Sensitivity-Informed Provable Pruning of Neural Networks.
SIAM Journal on Mathematics of Data Science(2022)
摘要
We introduce a family of pruning algorithms that sparsifies the parameters of a trained model in a way that approximately preserves the model's predictive accuracy. Our algorithms use a small batch of input points to construct a data-informed importance sampling distribution over the network's parameters and use either a sampling-based or a deterministic pruning procedure, or an adaptive mixture of both, to discard redundant weights. Our methods are simultaneously computationally efficient, provably accurate, and broadly applicable to various network architectures and data dis-tributions. The presented approaches are simple to implement and can be easily integrated into standard prune-retrain pipelines. We present empirical comparisons showing that our algorithms reliably generate highly compressed networks that incur minimal loss in performance, regardless of whether the original network is fully trained or randomly initialized.
更多查看译文
关键词
Key words, compression, pruning, generalization
AI 理解论文
溯源树
样例
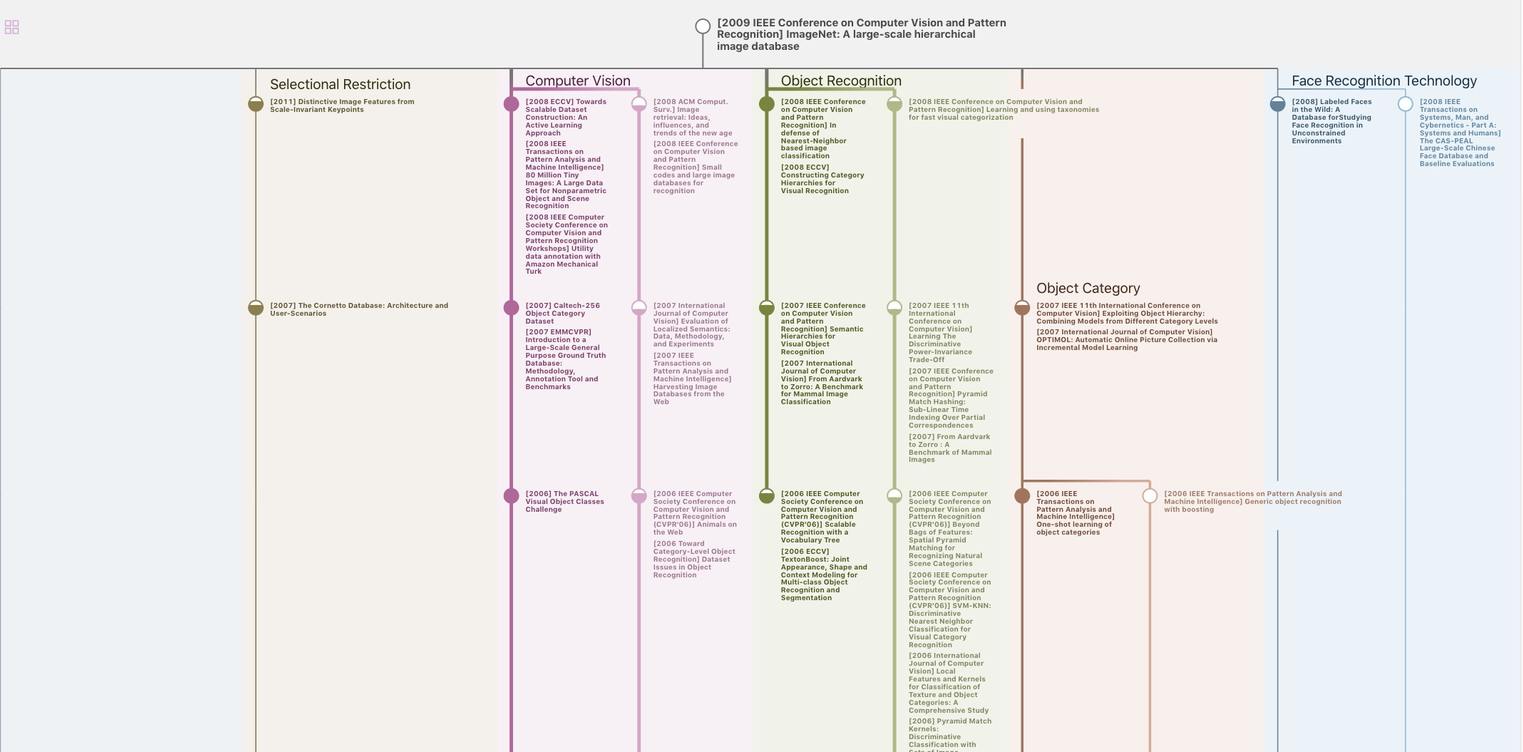
生成溯源树,研究论文发展脉络
Chat Paper
正在生成论文摘要