DGSAN: Discrete generative self-adversarial network
Neurocomputing(2021)
摘要
Although GAN-based methods have received many achievements in the last few years, they have not been entirely successful in generating discrete data. The most crucial challenge of these methods is the difficulty of passing the gradient from the discriminator to the generator when the generator outputs are discrete. Despite the fact that several attempts have been made to alleviate this problem, none of the existing GAN-based methods have improved the performance of text generation compared with the maximum likelihood approach in terms of both the quality and the diversity. In this paper, we proposed a new framework for generating discrete data by an adversarial approach in which there is no need to pass the gradient to the generator. The proposed method has an iterative manner in which each new generator is defined based on the last discriminator. It leverages the discreteness of data and the last discriminator to model the real data distribution implicitly. Moreover, the method is supported with theoretical guarantees, and experimental results generally show the superiority of the proposed DGSAN method compared to the other popular or recent methods in generating discrete sequential data.
更多查看译文
关键词
Discrete data,Generative model,Self adversarial,Sequential
AI 理解论文
溯源树
样例
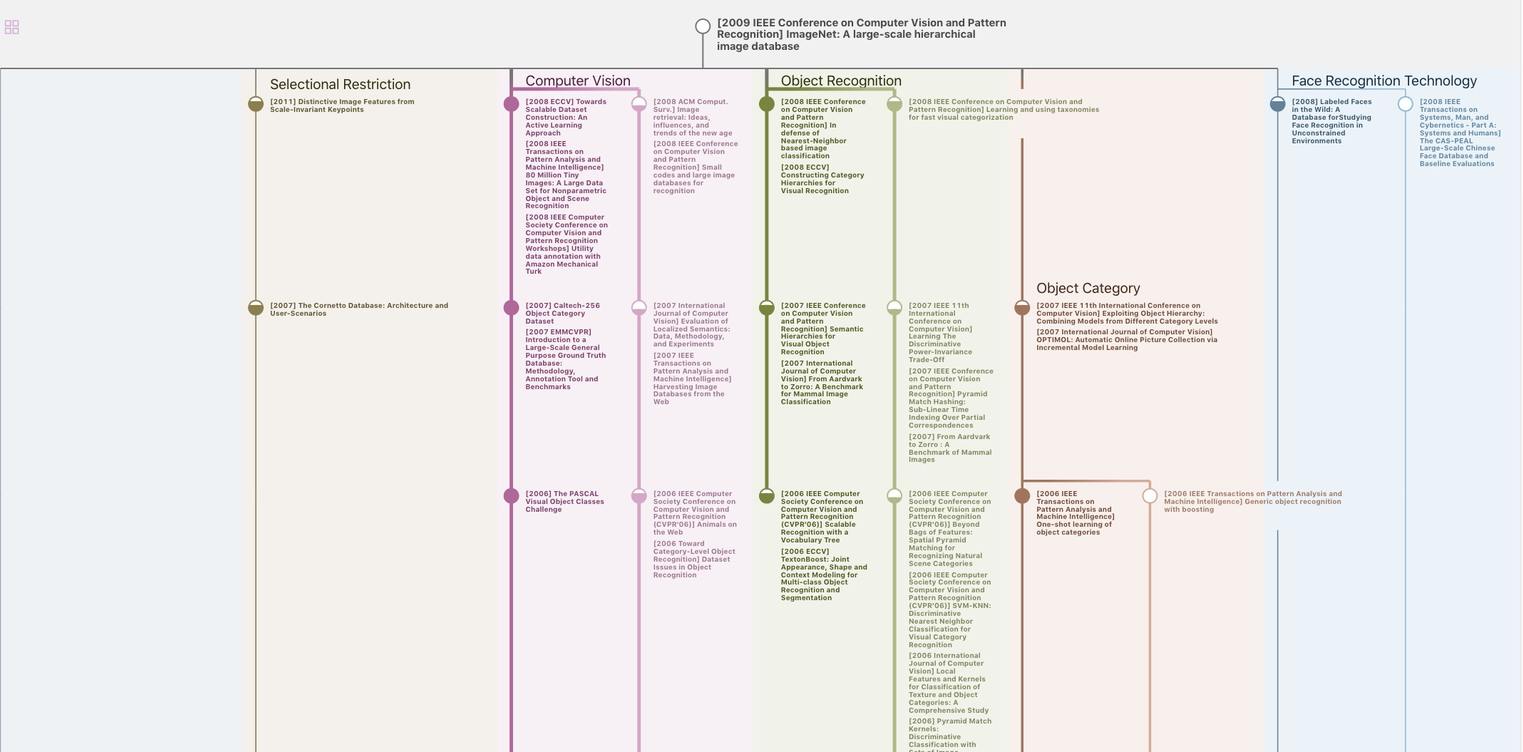
生成溯源树,研究论文发展脉络
Chat Paper
正在生成论文摘要