Composing Diverse Policies for Temporally Extended Tasks
IEEE ROBOTICS AND AUTOMATION LETTERS(2020)
摘要
Temporally extended and sequenced robot motion tasks are often characterized by discontinuous switches between different types of local dynamics. These change-points can be exploited to build approximate models of the interleaving regions, which in turn allow the design of region-specific controllers. These can then be combined to create the initiation state-space of a final policy. However, such a pipeline can become challenging to implement for combinatorially complex, temporarily extended tasks - especially so when sub-controllers work on different information streams, time scales and action spaces. In this paper, we introduce a method that can compose diverse policies based on scripted motion planning, dynamic motion primitives and neural networks. In order to do this, we extend the options framework to introduce a per-option dynamics module and a global function that evaluates a goal metric. Additionally, we can leverage expert demonstrations to sequence these local policies, converting the learning problem in hierarchical reinforcement learning to a planning problem at inference time. We first illustrate the core concepts with an MDP benchmark, and then with a physical gear assembly task solved on a PR2 robot. We show that the proposed approach successfully discovers the optimal sequence of policies and solves both tasks efficiently.
更多查看译文
关键词
Motion and path planning,learning and adaptive systems,learning from demonstration
AI 理解论文
溯源树
样例
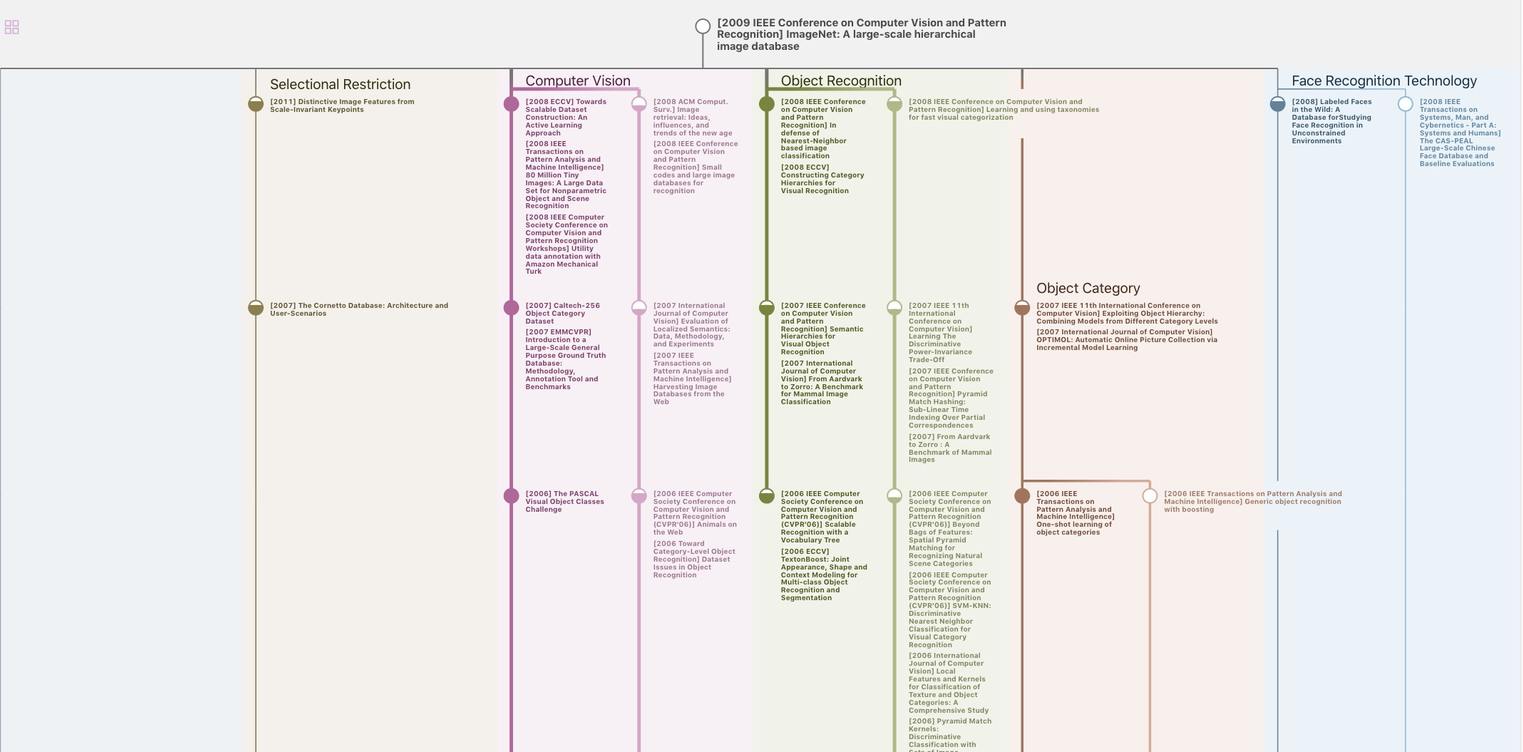
生成溯源树,研究论文发展脉络
Chat Paper
正在生成论文摘要