Testing Deep Learning for Deriving Stellar Atmospheric Parameters with Extended MILES Library
PUBLICATIONS OF THE ASTRONOMICAL SOCIETY OF THE PACIFIC(2019)
摘要
The stellar atmospheric parameters T-eff, log g, and [Fe/H] are important physical parameters. However, there are different ways to derive them using both spectroscopy and photometry. In this work, the extended MILES library has been convolved with the uvgri-band transmission of the Stellar Abundance and Galaxy Evolution Survey (SAGES), which aims to derive the stellar atmospheric parameters of 0.5 billion stars from observations. With the convolved SAGES magnitudes and MILES library, we examine the errors and stability of deep learning for deriving the stellar atmospheric parameters. The basic idea of our approach is to take photometric data such as images and train a deep neural network (DNN) with densely connected convolutional structure, which is widely used in the field of computer vision. Using this technique, stellar atmospheric parameters can be derived. Increasing the depth of the DNN makes it more difficult to train the DNN, but the dense connected convolutional structure can be a good solution to the gradient dispersion. Finally, we evaluate the performance of the proposed scheme with photometric data. Our method, trained by photometric data of only 483 stars, reduces the rms of T-eff, log g and [Fe/H] to 74 K, 0.11 dex and 0.06 dex, respectively in training and 110 K, 0.289 dex and 0.211 dex, respectively, in testing. It is worth noting that our method can directly calculate T-eff, log g and [Fe/H] continuously in range of similar to 4000-7000 K, similar to 2.0-5.0 dex and similar to-2.0-0.5 dex, respectively, rather than piecewise calculating the stellar atmospheric parameters in different stellar types and colors.
更多查看译文
关键词
methods: data analysis,stars: fundamental parameters,techniques: photometric
AI 理解论文
溯源树
样例
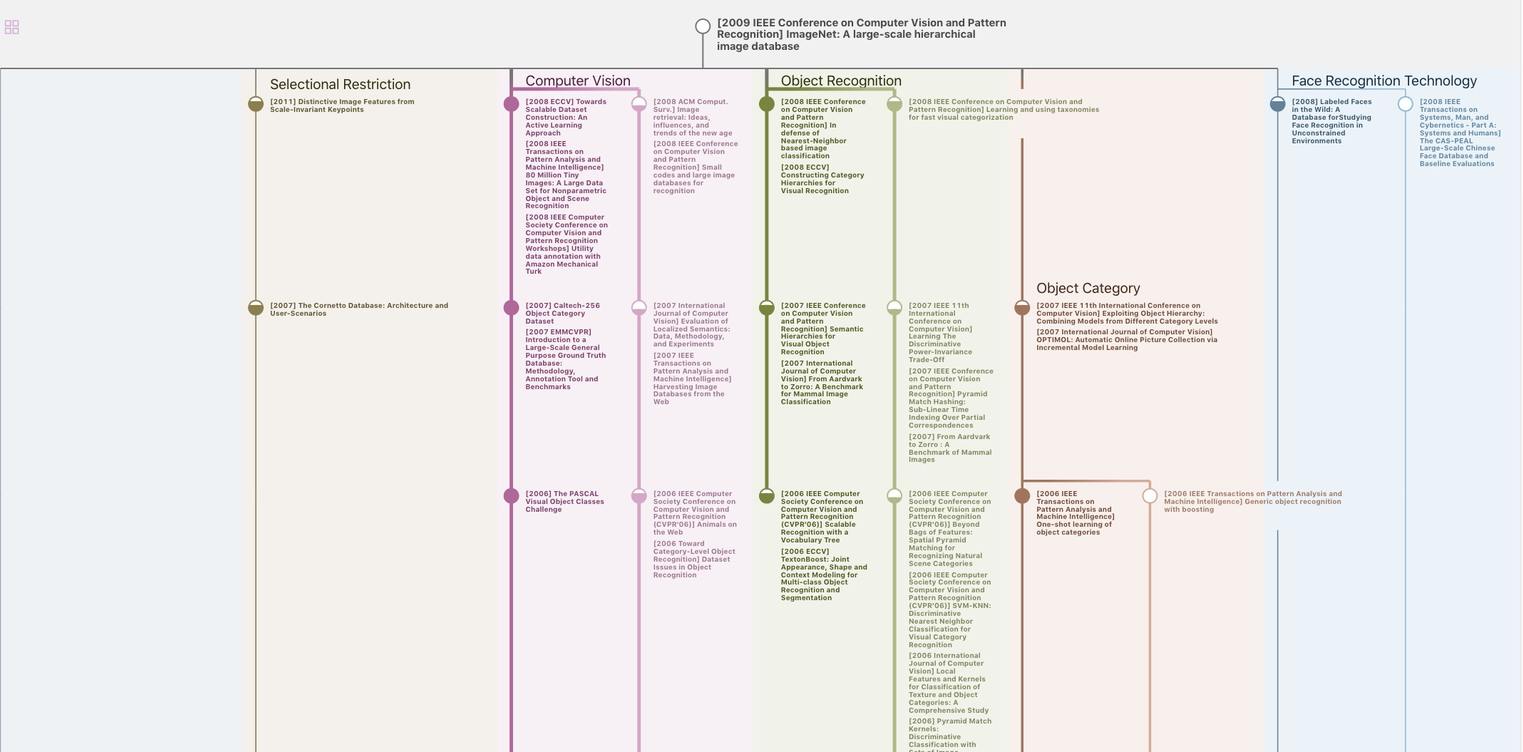
生成溯源树,研究论文发展脉络
Chat Paper
正在生成论文摘要