Deep Learning for Soil and Crop Segmentation from Remotely Sensed Data.
REMOTE SENSING(2019)
摘要
One of the most challenging problems in precision agriculture is to correctly identify and separate crops from the soil. Current precision farming algorithms based on artificially intelligent networks use multi-spectral or hyper-spectral data to derive radiometric indices that guide the operational management of agricultural complexes. Deep learning applications using these big data require sensitive filtering of raw data to effectively drive their hidden layer neural network architectures. Threshold techniques based on the normalized difference vegetation index (NDVI) or other similar metrics are generally used to simplify the development and training of deep learning neural networks. They have the advantage of being natural transformations of hyper-spectral or multi-spectral images that filter the data stream into a neural network, while reducing training requirements and increasing system classification performance. In this paper, to calculate a detailed crop/soil segmentation based on high resolution Digital Surface Model (DSM) data, we propose the redefinition of the radiometric index using a directional mathematical filter. To further refine the analysis, we feed this new radiometric index image of about 3500 x 4500 pixels into a relatively small Convolution Neural Network (CNN) designed for general image pattern recognition at 28 x 28 pixels to evaluate and resolve the vegetation correctly. We show that the result of applying a DSM filter to the NDVI radiometric index before feeding it into a Convolutional Neural Network can potentially improve crop separation hit rate by 65%.
更多查看译文
关键词
precision agriculture,digital surface model,multi-spectral images,CNN,UAV
AI 理解论文
溯源树
样例
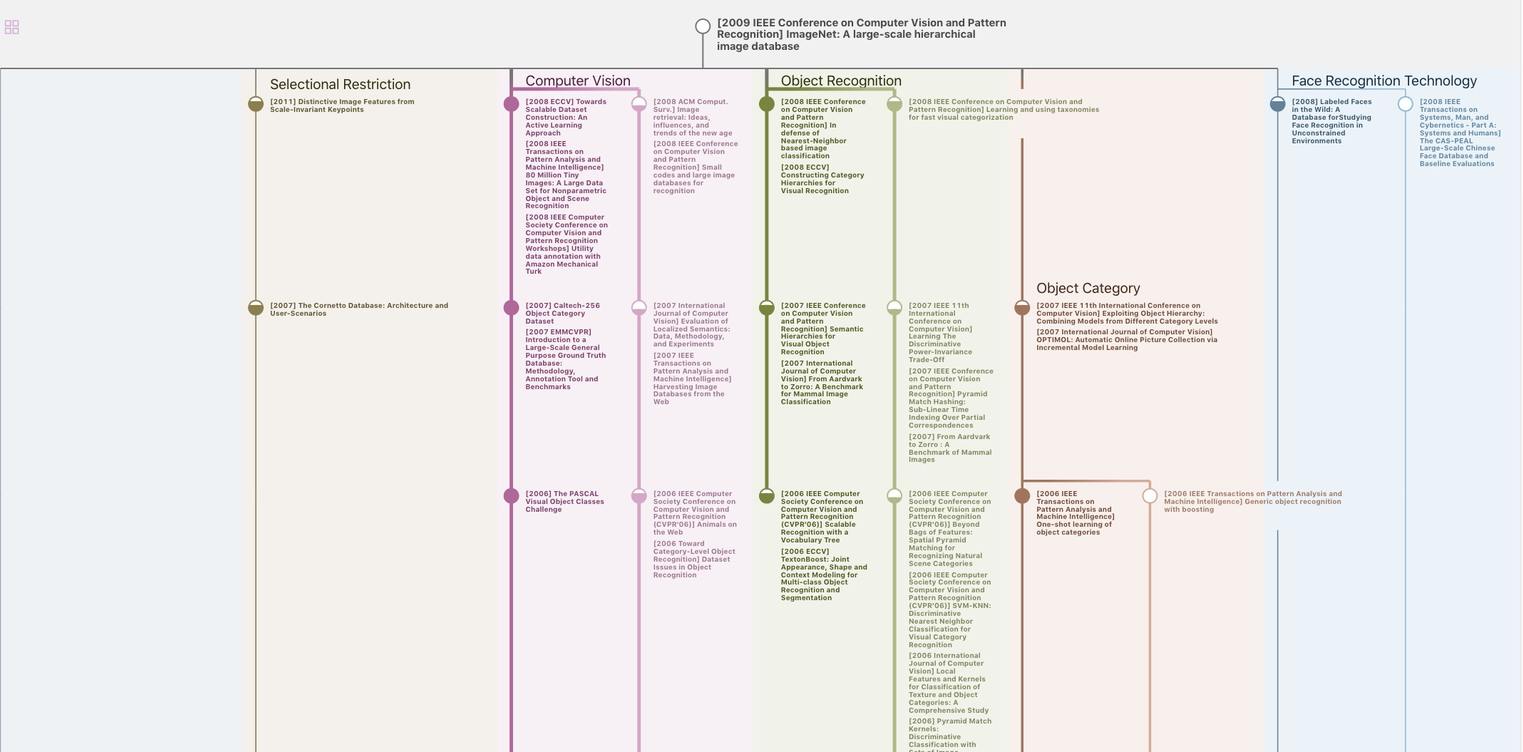
生成溯源树,研究论文发展脉络
Chat Paper
正在生成论文摘要