Distributed machine learning load balancing strategy in cloud computing services
WIRELESS NETWORKS(2019)
摘要
Mobile service computing is a new cloud computing model that provides various cloud services for mobile intelligent terminal users through mobile internet access. The quality of service is an essential problem faced by mobile service computing. In this paper, we demonstrate a series of research studies on how to accelerate the training of a distributed machine learning (ML) model based on cloud service. Distributed ML has become the mainstream way of today’s ML models training. In traditional distributed ML based on bulk synchronous parallel, the temporary slowdown of any node in the cluster will delay the calculation of other nodes because of the frequent occurrence of synchronous barriers, resulting in overall performance degradation. Our paper proposes a load balancing strategy named adaptive fast reassignment (AdaptFR). Based on this, we built a distributed parallel computing model called adaptive-dynamic synchronous parallel (A-DSP). A-DSP uses a more relaxed synchronization model to reduce the performance consumption caused by synchronous operations while ensuring the consistency of the model. At the same time, A-DSP also implements the AdaptFR load balancing strategy, which addresses the straggler problem caused by the performance difference between nodes under the premise of ensuring the accuracy of the model. The experiments show that A-DSP can effectively improve the training speed while ensuring the accuracy of the model in the distributed ML model training.
更多查看译文
关键词
Mobile service computing,Cloud service,Distributed machine learning,Load balancing,Adaptive fast reassignment
AI 理解论文
溯源树
样例
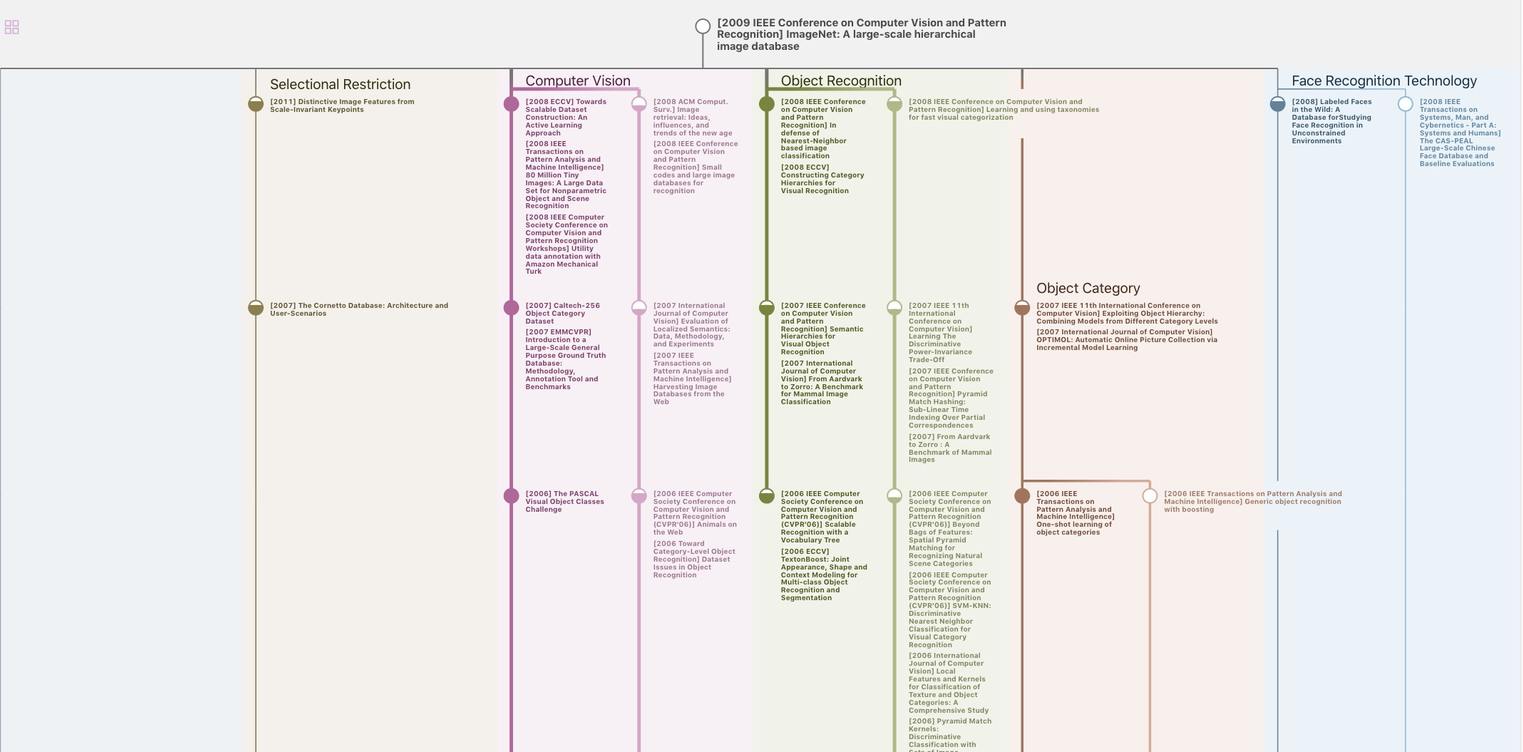
生成溯源树,研究论文发展脉络
Chat Paper
正在生成论文摘要