Collaborative Denoising Autoencoder For High Glycated Haemoglobin Prediction
ARTIFICIAL NEURAL NETWORKS AND MACHINE LEARNING - ICANN 2019: WORKSHOP AND SPECIAL SESSIONS(2019)
摘要
A pioneering study is presented demonstrating that the presence of high glycated haemoglobin (HbA1c) levels in a patient's blood can be reliably predicted from routinely collected clinical data. This paves the way for performing early detection of Type-2 Diabetes Mellitus (T2DM). This will save healthcare providers a major cost associated with the administration and assessment of clinical tests for HbA1c. A novel collaborative denoising autoencoder framework is used to address this challenge. The framework builds an independent denoising autoencoder model for the high and low HbA1c level, which extracts feature representations in the latent space. A baseline model using just three features: patient age together with triglycerides and glucose level achieves 76% F1-score with an SVM classifier. The collaborative denoising autoencoder uses 78 features and can predict HbA1c level with 81% F1-score.
更多查看译文
关键词
Healthcare, Machine learning, Deep learning, Collaborative denoising autoencoder, Clinical data, Diabetes, Type-2 Diabetes Mellitus, Glycated haemoglobin, HbA1c, KAIMRC dataset
AI 理解论文
溯源树
样例
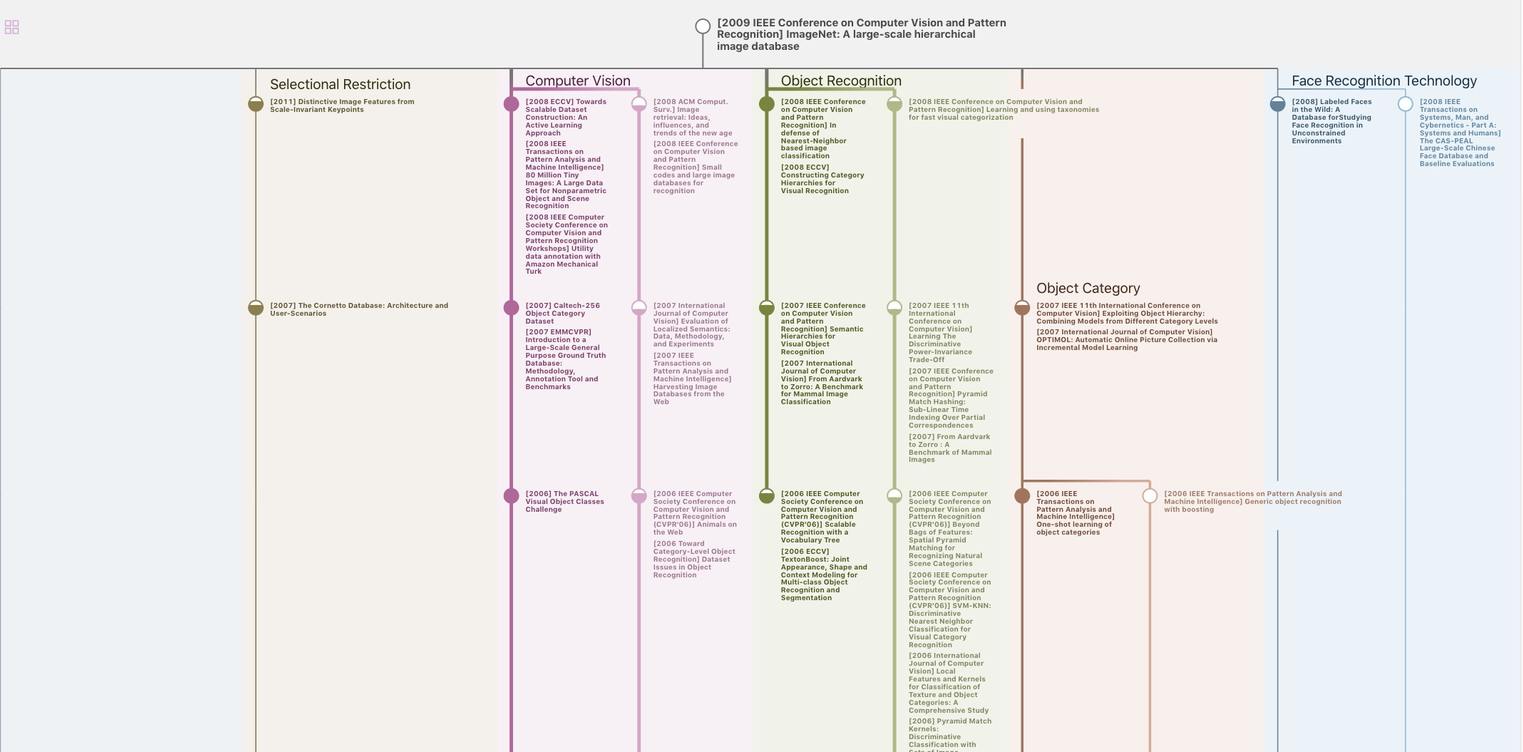
生成溯源树,研究论文发展脉络
Chat Paper
正在生成论文摘要