Efficient Answer-Annotation For Frequent Questions
EXPERIMENTAL IR MEETS MULTILINGUALITY, MULTIMODALITY, AND INTERACTION (CLEF 2019)(2019)
摘要
Ground truth is a crucial resource for the creation of effective question-answering (Q-A) systems. When no appropriate ground truth is available, as it is often the case in domain-specific Q-A systems (e.g. customer-support, tourism) or in languages other than English, new ground truth can be created by human annotation. The annotation process in which a human annotator looks up the corresponding answer label for each question from an answer catalog (Sequential approach), however, is usually time-consuming and costly. In this paper, we propose a new approach, in which the annotator first manually groups questions that have the same intent as a candidate question, and then, labels the entire group in one step (Group-Wise approach). To retrieve same-intent questions effectively, we evaluate various unsupervised semantic similarity methods from recent literature, and implement the most effective one in our annotation approach. Afterwards, we compare the Group-Wise approach with the Sequential approach with respect to answer look-ups, annotation time, and label-quality. We show based on 500 German customer-support questions that the Group-Wise approach requires 51% fewer answer look-ups, is 41% more time-efficient, and retains the same label-quality as the Sequential approach. Note that the described approach is limited to Q-A systems where frequently asked questions occur.
更多查看译文
关键词
Question-answering, Unsupervised semantic text similarity, Data annotation
AI 理解论文
溯源树
样例
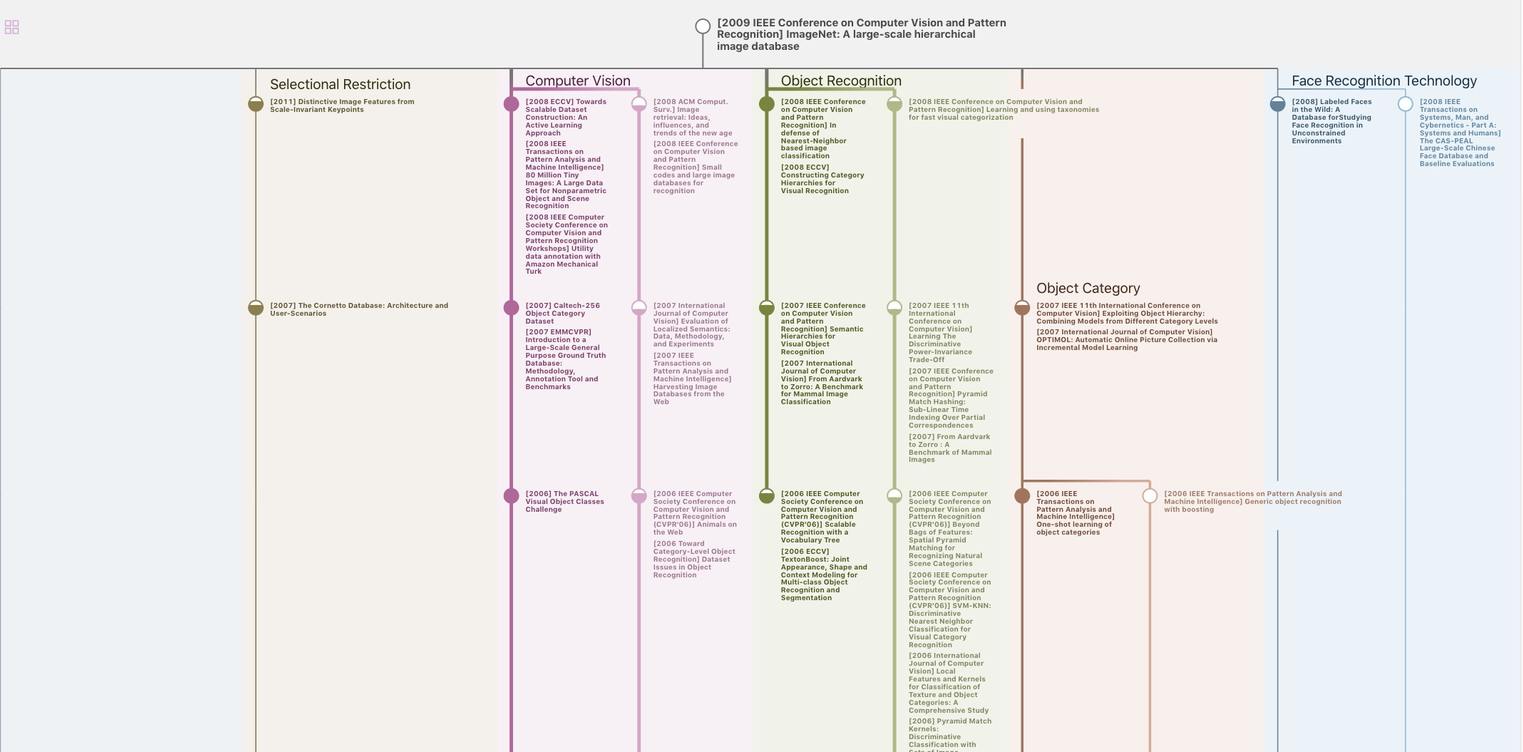
生成溯源树,研究论文发展脉络
Chat Paper
正在生成论文摘要