Non-Invasive In-Home Sleep Stage Classification Using A Ballistocardiography Bed Sensor
2019 IEEE EMBS INTERNATIONAL CONFERENCE ON BIOMEDICAL & HEALTH INFORMATICS (BHI)(2019)
摘要
Longitudinal monitoring of sleep related parameters can be used for early detection of diseases and also as an indication to physicians for effective adjustment of medication and dosage treatments for people at risk. The correlation between sleep disorders and health conditions such as Alzheimer's and Parkinson's diseases has already been reported in the literature. In this paper, we propose the use of a hydraulic bed sensor for sleep stage classification. Our main motivation of using the bed sensor is to provide a non-invasive, in-home monitoring system, which tracks the changes in health conditions of the subjects over time. Regular polysomnography data from a Sleep Lab have been used as the ground truth, with the focus on three sleep stages, namely, awake, rapid eye movement (REM) and non-REM sleep (NREM). A total of 74 features including heart rate variability (HRV) features, respiratory rate variability (RV) features, and linear frequency cepstral coefficients (LFCC) were extracted from the bed sensor data. Support Vector Machines (SVM) and K-Nearest Neighbors (KNN) classification methods were applied to these features. Our results show accuracy as good as 85% with 0.74 kappa, in the detection of these three sleep stages. These results show promise in the ability of the bed sensor to monitor and track sleep quality and sleep related disorders noninvasively.
更多查看译文
关键词
classification, sleep stages, in-home monitoring
AI 理解论文
溯源树
样例
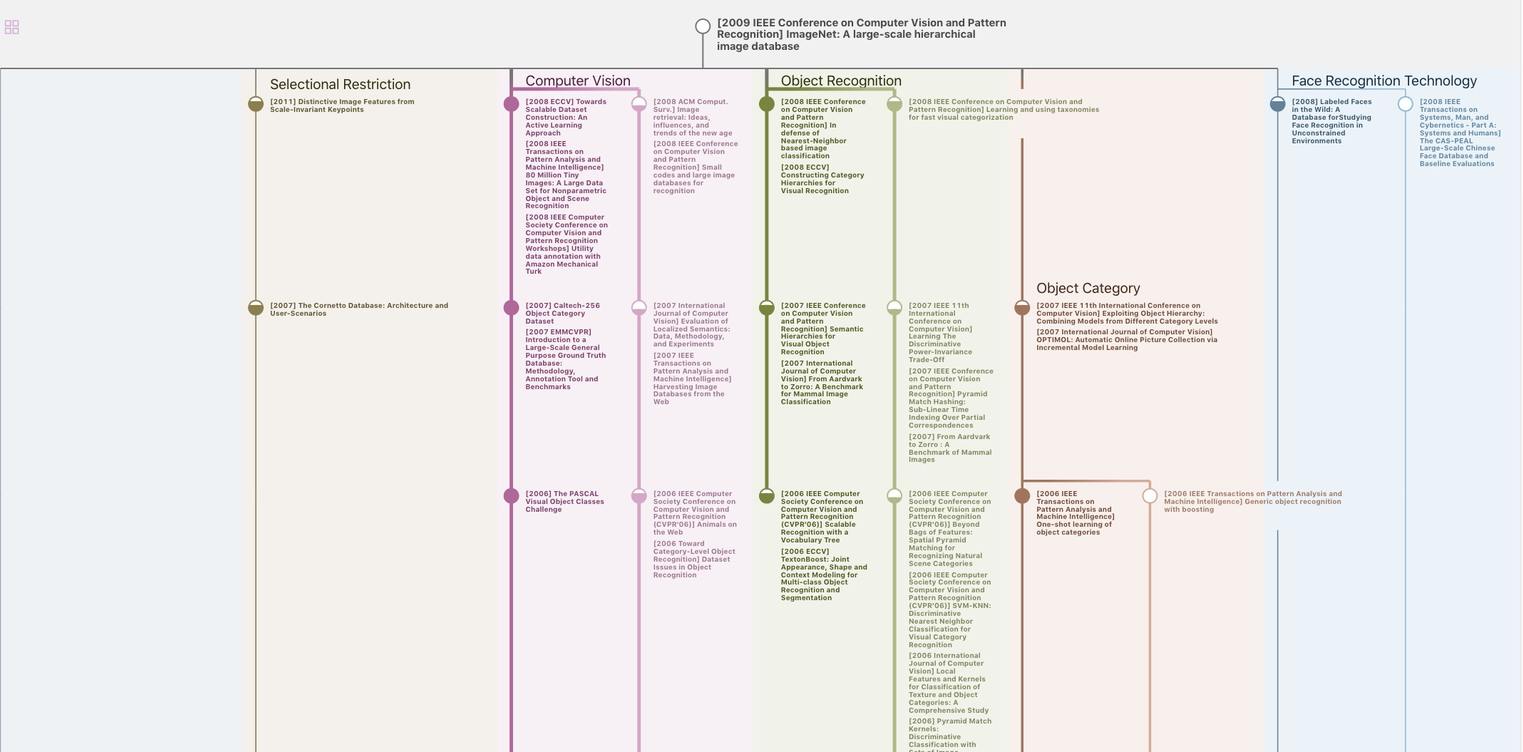
生成溯源树,研究论文发展脉络
Chat Paper
正在生成论文摘要