RecoNet - An Interpretable Neural Architecture for Recommender Systems.
IJCAI(2019)
摘要
Neural systems offer high predictive accuracy but are plagued by long training times and low interpretability. We present a simple neural architecture for recommender systems that lifts several of these shortcomings Firstly, the approach has a high predictive power that is comparable to state-of-the-art recommender approaches. Secondly, owing to its simplicity, the trained model can be interpreted easily because it provides the individual contribution of each input feature to the decision. Our method is three orders of magnitude faster than general-purpose explanatory approaches, such as LIME. Finally, thanks to its design, our architecture addresses cold-start issues, and therefore the model does not require retraining in the presence of new users.
更多查看译文
AI 理解论文
溯源树
样例
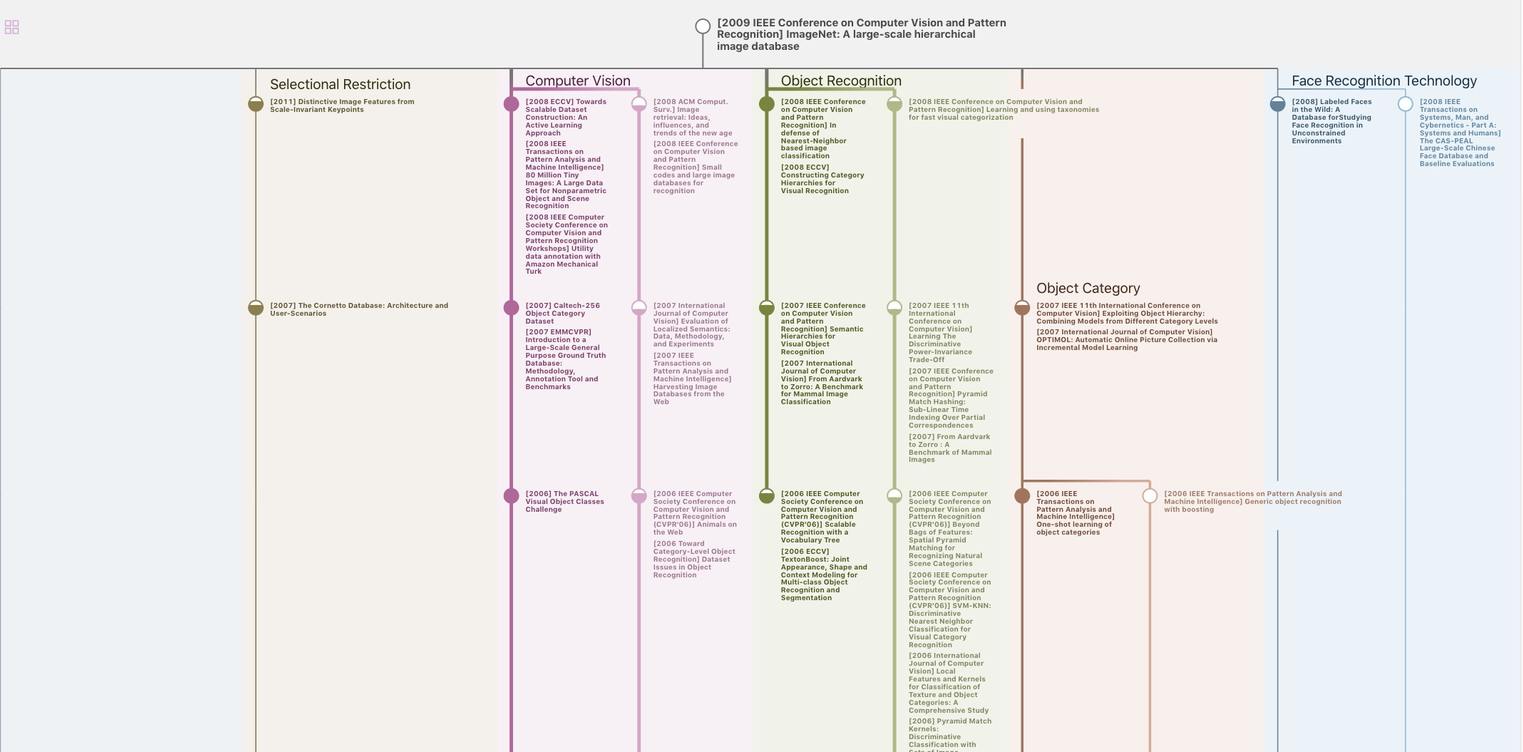
生成溯源树,研究论文发展脉络
Chat Paper
正在生成论文摘要