Paired-Consistency - An Example-Based Model-Agnostic Approach to Fairness Regularization in Machine Learning.
CoRR(2019)
摘要
As AI systems develop in complexity it is becoming increasingly hard to ensure non-discrimination on the basis of protected attributes such as gender, age, and race. Many recent methods have been developed for dealing with this issue as long as the protected attribute is explicitly available for the algorithm. We address the setting where this is not the case (with either no explicit protected attribute, or a large set of them). Instead, we assume the existence of a fair domain expert capable of generating an extension to the labeled dataset - a small set of example pairs, each having a different value on a subset of protected variables, but judged to warrant a similar model response. We define a performance metric - paired consistency. Paired consistency measures how close the output (assigned by a classifier or a regressor) is on these carefully selected pairs of examples for which fairness dictates identical decisions. In some cases consistency can be embedded within the loss function during optimization and serve as a fairness regularizer, and in others it is a tool for fair model selection. We demonstrate our method using the well studied Income Census dataset.
更多查看译文
关键词
Fairness, AI, Machine learning, Social responsibility
AI 理解论文
溯源树
样例
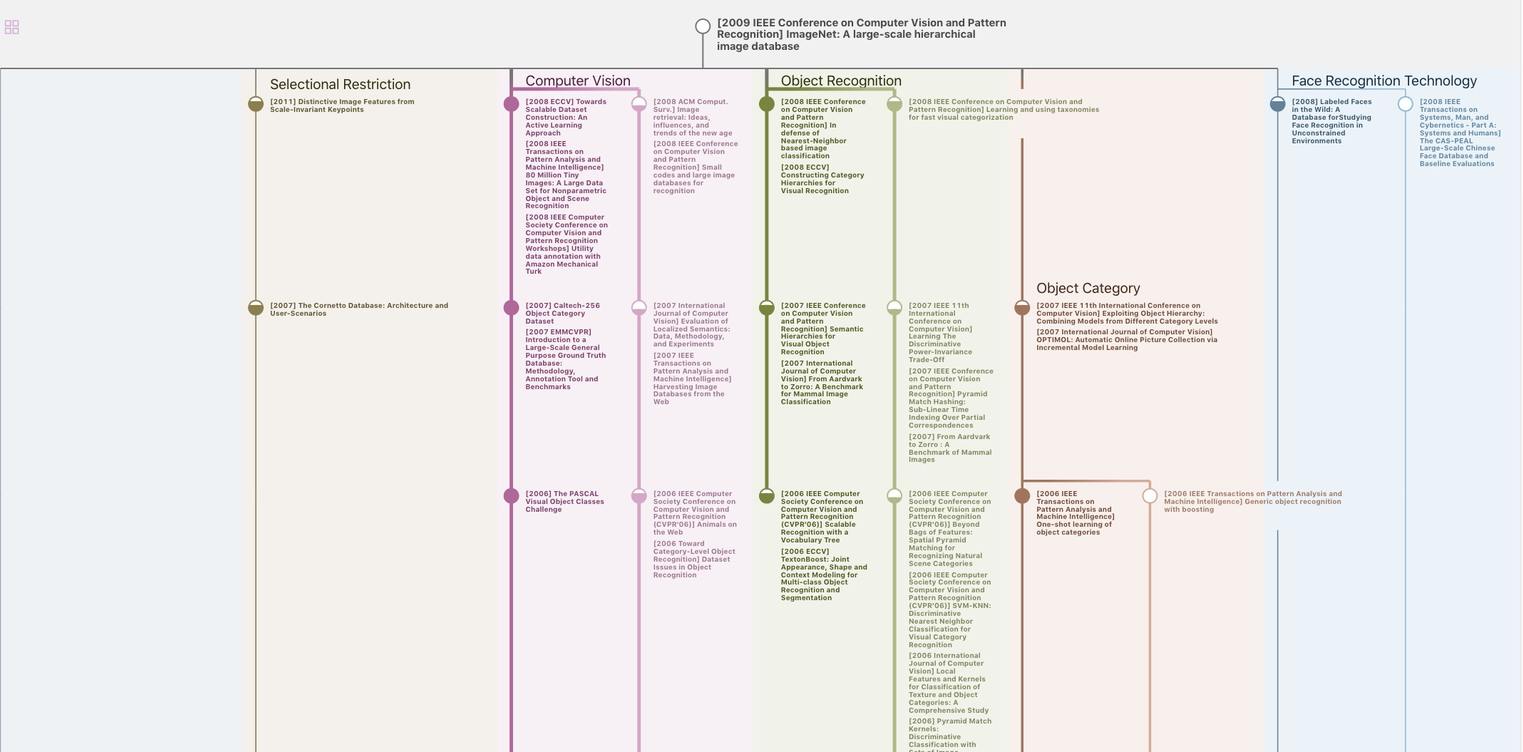
生成溯源树,研究论文发展脉络
Chat Paper
正在生成论文摘要