Extending The Step-Size Restriction For Gradient Descent To Avoid Strict Saddle Points
SIAM JOURNAL ON MATHEMATICS OF DATA SCIENCE(2020)
摘要
We provide larger step-size restrictions for which gradient descent-based algorithms (almost surely) avoid strict saddle points. In particular, consider a twice differentiable (nonconvex) objective function whose gradient has Lipschitz constant L and that the set of points that obtain the maximum value of the spectral norm of the Hessian is measure zero. We prove that given one uniformly random initialization, the probability that gradient descent with a step-size up to 2/L will converge to a strict saddle point is zero. This extends previous results up to the sharp limit imposed by the convex quadratic case (provably converging to local minimizers). In addition, the arguments hold in the case when a learning rate schedule is given, with either a continuous decaying rate or a piecewise constant schedule. We show that the assumptions are robust in the sense that functions which do not satisfy the assumptions are meager with respect to analytic functions.
更多查看译文
关键词
gradient descent, nonconvex, strict saddles, optimization
AI 理解论文
溯源树
样例
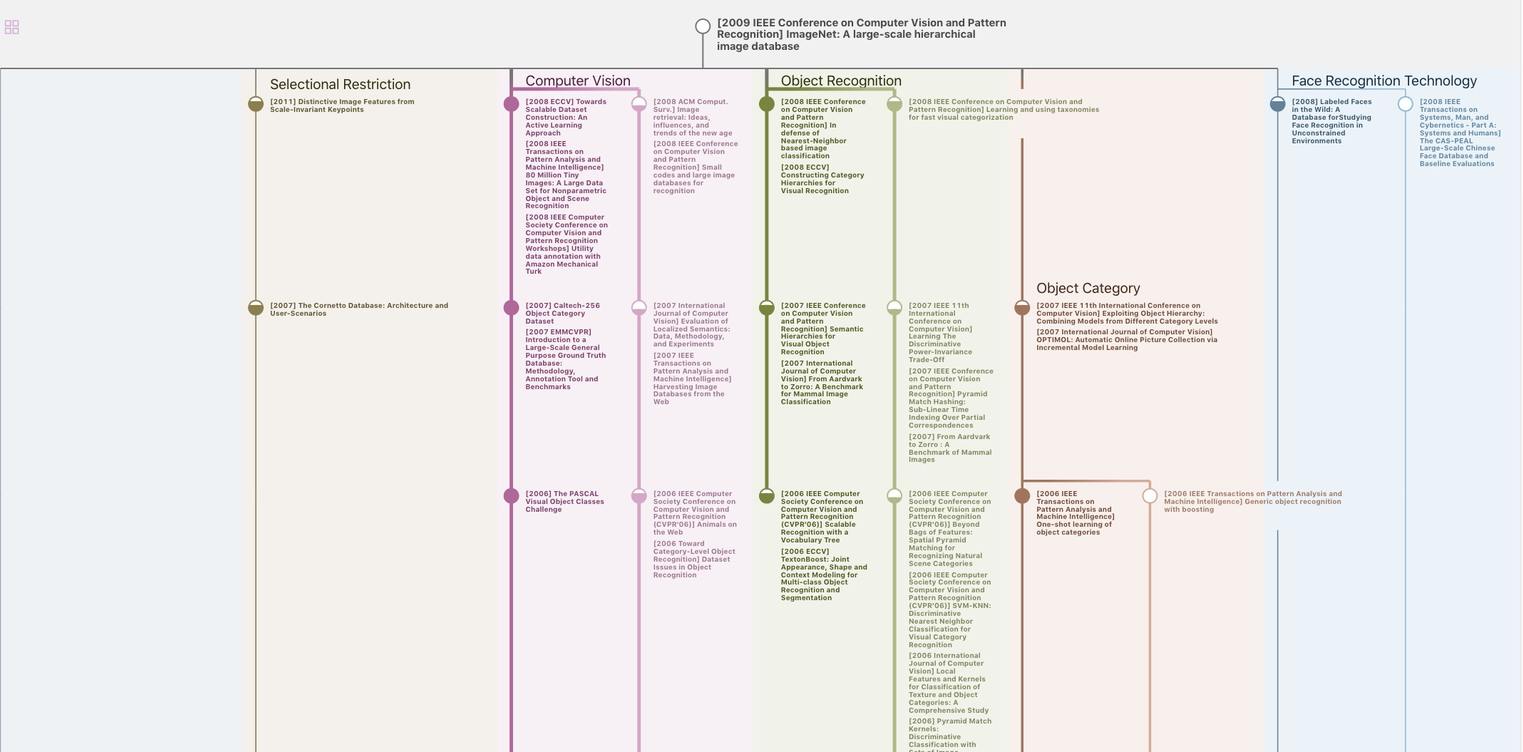
生成溯源树,研究论文发展脉络
Chat Paper
正在生成论文摘要