Model Agnostic Defence Against Backdoor Attacks in Machine Learning
IEEE Transactions on Reliability(2022)
摘要
Machine learning (ML) has automated a multitude of our day-to-day decision-making domains, such as education, employment, and driving automation. The continued success of ML largely depends on our ability to trust the model we are using. Recently, a new class of attacks called backdoor attacks have been developed. These attacks undermine the user’s trust in ML models. In this article, we present
Neo
, a model agnostic framework to detect and mitigate such backdoor attacks in image classification ML models. For a given image classification model, our approach analyzes the inputs it receives and determines if the model is backdoored. In addition to this feature, we also mitigate these attacks by determining the correct predictions of the poisoned images. We have implemented
Neo
and evaluated it against three state-of-the-art poisoned models. In our evaluation, we show that
Neo
can detect
$\approx$
88% of the poisoned inputs on average and it is as fast as 4.4 ms per input image. We also compare our
Neo
approach with the state-of-the-art defence methodologies proposed for backdoor attacks. Our evaluation reveals that despite being a blackbox approach,
Neo
is more effective in thwarting backdoor attacks than the existing techniques. Finally, we also reconstruct the exact poisoned input for the user to effectively test their systems.
更多查看译文
关键词
Computer security,machine learning,data integrity
AI 理解论文
溯源树
样例
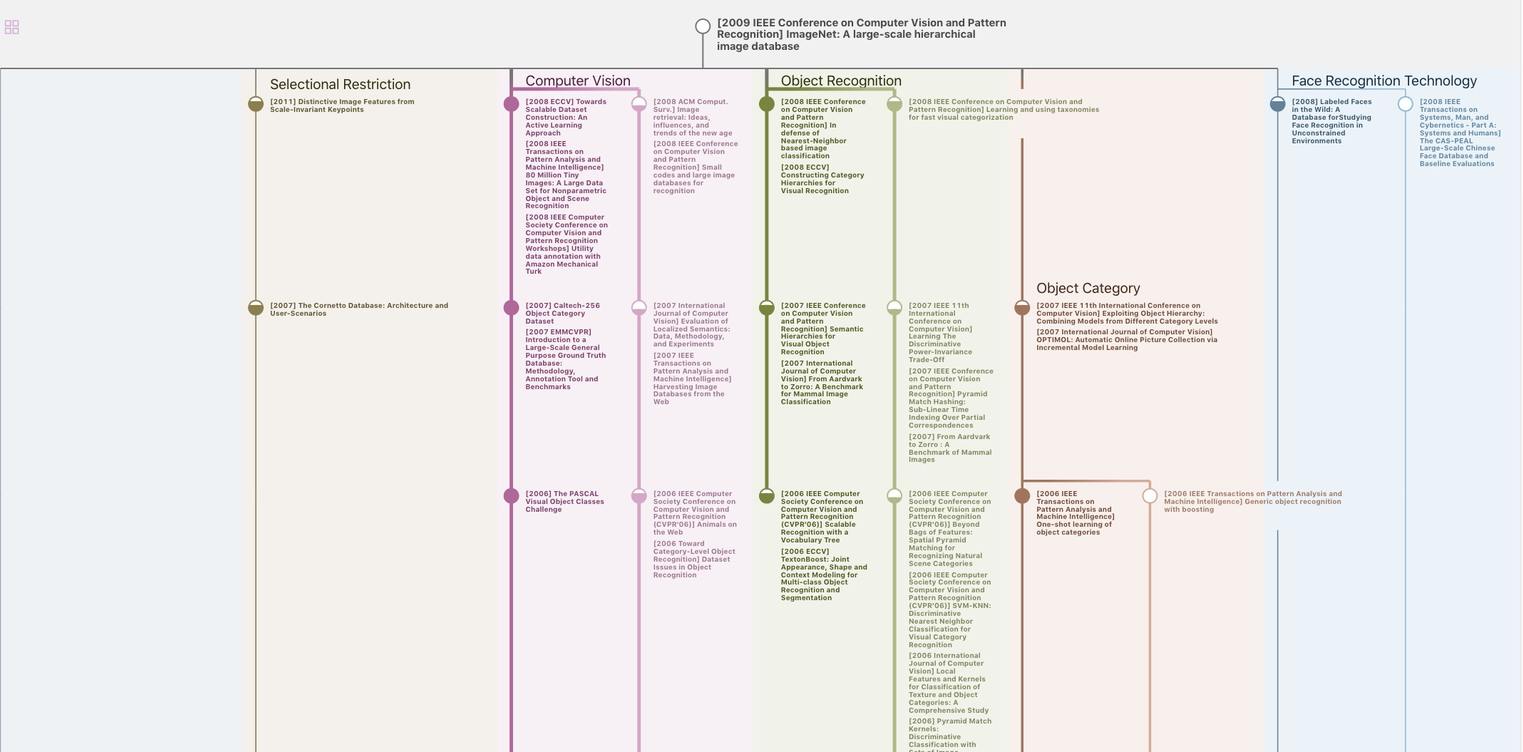
生成溯源树,研究论文发展脉络
Chat Paper
正在生成论文摘要