also found that using the minimum edit distance (MED) between results and candidates to rebuild the tuples was beneficial in our experiments.
更多查看译文
关键词
Spoken language understanding, BERT, multi-task learning, classification, text tagging
AI 理解论文
溯源树
样例
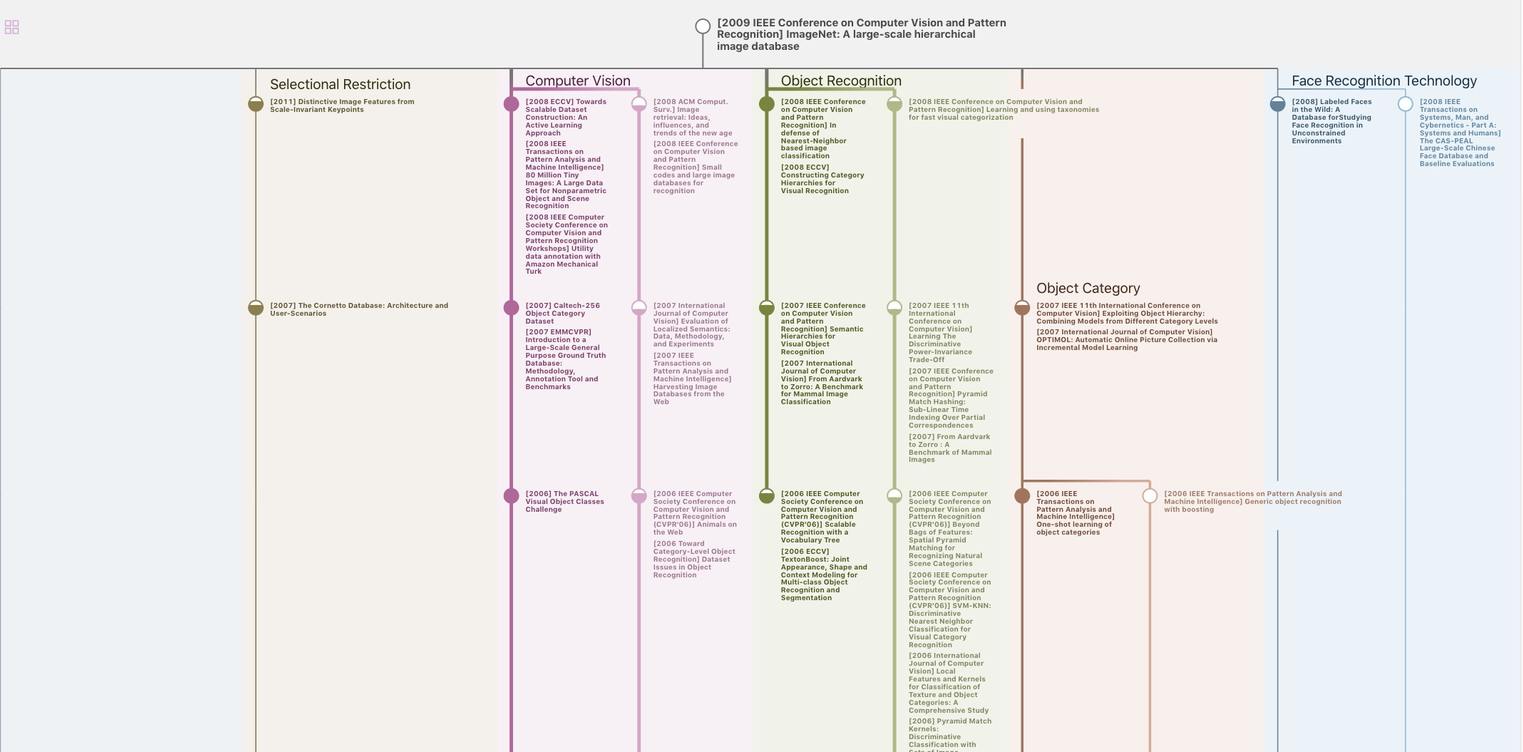
生成溯源树,研究论文发展脉络
Chat Paper
正在生成论文摘要