On The Rate Of Convergence Of Fully Connected Deep Neural Network Regression Estimates
ANNALS OF STATISTICS(2021)
摘要
Recent results in nonparametric regression show that deep learning, that is, neural network estimates with many hidden layers, are able to circumvent the so-called curse of dimensionality in case that suitable restrictions on the structure of the regression function hold. One key feature of the neural networks used in these results is that their network architecture has a further constraint, namely the network sparsity. In this paper, we show that we can get similar results also for least squares estimates based on simple fully connected neural networks with ReLU activation functions. Here, either the number of neurons per hidden layer is fixed and the number of hidden layers tends to infinity suitably fast for sample size tending to infinity, or the number of hidden layers is bounded by some logarithmic factor in the sample size and the number of neurons per hidden layer tends to infinity suitably fast for sample size tending to infinity. The proof is based on new approximation results concerning deep neural networks.
更多查看译文
关键词
Curse of dimensionality, deep learning, neural networks, nonparametric regression, rate of convergence
AI 理解论文
溯源树
样例
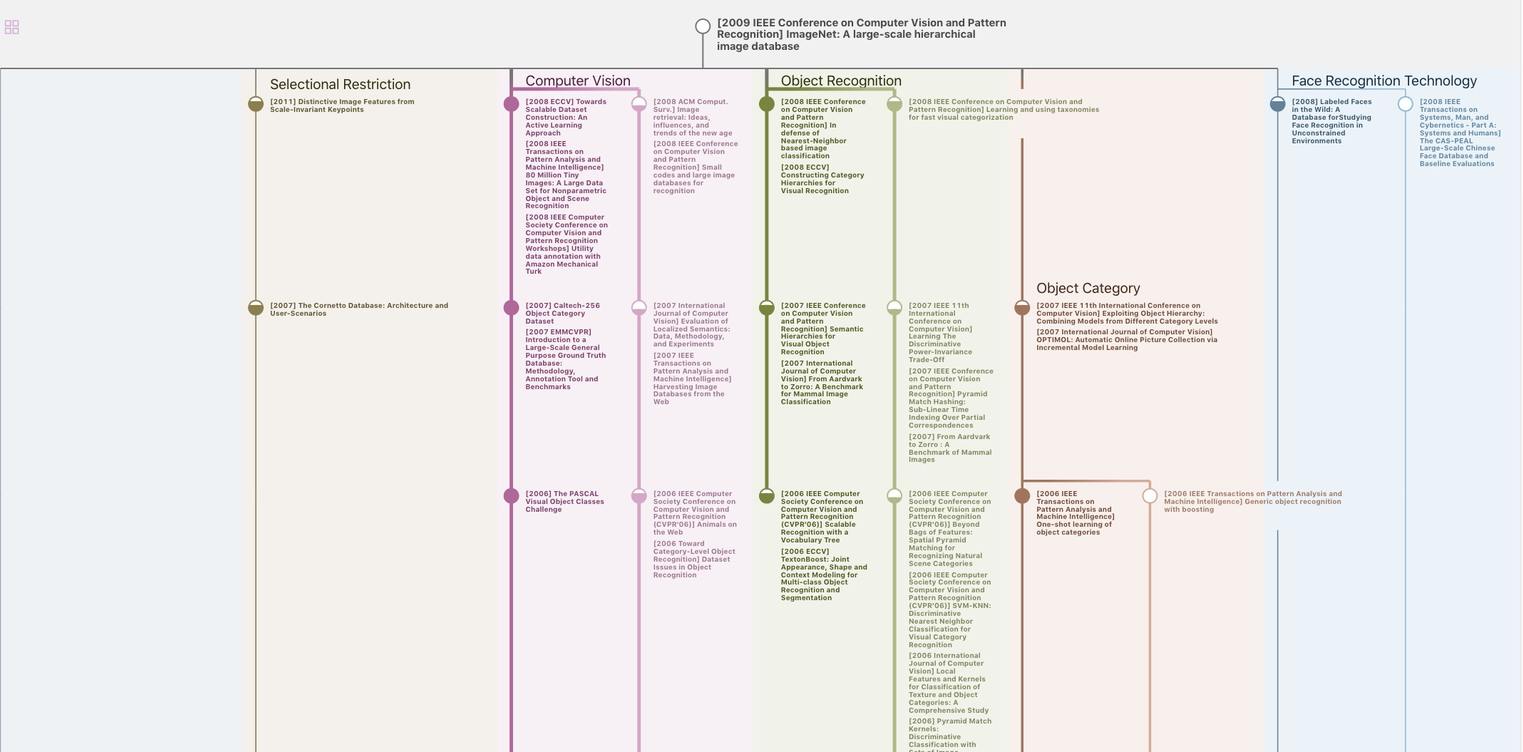
生成溯源树,研究论文发展脉络
Chat Paper
正在生成论文摘要