Sub-critical exponential random graphs: concentration of measure and some applications
TRANSACTIONS OF THE AMERICAN MATHEMATICAL SOCIETY(2024)
摘要
The exponential random graph model (ERGM) is a central object in the study of clustering properties in social networks as well as canonical ensembles in statistical physics. Despite some breakthrough works in the mathematical understanding of ERGM, most notably by Bhamidi, Bresler, and Sly [Ann. Appl. Probab. 21 (2011), pp. 2146-2170] through the analysis of a natural Heat-bath Glauber dynamics, and by Chatterjee and Diaconis [Ann. Statist. 41 (2013), pp. 2428-2461; Eldan [Geom. Funct. Anal. 28 (2018), pp. 1548-1596; and Eldan and Gross [Ann. Appl. Probab. 28 (2018), pp. 3698-3735] via a large deviation theoretic perspective, several basic questions have remained unanswered owing to the lack of exact solvability unlike the much studied Curie-Weiss model (Ising model on the complete graph). In this paper, we establish a series of new concentration of measure results for the ERGM throughout the entire sub-critical phase, including a Poincar ' e inequality, Gaussian concentration for Lipschitz functions, and a central limit theorem. In addition, a new proof of a quantitative bound on the W-1- Wasserstein distance to Erd.os-R ' enyi graphs, previously obtained by Reinert and Ross [Ann. Appl. Probab. 29 (2019), pp. 3201-3229], is also presented. The arguments rely on translating temporal mixing properties of Glauber dynamics to static spatial mixing properties of the equilibrium measure and have the potential of being useful in proving similar functional inequalities for other Gibbsian systems beyond the perturbative regime.
更多查看译文
关键词
Exponential random graphs,Erdos-Renyi graph,Glauber dynamics,mixing of Markov chains,Poincare inequality,concentration,central limit theorem,Stein's method,Wasserstein distance
AI 理解论文
溯源树
样例
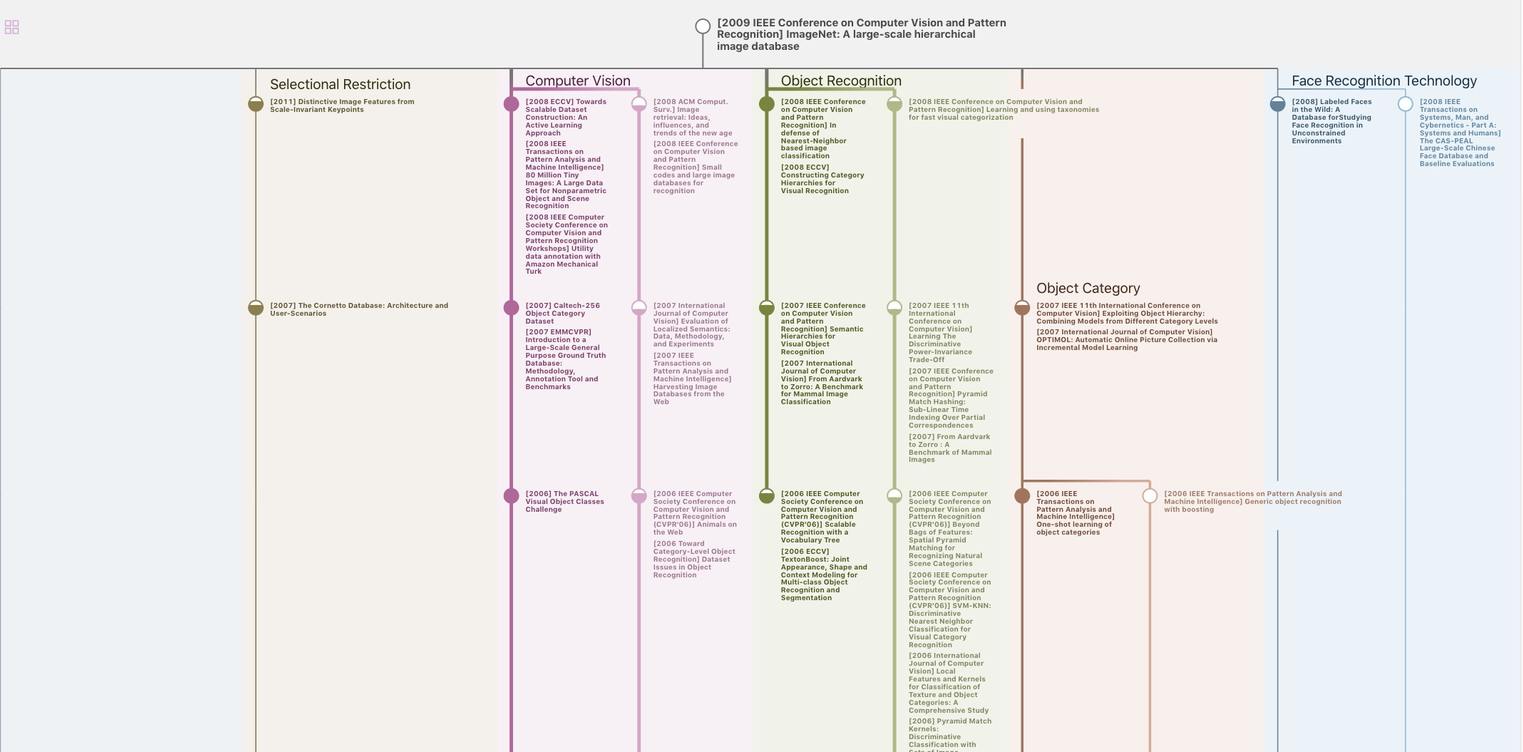
生成溯源树,研究论文发展脉络
Chat Paper
正在生成论文摘要