Generative Question Refinement with Deep Reinforcement Learning in Retrieval-based QA System
PROCEEDINGS OF THE 28TH ACM INTERNATIONAL CONFERENCE ON INFORMATION & KNOWLEDGE MANAGEMENT (CIKM '19)(2019)
摘要
In real-world question-answering (QA) systems, ill-formed questions, such as wrong words, ill word order and noisy expressions, are common and may prevent the QA systems from understanding and answering them accurately. In order to eliminate the effect of ill-formed questions, we approach the question refinement task and propose a unified model, QREFINE, to refine the ill-formed questions to well-formed question. The basic idea is to learn a Seq2Seq model to generate a new question from the original one. To improve the quality and retrieval performance of the generated questions, we make two major improvements: 1) To better encode the semantics of ill-formed questions, we enrich the representation of questions with character embedding and the recent proposed contextual word embedding such as BERT, besides the traditional context-free word embeddings; 2) To make it capable to generate desired questions, we train the model with deep reinforcement learning techniques that considers an appropriate wording of the generation as an immediate reward and the correlation between generated question and answer as time-delayed long-term rewards. Experimental results on real-world datasets show that the proposed QREFINE method can generate refined questions with more readability but fewer mistakes than the original questions provided by users. Moreover, the refined questions also significantly improve the accuracy of answer retrieval.
更多查看译文
关键词
multigrain word embedding, off-policy reinforcement learning, question refinement
AI 理解论文
溯源树
样例
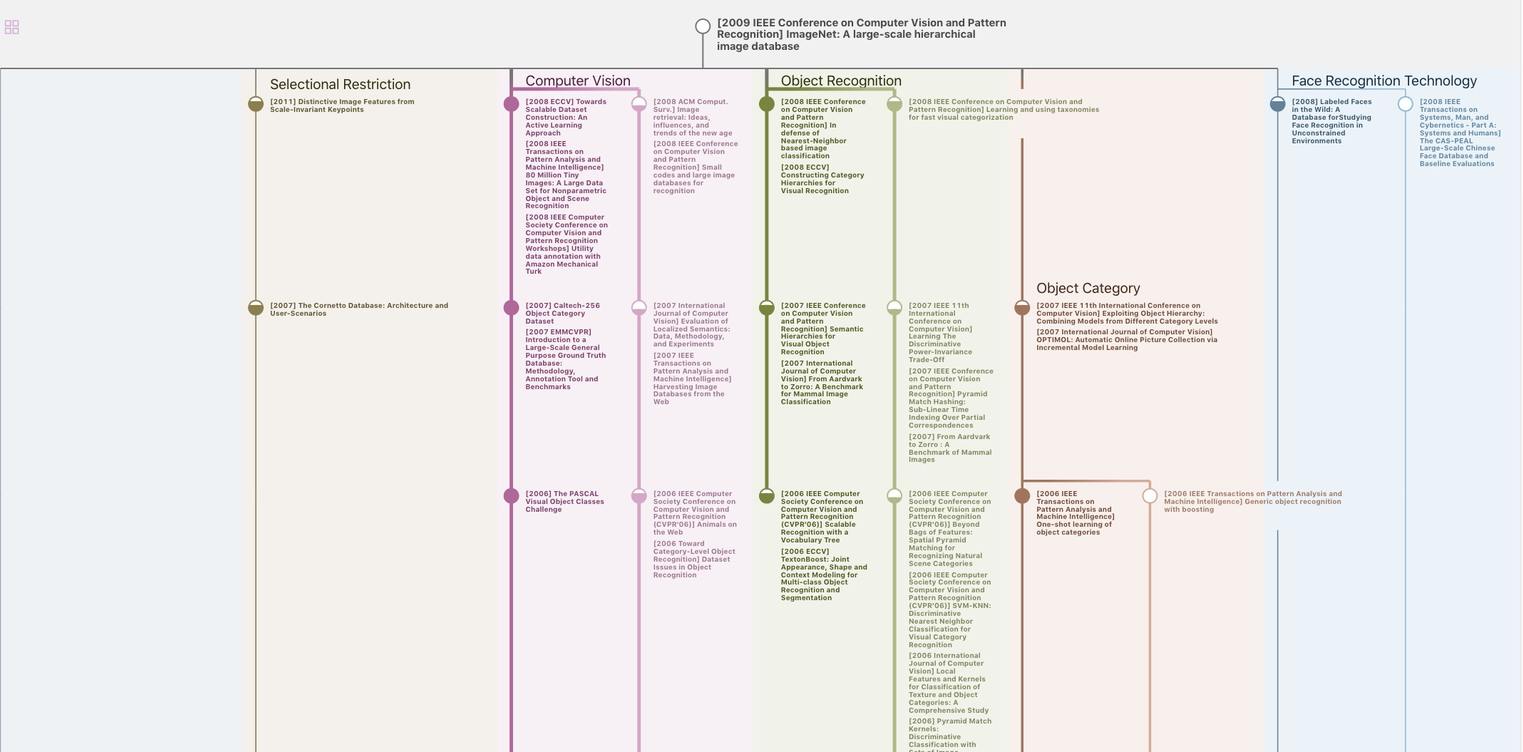
生成溯源树,研究论文发展脉络
Chat Paper
正在生成论文摘要