A Simple yet Effective Baseline for Robust Deep Learning with Noisy Labels
arxiv(2019)
摘要
Recently deep neural networks have shown their capacity to memorize training data, even with noisy labels, which hurts generalization performance. To mitigate this issue, we propose a simple but effective baseline that is robust to noisy labels, even with severe noise. Our objective involves a variance regularization term that implicitly penalizes the Jacobian norm of the neural network on the whole training set (including the noisy-labeled data), which encourages generalization and prevents overfitting to the corrupted labels. Experiments on both synthetically generated incorrect labels and realistic large-scale noisy datasets demonstrate that our approach achieves state-of-the-art performance with a high tolerance to severe noise.
更多查看译文
关键词
robust deep learning,labels,effective baseline,noisy
AI 理解论文
溯源树
样例
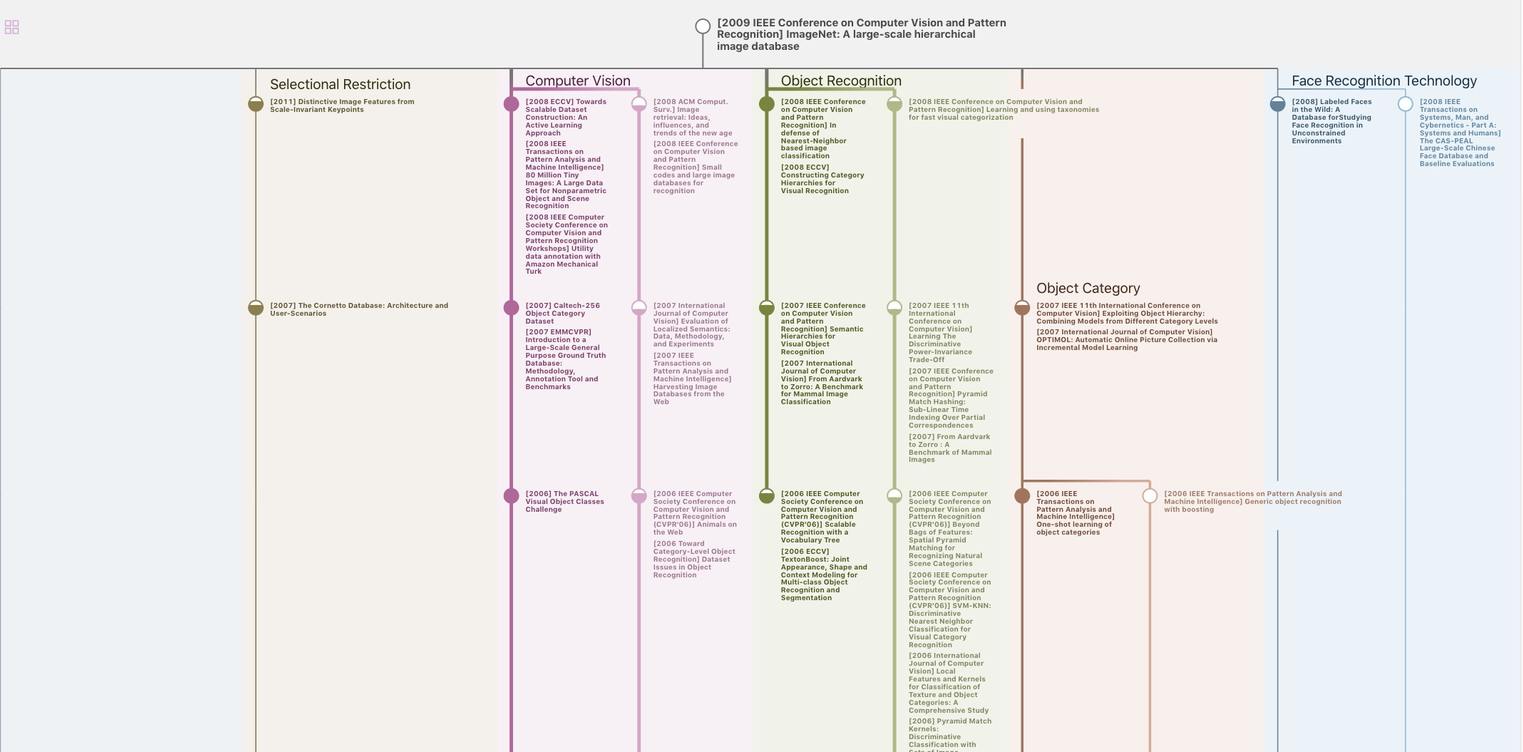
生成溯源树,研究论文发展脉络
Chat Paper
正在生成论文摘要