Representation Learning vs. Handcrafted Features for Music Genre Classification
2019 INTERNATIONAL JOINT CONFERENCE ON NEURAL NETWORKS (IJCNN)(2019)
摘要
In this work we present a comprehensive set of experiments aiming to perform music genre classification using learned and handcrafted features plus the fusion of them. Handcrafted features were obtained from the audio signal itself, lyrics, chords and spectrogram images extracted from the audio. The rationale behind this investigation is based on the assumption that one can find some complementarity between classifiers created from these different resources. The experimental protocol was conducted on the Brazilian Music Dataset using the artist filter restriction and they confirm the power of non-handcrafted features to perform audio classification tasks. The experimental results have shown a significant complementarity among the handcrafted features for which the evaluated fusion strategies allowed an improvement in the classification accuracy up to 4 percent points. On the other hand, the fusion of learned and handcrafted features provided similar accuracy than the best individual CNN (0.7815).
更多查看译文
关键词
Music genre classification, handcrafted features, non-handcrafted features
AI 理解论文
溯源树
样例
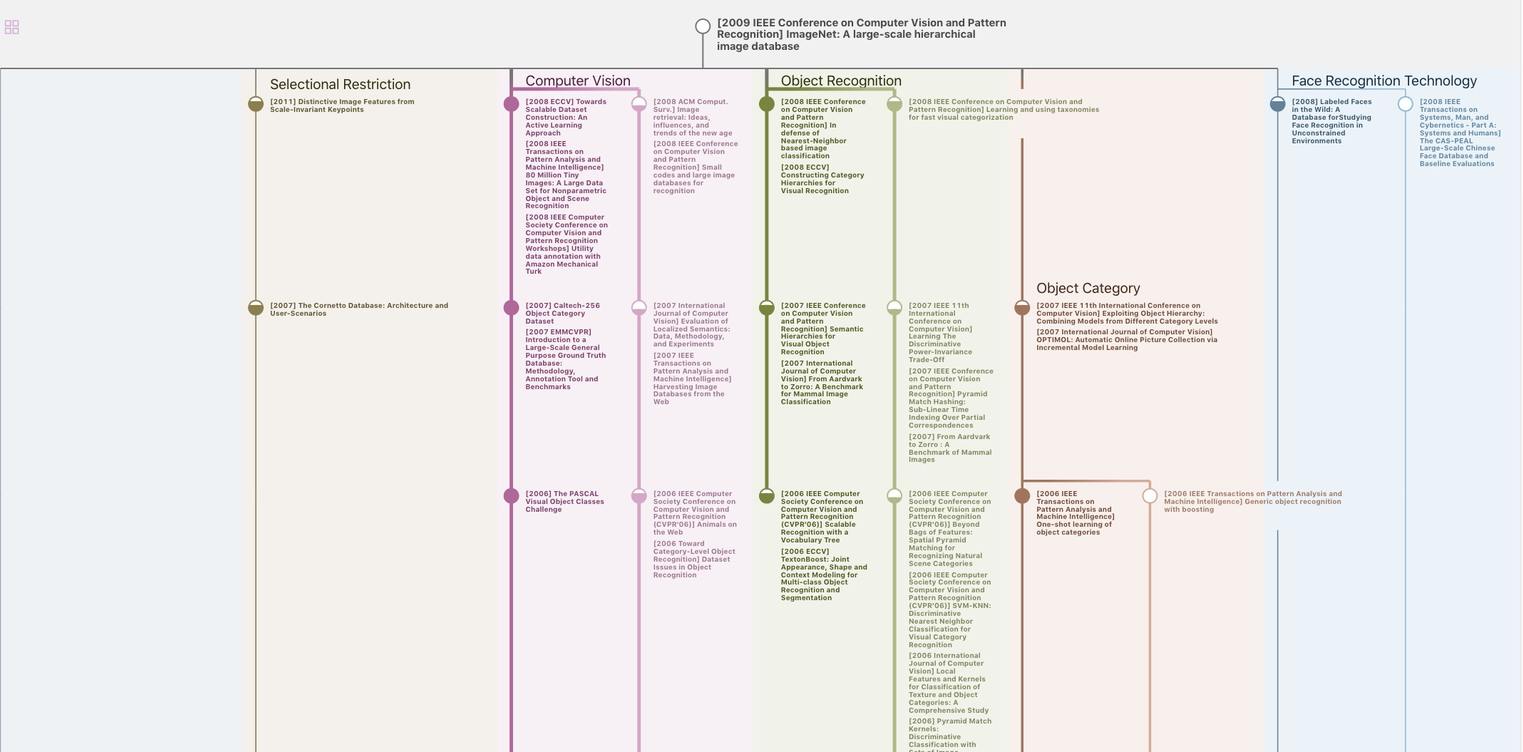
生成溯源树,研究论文发展脉络
Chat Paper
正在生成论文摘要