SCRDet: Towards More Robust Detection for Small, Cluttered and Rotated Objects
2019 IEEE/CVF INTERNATIONAL CONFERENCE ON COMPUTER VISION (ICCV 2019)(2019)
摘要
Object detection has been a building block in computer vision. Though considerable progress has been made, there still exist challenges for objects with small size, arbitrary direction, and dense distribution. Apart from natural images, such issues are especially pronounced for aerial images of great importance. This paper presents a novel multi-category rotation detector for small, cluttered and rotated objects, namely SCRDet. Specifically, a sampling fusion network is devised which fuses multi-layer feature with effective anchor sampling, to improve the sensitivity to small objects. Meanwhile, the supervised pixel attention network and the channel attention network are jointly explored for small and cluttered object detection by suppressing the noise and highlighting the objects feature. For more accurate rotation estimation, the IoU constant factor is added to the smooth L1 loss to address the boundary problem for the rotating bounding box. Extensive experiments on two remote sensing public datasets DOTA, NWPU VHR-10 as well as natural image datasets COCO, VOC2007 and scene text data ICDAR2015 show the state-of-the-art performance of our detector. The code and models will be available at https://github.com/DetectionTeamUCAS.
更多查看译文
关键词
SCRDet,robust detection,natural images,aerial images,novel multicategory rotation detector,small objects,rotated objects,sampling fusion network,supervised pixel attention network,channel attention network,cluttered object detection,natural image datasets,COCO,computer vision
AI 理解论文
溯源树
样例
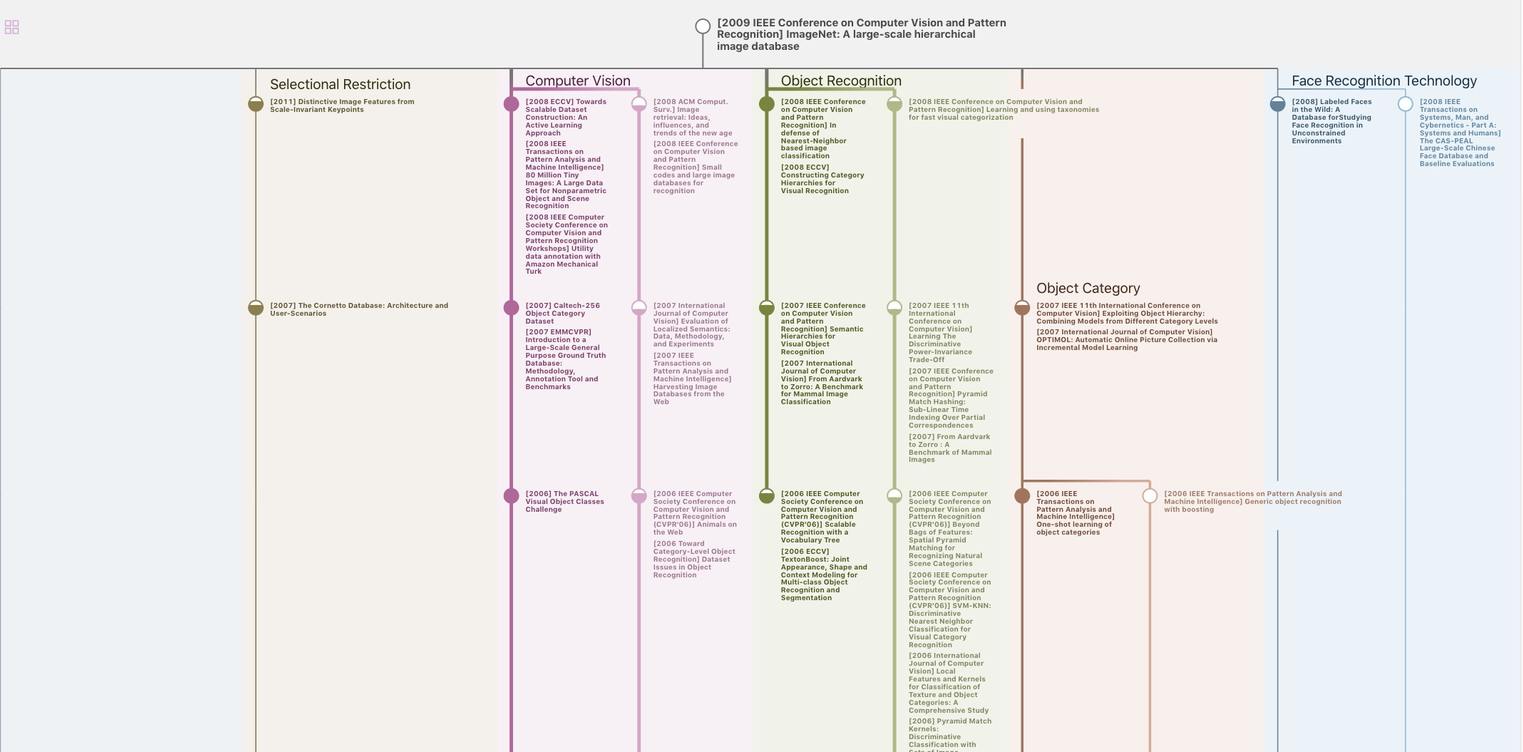
生成溯源树,研究论文发展脉络
Chat Paper
正在生成论文摘要