Learning Transferable Domain Priors For Safe Exploration In Reinforcement Learning
2020 INTERNATIONAL JOINT CONFERENCE ON NEURAL NETWORKS (IJCNN)(2020)
摘要
Prior access to domain knowledge could significantly improve the performance of a reinforcement learning agent. In particular, it could help agents avoid potentially catastrophic exploratory actions, which would otherwise have to be experienced during learning. In this work, we identify consistently undesirable actions in a set of previously learned tasks, and use pseudo-rewards associated with them to learn a prior policy. In addition to enabling safer exploratory behaviors in subsequent tasks in the domain, we show that these priors are transferable to similar environments, and can be learned offpolicy and in parallel with the learning of other tasks in the domain. We compare our approach to established, state-of-the-art algorithms in both discrete as well as continuous environments, and demonstrate that it exhibits a safer exploratory behavior while learning to perform arbitrary tasks in the domain. We also present a theoretical analysis to support these results, and briefly discuss the implications and some alternative formulations of this approach, which could also be useful in certain scenarios.
更多查看译文
关键词
safe exploration, domain priors, reinforcement learning
AI 理解论文
溯源树
样例
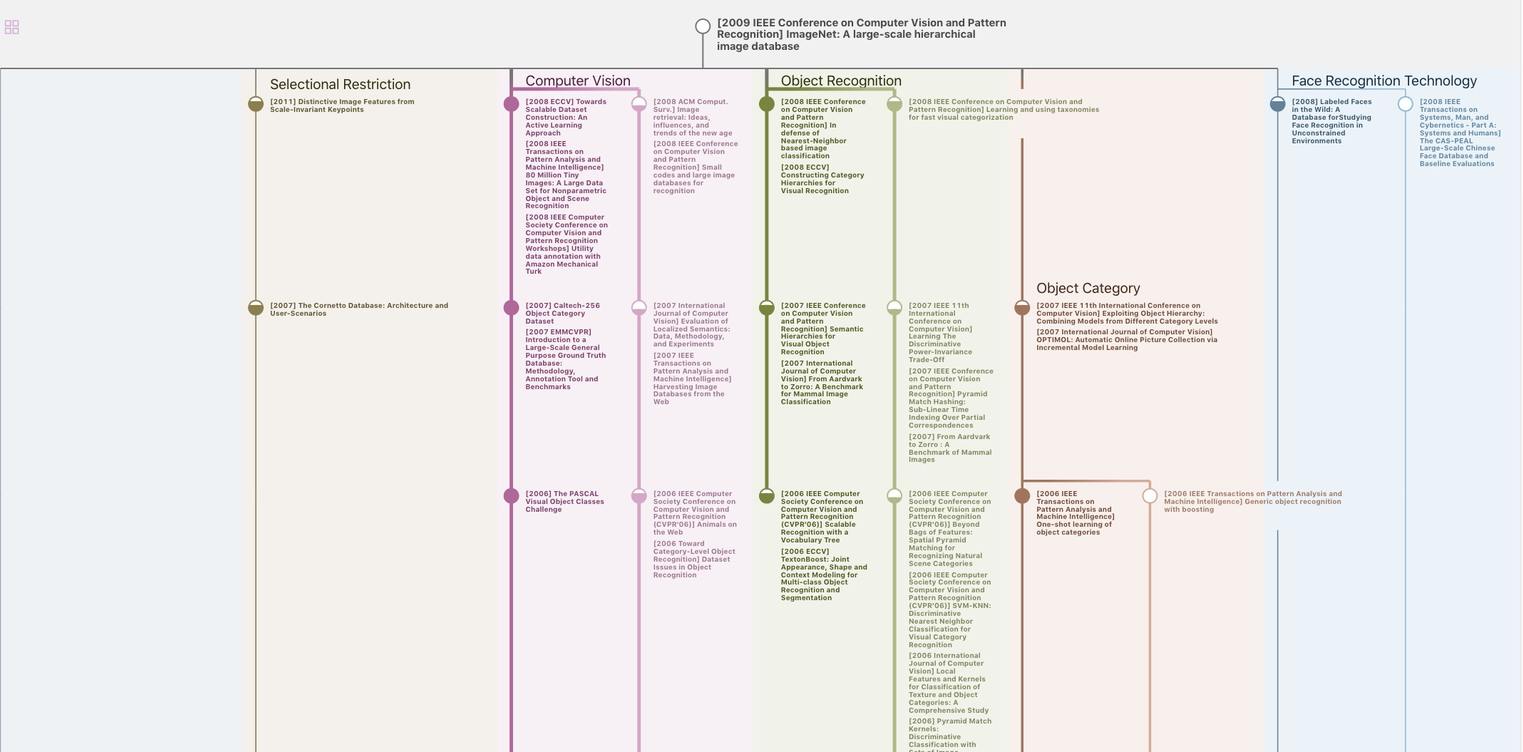
生成溯源树,研究论文发展脉络
Chat Paper
正在生成论文摘要