Multi-Step Greedy and Approximate Real Time Dynamic Programming
arxiv(2019)
摘要
Real Time Dynamic Programming (RTDP) is a well-known Dynamic Programming (DP) based algorithm that combines planning and learning to find an optimal policy for an MDP. It is a planning algorithm because it uses the MDP's model (reward and transition functions) to calculate a 1-step greedy policy w.r.t.~an optimistic value function, by which it acts. It is a learning algorithm because it updates its value function only at the states it visits while interacting with the environment. As a result, unlike DP, RTDP does not require uniform access to the state space in each iteration, which makes it particularly appealing when the state space is large and simultaneously updating all the states is not computationally feasible. In this paper, we study a generalized multi-step greedy version of RTDP, which we call $h$-RTDP, in its exact form, as well as in three approximate settings: approximate model, approximate value updates, and approximate state abstraction. We analyze the sample, computation, and space complexities of $h$-RTDP and establish that increasing $h$ improves sample and space complexity, with the cost of additional offline computational operations. For the approximate cases, we prove that the asymptotic performance of $h$-RTDP is the same as that of a corresponding approximate DP -- the best one can hope for without further assumptions on the approximation errors. $h$-RTDP is the first algorithm with a provably improved sample complexity when increasing the lookahead horizon.
更多查看译文
AI 理解论文
溯源树
样例
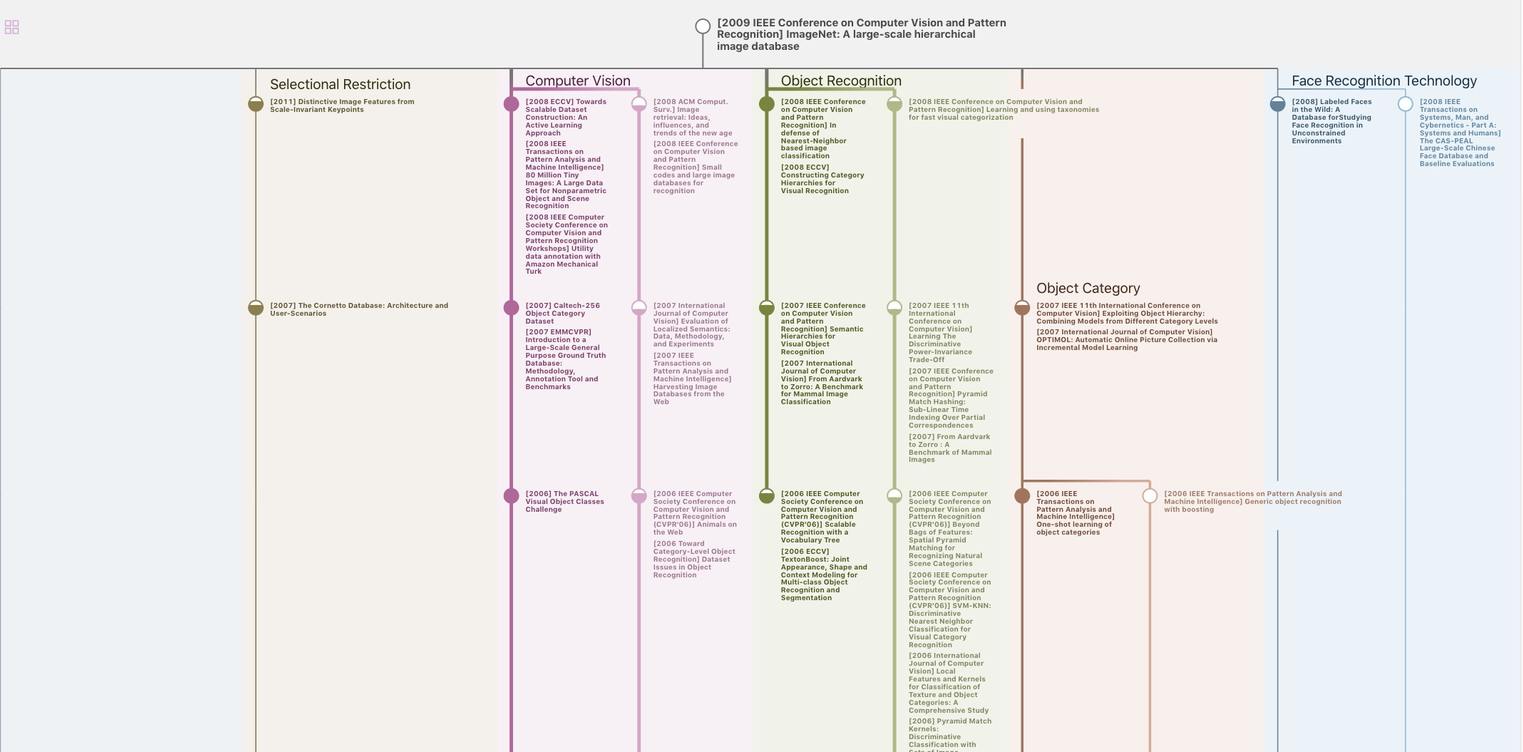
生成溯源树,研究论文发展脉络
Chat Paper
正在生成论文摘要