Accurate total solar irradiance estimates under irradiance measurements scarcity scenarios
Environmental Monitoring and Assessment(2019)
摘要
Accurate estimates of total global solar irradiance reaching the Earth’s surface are relevant since routine measurements are not always available. This work aimed to determine which of the models used to estimate daily total global solar irradiance (TGSI) is the best model when irradiance measurements are scarce in a given site. A model based on an artificial neural network (ANN) and empirical models based on temperature and sunshine measurements were analyzed and evaluated in Córdoba, Argentina. The performance of the models was benchmarked using different statistical estimators such as the mean bias error (MBE), the mean absolute bias error (MABE), the correlation coefficient ( r ), the Nash-Sutcliffe equation (NSE), and the statistics t test ( t value). The results showed that when enough measurements were available, both the ANN and the empirical models accurately predicted TGSI (with MBE and MABE ≤ |0.11| and ≤ |1.98| kWh m −2 day −1 , respectively; NSE ≥ 0.83; r ≥ 0.95; and | t values| < t critical value). However, when few TGSI measurements were available (2, 3, 5, 7, or 10 days per month) only the ANN-based method was accurate (| t value| < t critical value), yielding precise results although only 2 measurements per month were available for 1 year. This model has an important advantage over the empirical models and is very relevant to Argentina due to the scarcity of TGSI measurements.
更多查看译文
关键词
Artificial neural network,Scarce measurements,Solar energy,Solar radiation estimation
AI 理解论文
溯源树
样例
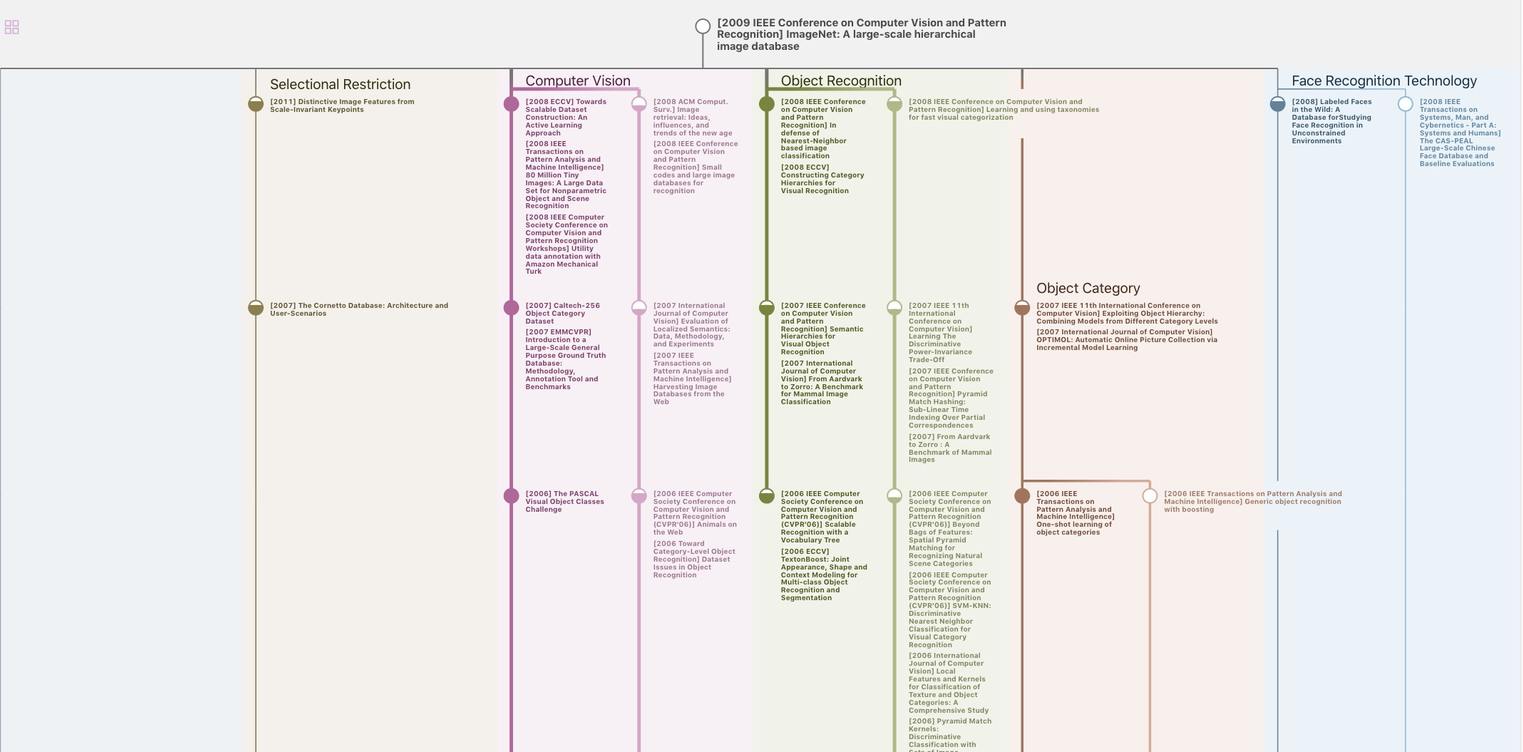
生成溯源树,研究论文发展脉络
Chat Paper
正在生成论文摘要