Generative Speech Enhancement Based on Cloned Networks
2019 IEEE Workshop on Applications of Signal Processing to Audio and Acoustics (WASPAA)(2019)
摘要
We propose to implement speech enhancement by the regeneration of clean speech from a `salient' representation extracted from the noisy signal. The network that extracts salient features is trained using a set of weight-sharing clones of the extractor network. The clones receive mel-frequency spectra of different noisy versions of the same speech signal as input. By encouraging the outputs of the clones to be similar for these different input signals, we train a feature extractor network that is robust to noise. At inference, the salient features form the input to a WaveNet network that generates a natural and clean speech signal with the same attributes as the ground-truth clean signal. As the signal becomes noisier, our system produces natural sounding errors that stay on the speech manifold, in place of traditional artifacts found in other systems. Our experiments confirm that our generative enhancement system provides state-of-the-art enhancement performance within the generative class of enhancers according to a MUSHRA-like test. The clones based system matches or outperforms the other systems at each input signal-to-noise (SNR) range with statistical significance.
更多查看译文
关键词
speech enhancement,learned representations,generative model
AI 理解论文
溯源树
样例
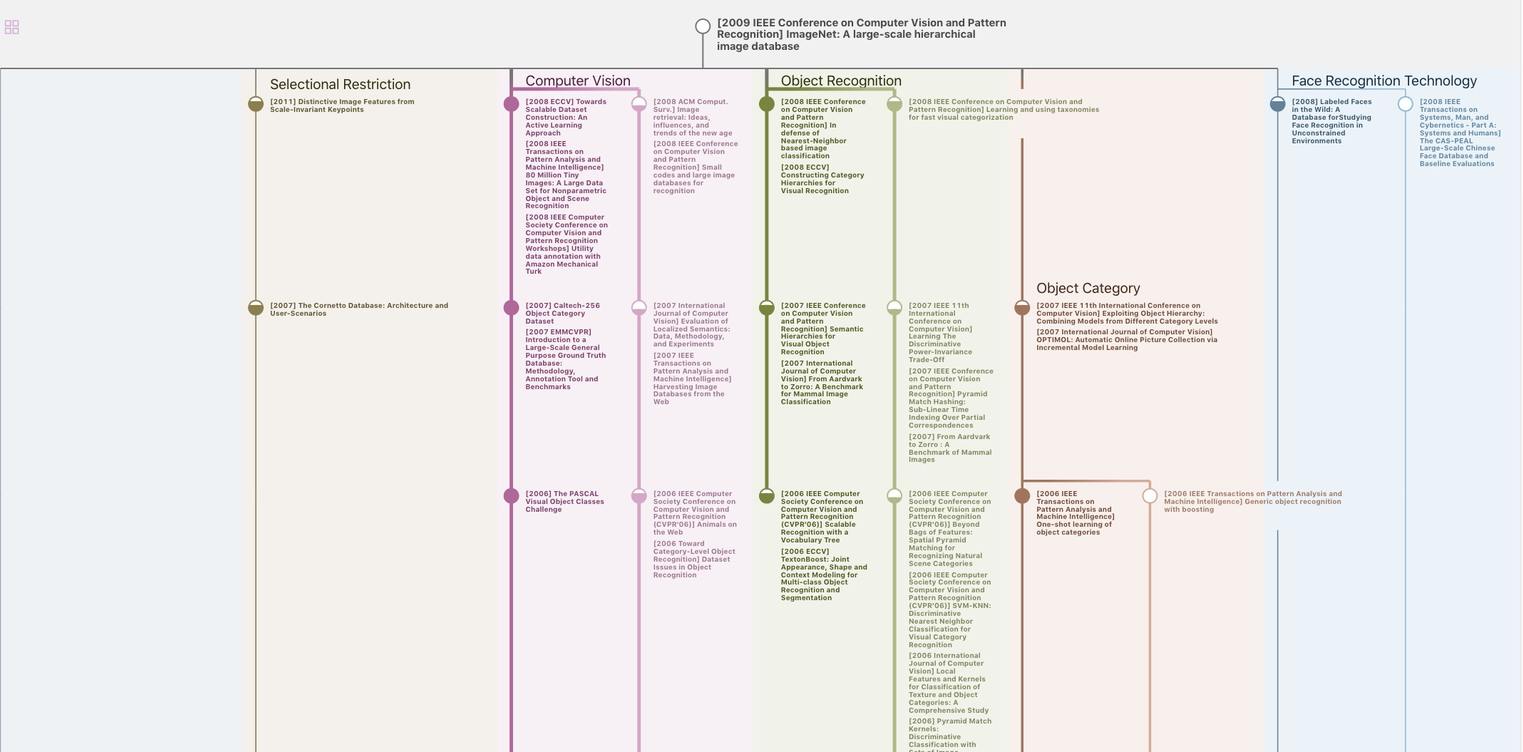
生成溯源树,研究论文发展脉络
Chat Paper
正在生成论文摘要