Misbehaviour prediction for autonomous driving systems
International Conference on Software Engineering(2020)
摘要
ABSTRACTDeep Neural Networks (DNNs) are the core component of modern autonomous driving systems. To date, it is still unrealistic that a DNN will generalize correctly to all driving conditions. Current testing techniques consist of offline solutions that identify adversarial or corner cases for improving the training phase. In this paper, we address the problem of estimating the confidence of DNNs in response to unexpected execution contexts with the purpose of predicting potential safety-critical misbehaviours and enabling online healing of DNN-based vehicles. Our approach SelfOracle is based on a novel concept of self-assessment oracle, which monitors the DNN confidence at runtime, to predict unsupported driving scenarios in advance. SelfOracle uses autoencoder-and time series-based anomaly detection to reconstruct the driving scenarios seen by the car, and to determine the confidence boundary between normal and unsupported conditions. In our empirical assessment, we evaluated the effectiveness of different variants of SelfOracle at predicting injected anomalous driving contexts, using DNN models and simulation environment from Udacity. Results show that, overall, SelfOracle can predict 77% misbehaviours, up to six seconds in advance, outperforming the online input validation approach of DeepRoad.
更多查看译文
关键词
misbehaviour prediction, testing, deep learning, anomaly detection
AI 理解论文
溯源树
样例
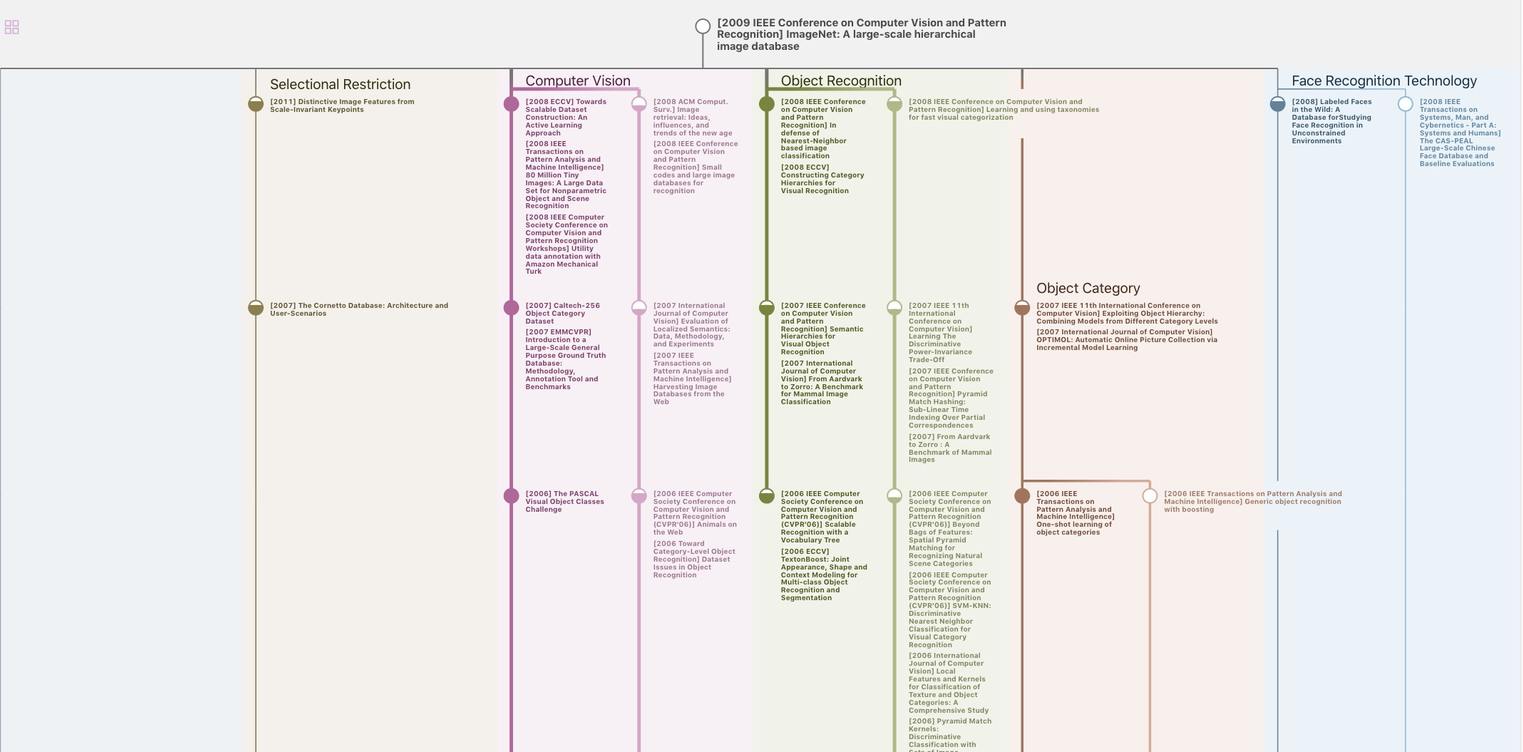
生成溯源树,研究论文发展脉络
Chat Paper
正在生成论文摘要