An Investigation into On-Device Personalization of End-to-End Automatic Speech Recognition Models
CoRR(2019)
摘要
Speaker-independent speech recognition systems trained with data from many users are generally robust against speaker variability and work well for a large population of speakers. However, these systems do not always generalize well for users with very different speech characteristics. This issue can be addressed by building personalized systems that are designed to work well for each specific user. In this paper, we investigate the idea of securely training personalized end-to-end speech recognition models on mobile devices so that user data and models never leave the device and are never stored on a server. We study how the mobile training environment impacts performance by simulating on-device data consumption. We conduct experiments using data collected from speech impaired users for personalization. Our results show that personalization achieved 63.7\% relative word error rate reduction when trained in a server environment and 58.1% in a mobile environment. Moving to on-device personalization resulted in 18.7% performance degradation, in exchange for improved scalability and data privacy. To train the model on device, we split the gradient computation into two and achieved 45% memory reduction at the expense of 42% increase in training time.
更多查看译文
关键词
speech recognition, personalization, adaptation
AI 理解论文
溯源树
样例
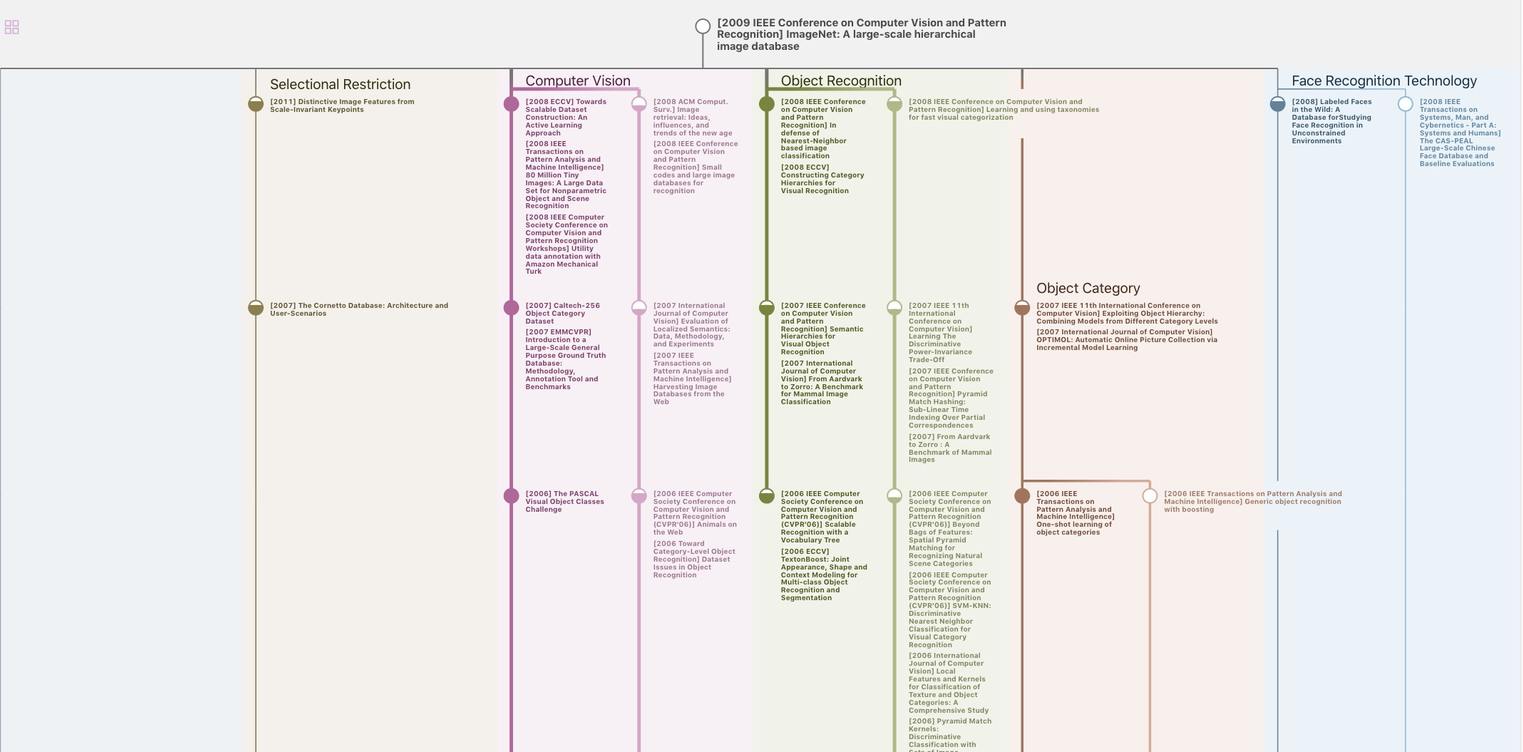
生成溯源树,研究论文发展脉络
Chat Paper
正在生成论文摘要