Splitting Steepest Descent for Growing Neural Architectures
ADVANCES IN NEURAL INFORMATION PROCESSING SYSTEMS 32 (NIPS 2019)(2019)
摘要
We develop a progressive training approach for neural networks which adaptively grows the network structure by splitting existing neurons to multiple off-springs. By leveraging a functional steepest descent idea, we derive a simple criterion for deciding the best subset of neurons to split and a splitting gradientfor optimally updating the off-springs. Theoretically, our splitting strategy is a second-order functional steepest descent for escaping saddle points in an infinity-Wasserstein metric space, on which the standard parametric gradient descent is a first-order steepest descent. Our method provides a new practical approach for optimizing neural network structures, especially for learning lightweight neural architectures in resource-constrained settings.
更多查看译文
关键词
neural networks,network structure
AI 理解论文
溯源树
样例
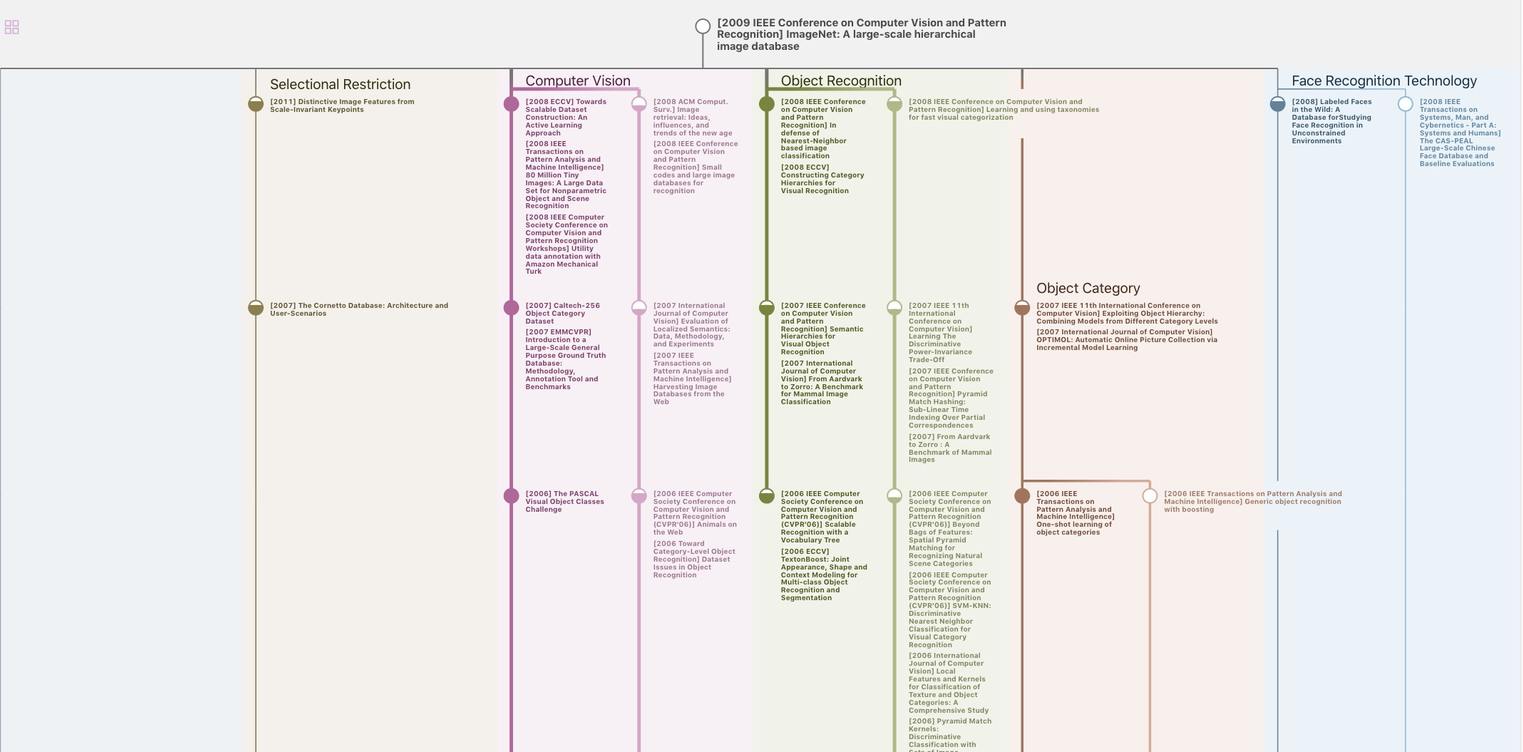
生成溯源树,研究论文发展脉络
Chat Paper
正在生成论文摘要