A Survey on Data Collection for Machine Learning: A Big Data - AI Integration Perspective
IEEE Transactions on Knowledge and Data Engineering(2021)
摘要
Data collection is a major bottleneck in machine learning and an active research topic in multiple communities. There are largely two reasons data collection has recently become a critical issue. First, as machine learning is becoming more widely-used, we are seeing new applications that do not necessarily have enough labeled data. Second, unlike traditional machine learning, deep learning techniques automatically generate features, which saves feature engineering costs, but in return may require larger amounts of labeled data. Interestingly, recent research in data collection comes not only from the machine learning, natural language, and computer vision communities, but also from the data management community due to the importance of handling large amounts of data. In this survey, we perform a comprehensive study of data collection from a data management point of view. Data collection largely consists of data acquisition, data labeling, and improvement of existing data or models. We provide a research landscape of these operations, provide guidelines on which technique to use when, and identify interesting research challenges. The integration of machine learning and data management for data collection is part of a larger trend of Big data and Artificial Intelligence (AI) integration and opens many opportunities for new research.
更多查看译文
关键词
Data collection,data acquisition,data labeling,machine learning
AI 理解论文
溯源树
样例
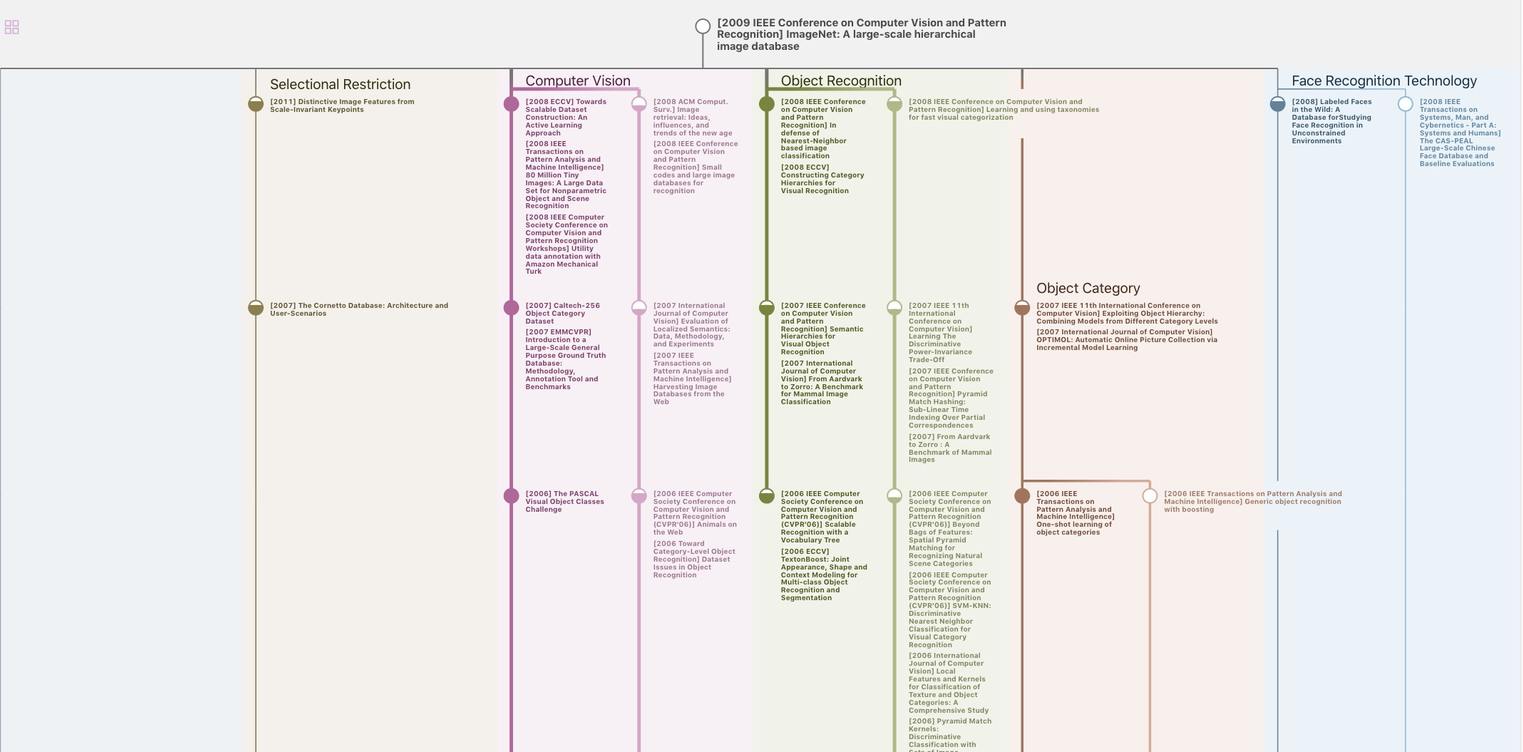
生成溯源树,研究论文发展脉络
Chat Paper
正在生成论文摘要