Stochastic Iterative Hard Thresholding for Low-Tucker-Rank Tensor Recovery
2020 Information Theory and Applications Workshop (ITA)(2020)
摘要
Low-rank tensor recovery problems have been widely studied in many signal processing and machine learning applications. Tensor rank is typically defined under certain tensor decomposition. In particular, Tucker decomposition is known as one of the most popular tensor decompositions. In recent years, researchers have developed many state-of-the-art algorithms to address the problem of low-Tucker-rank tensor recovery. Motivated by the favorable properties of the stochastic algorithms, such as stochastic gradient descent and stochastic iterative hard thresholding, we aim to extend the stochastic iterative hard thresholding algorithm from vectors to tensors in order to address the problem of recovering a low-Tucker-rank tensor from its linear measurements. We have also developed linear convergence analysis for the proposed method and conducted a series of experiments with both synthetic and real data to illustrate the performance of the proposed method.
更多查看译文
关键词
tensor rank,tensor decomposition,Tucker decomposition,low-Tucker-rank tensor recovery,stochastic algorithms,stochastic iterative hard thresholding algorithm
AI 理解论文
溯源树
样例
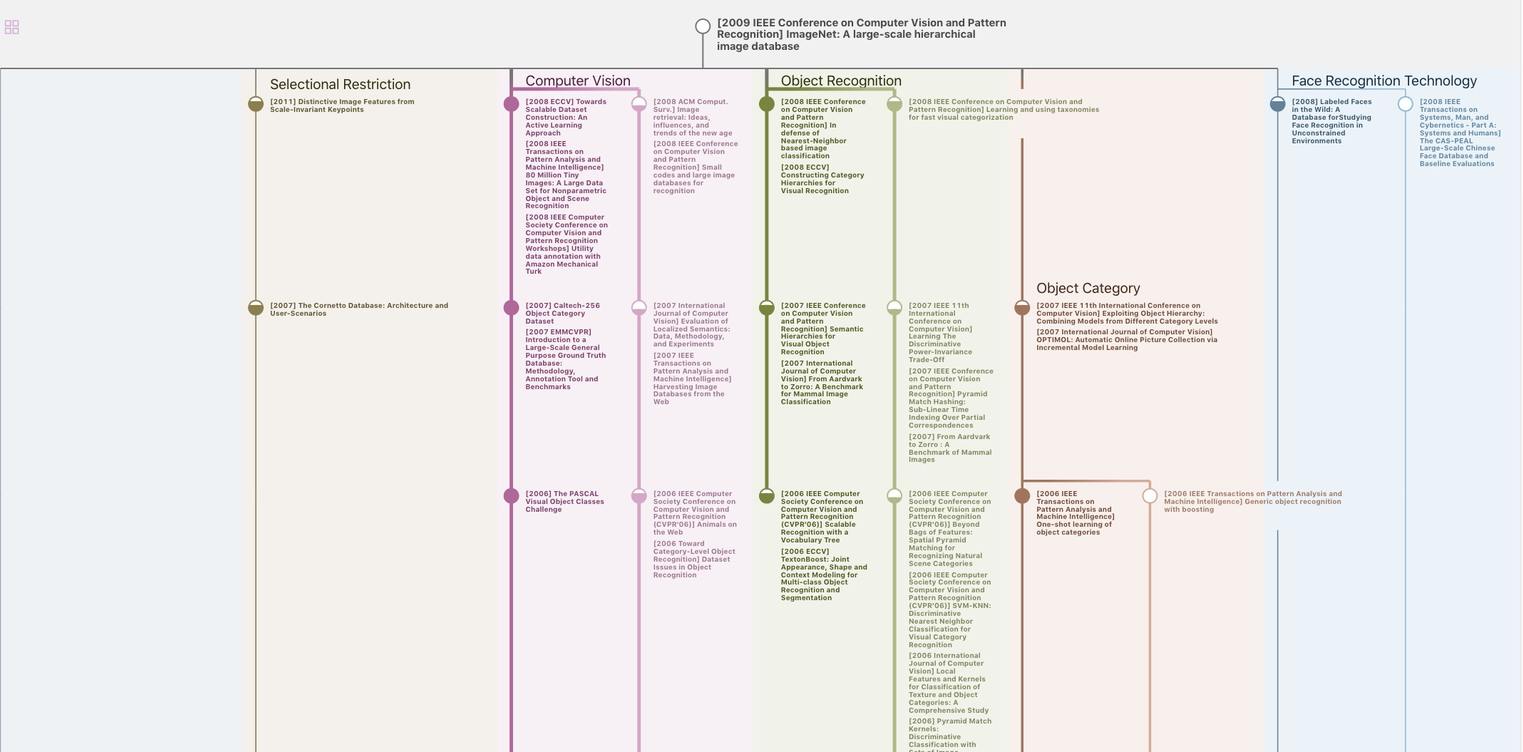
生成溯源树,研究论文发展脉络
Chat Paper
正在生成论文摘要