Forecasting under model uncertainty:Non-homogeneous hidden Markov models with Polya-Gamma data augmentation
JOURNAL OF FORECASTING(2020)
摘要
We consider finite state-space non-homogeneous hidden Markov models for forecasting univariate time series. Given a set of predictors, the time series are modeled via predictive regressions with state-dependent coefficients and time-varying transition probabilities that depend on the predictors via a logistic/multinomial function. In a hidden Markov setting, inference for logistic regression coefficients becomes complicated and in some cases impossible due to convergence issues. In this paper, we aim to address this problem utilizing the recently proposed Polya-Gamma latent variable scheme. Also, we allow for model uncertainty regarding the predictors that affect the series both linearly - in the mean - and non-linearly - in the transition matrix. Predictor selection and inference on the model parameters are based on an automatic Markov chain Monte Carlo scheme with reversible jump steps. Hence the proposed methodology can be used as a black box for predicting time series. Using simulation experiments, we illustrate the performance of our algorithm in various setups, in terms of mixing properties, model selection and predictive ability. An empirical study on realized volatility data shows that our methodology gives improved forecasts compared to benchmark models.
更多查看译文
关键词
non-homogeneous hidden Markov models,model selection,forecasting,Polya-Gamma data augmentation,realized volatility
AI 理解论文
溯源树
样例
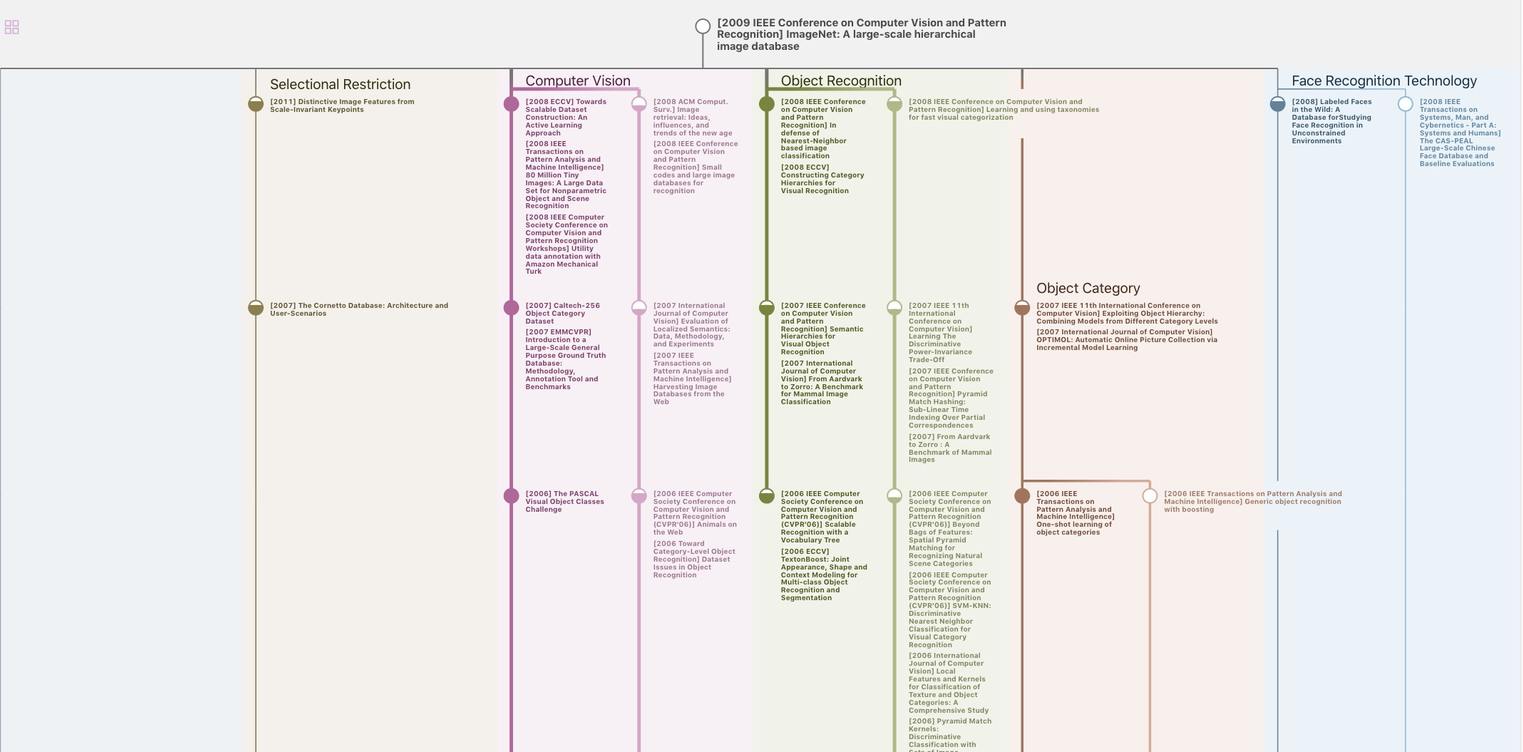
生成溯源树,研究论文发展脉络
Chat Paper
正在生成论文摘要