Efficient and Effective Express via Contextual Cooperative Reinforcement Learning
KDD '19: The 25th ACM SIGKDD Conference on Knowledge Discovery and Data Mining Anchorage AK USA August, 2019(2019)
摘要
Express systems are widely deployed in many major cities. Couriers in an express system load parcels at transit station and deliver them to customers. Meanwhile, they also try to serve the pick-up requests which come stochastically in real time during the delivery process. Having brought much convenience and promoted the development of e-commerce, express systems face challenges on courier management to complete the massive number of tasks per day. Considering this problem, we propose a reinforcement learning based framework to learn a courier management policy. Firstly, we divide the city into independent regions, in each of which a constant number of couriers deliver parcels and serve requests cooperatively. Secondly, we propose a soft-label clustering algorithm named Balanced Delivery-Service Burden (BDSB) to dispatch parcels to couriers in each region. BDSB guarantees that each courier has almost even delivery and expected request-service burden when departing from transit station, giving a reasonable initialization for online management later. As pick-up requests come in real time, a Contextual Cooperative Reinforcement Learning (CCRL) model is proposed to guide where should each courier deliver and serve in each short period. Being formulated in a multi-agent way, CCRL focuses on the cooperation among couriers while also considering the system context. Experiments on real-world data from Beijing are conducted to confirm the outperformance of our model.
更多查看译文
关键词
constrained clustering, express system, reinforcement learning
AI 理解论文
溯源树
样例
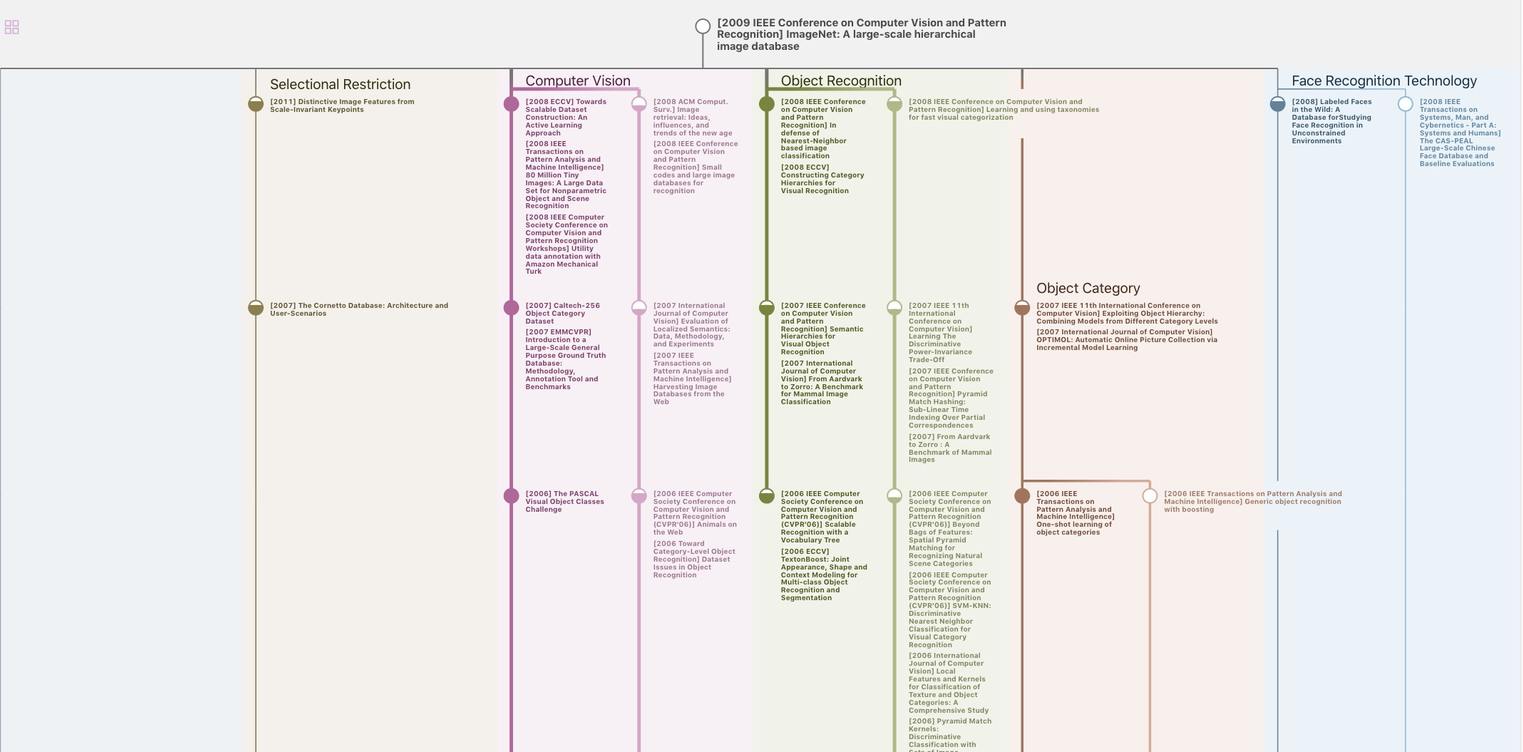
生成溯源树,研究论文发展脉络
Chat Paper
正在生成论文摘要