Reducing the Dependence of the Neural Network Function to Systematic Uncertainties in the Input Space
Computing and Software for Big Science(2020)
摘要
Applications of neural networks to data analyses in natural sciences are complicated by the fact that many inputs are subject to systematic uncertainties. To control the dependence of the neural network function to variations of the input space within these systematic uncertainties, several methods have been proposed. In this work, we propose a new approach of training the neural network by introducing penalties on the variation of the neural network output directly in the loss function. This is achieved at the cost of only a small number of additional hyperparameters. It can also be pursued by treating all systematic variations in the form of statistical weights. The proposed method is demonstrated with a simple example, based on pseudo-experiments, and by a more complex example from high-energy particle physics.
更多查看译文
关键词
Neural networks,Systematic uncertainties,High-energy particle physics
AI 理解论文
溯源树
样例
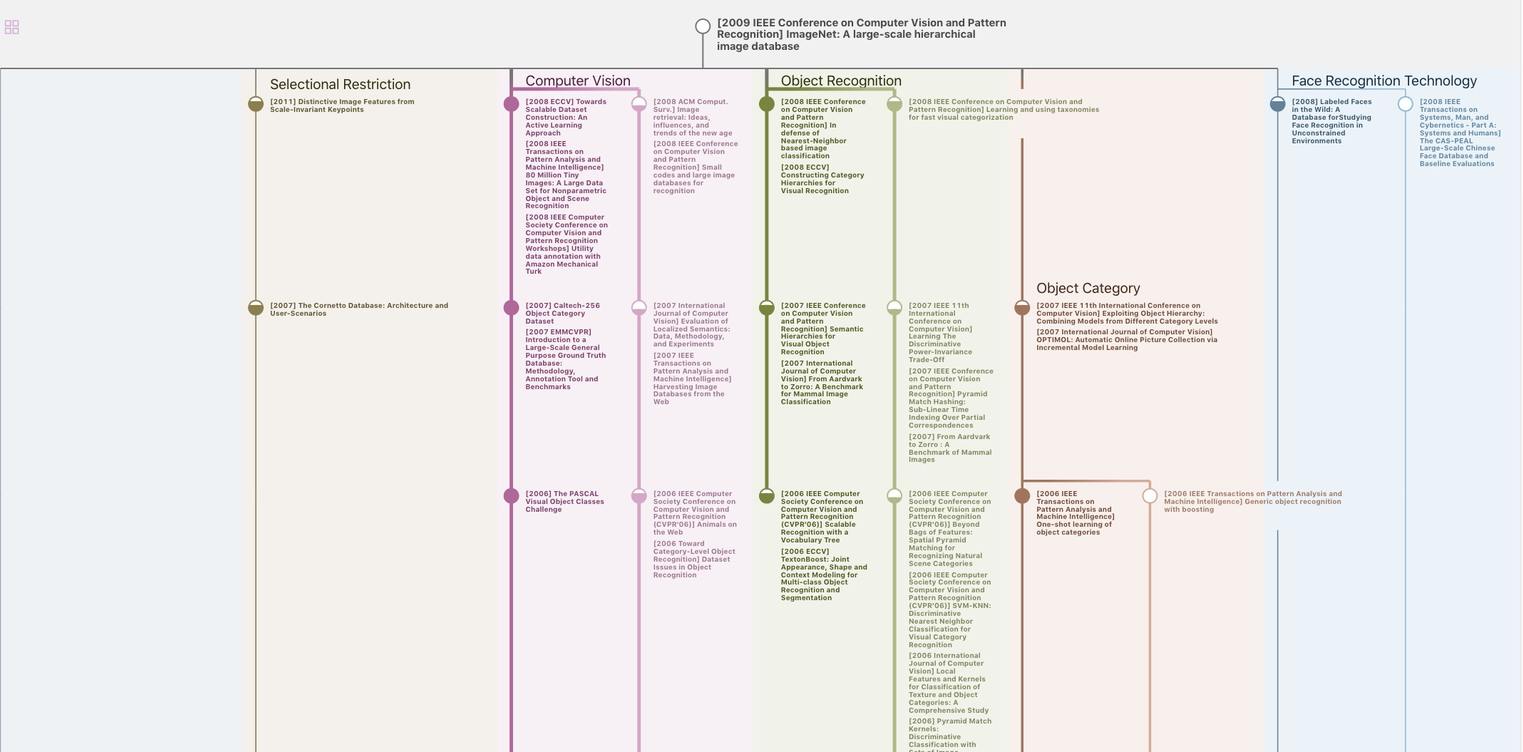
生成溯源树,研究论文发展脉络
Chat Paper
正在生成论文摘要