Learned Map Prediction for Enhanced Mobile Robot Exploration
2019 INTERNATIONAL CONFERENCE ON ROBOTICS AND AUTOMATION (ICRA)(2019)
摘要
We demonstrate an autonomous ground robot capable of exploring unknown indoor environments for reconstructing their 2D maps. This problem has been traditionally tackled by geometric heuristics and information theory. More recently, deep learning and reinforcement learning based approaches have been proposed to learn exploration behavior in an end-to-end manner. We present a method that combines the strengths of these different approaches. Specifically, we employ a state-of-the-art generative neural network to predict unknown regions of a partially explored map, and use the prediction to enhance the exploration in an information-theoretic manner. We evaluate our system in simulation using floor plans of real buildings. We also present comparisons with traditional methods which demonstrate the advantage of our method in terms of exploration efficiency. We retain an advantage over end-to-end learned exploration methods in that the robot's behavior is easily explicable in terms of the predicted map.
更多查看译文
关键词
autonomous ground robot,geometric heuristics,information theory,deep learning,mobile robot exploration,generative neural network,2D maps,reinforcement learning,robots behavior
AI 理解论文
溯源树
样例
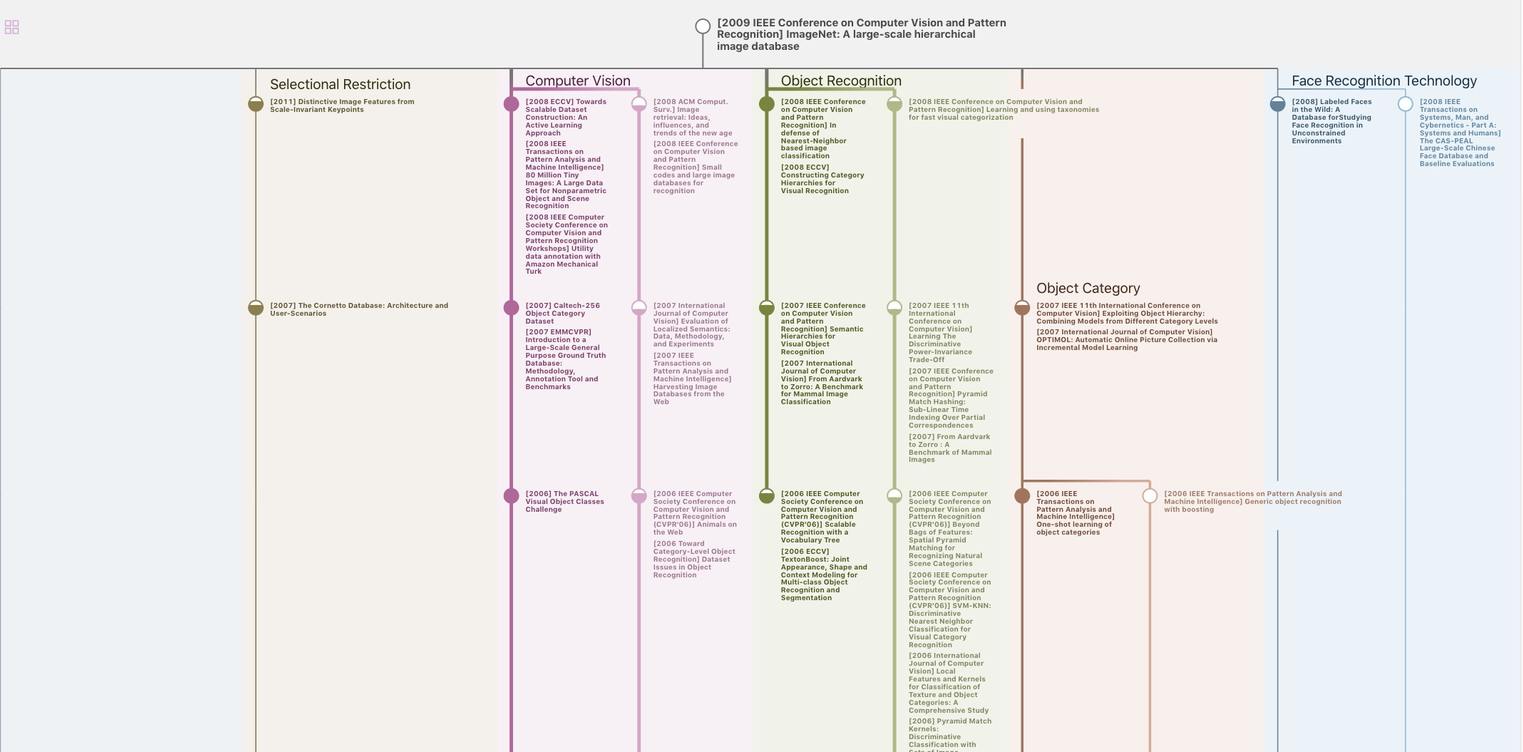
生成溯源树,研究论文发展脉络
Chat Paper
正在生成论文摘要