Efficient Implementation Of Convolutional Neural Networks With End To End Integer-Only Dataflow
2019 IEEE INTERNATIONAL CONFERENCE ON MULTIMEDIA AND EXPO (ICME)(2019)
摘要
Linear INT8 quantization is presented to construct an end to end integer-only dataflow for efficient inference of modern CNNs. The INT8 method is implemented with unified layer representation, thus quantized CNNs can be partitioned into computation subgraphs consisting of stacked unified layers with simplified integer-only arithmetic flow and scaling back mechanism, indicating high effectiveness for specific hardware realization. Experimental results show that both the classification and object detection models quantized by proposed INT8 method suffer approximate 1% accuracy loss, exhibiting comparable results with TensorRT. As a result, the deep learning accelerator (DLA) with integer-only dataflow and efficient memory hierarchy is designed for CNN applications.
更多查看译文
关键词
model compression, INT8 quantization, unified layer representation, FPGA implementation
AI 理解论文
溯源树
样例
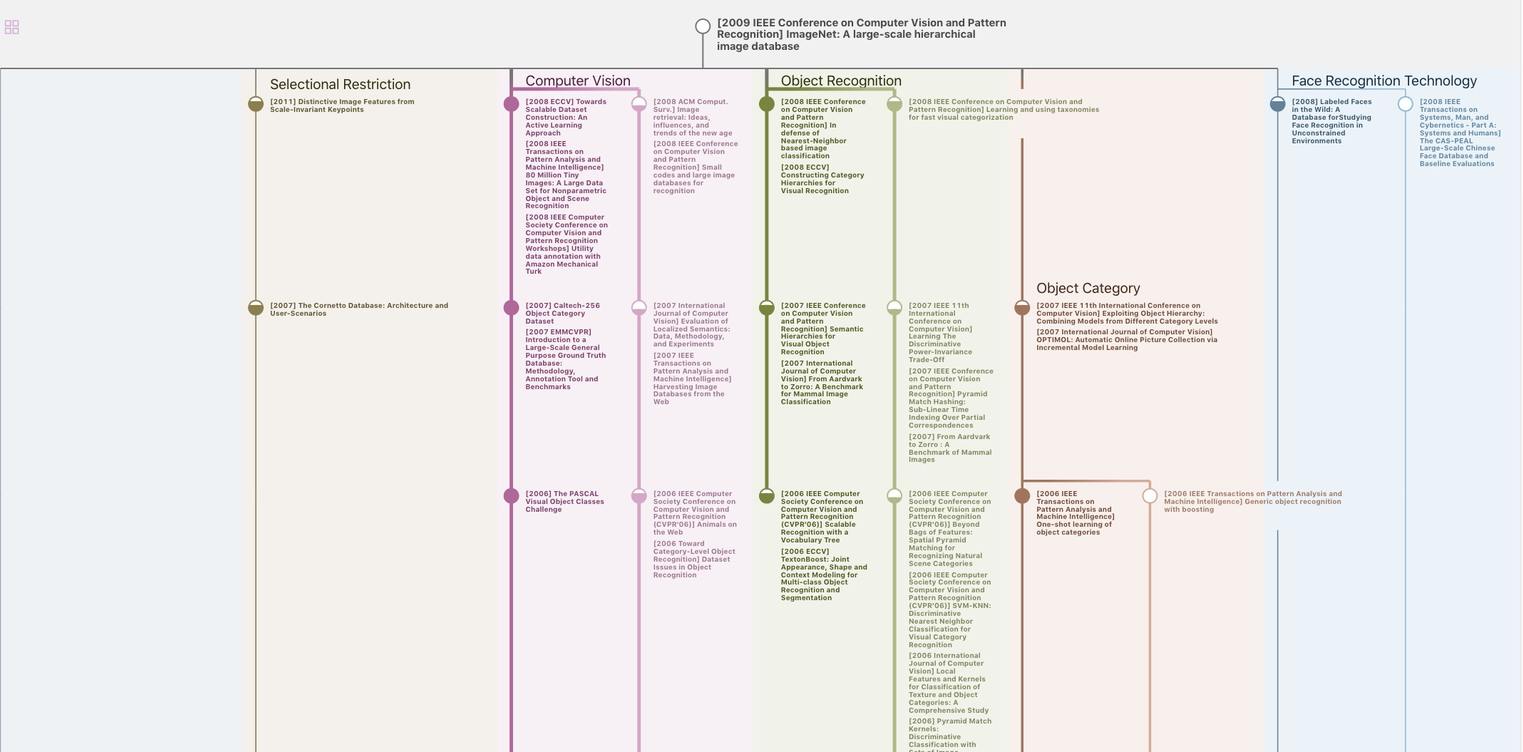
生成溯源树,研究论文发展脉络
Chat Paper
正在生成论文摘要