Fast Algorithms at Low Temperatures via Markov Chains.
arXiv: Data Structures and Algorithms(2019)
摘要
Efficient algorithms for approximate counting and sampling in spin systems typically apply in the so-called high-temperature regime, where the interaction between neighboring spins is "weak." Instead, recent work of Jenssen, Keevash, and Perkins yields polynomial-time algorithms in the low-temperature regime on bounded-degree (bipartite) expander graphs using polymer models and the cluster expansion. In order to speed up these algorithms (so the exponent in the run time does not depend on the degree bound) we present a Markov chain for polymer models and show that it is rapidly mixing under exponential decay of polymer weights. This yields, for example, anO(nlogn)-time sampling algorithm for the low-temperature ferromagnetic Potts model on bounded-degree expander graphs. Combining our results for the hard-core and Potts models with Markov chain comparison tools, we obtain polynomial mixing time for Glauber dynamics restricted to appropriate portions of the state space.
更多查看译文
关键词
approximate counting, expander graphs, hard-core model, Markov chains, Potts model
AI 理解论文
溯源树
样例
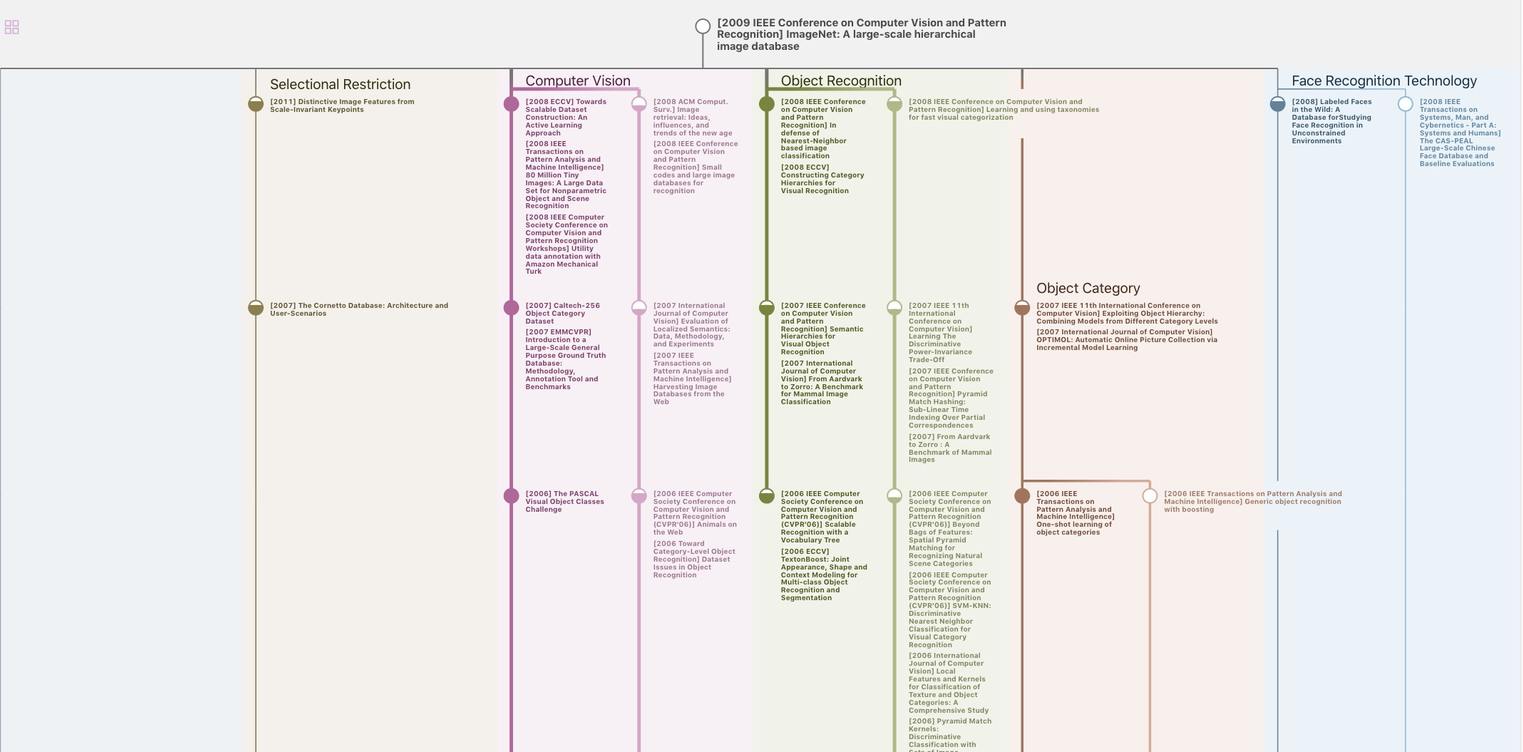
生成溯源树,研究论文发展脉络
Chat Paper
正在生成论文摘要